Title: A 2.1TFLOPS/W Mobile Deep RL Accelerator with Transposable PE Array and Experience Compression
Authors: Chang-Hyeon Kim, Sang-Hoon Kang, Don-Joo Shin, Sung-Pill Choi, Young-Woo Kim and Hoi-Jun Yoo
Recently, deep neural networks (DNNs) are actively used for action control so that an autonomous system, such as the robot, can perform human-like behaviors and operations. Unlike recognition tasks, the real-time operation is essential in action control, and it is too slow to use remote learning on a server communicating through a network. New learning techniques, such as reinforcement learning (RL), are needed to determine and select the correct robot behavior locally. In this paper, We propose DRL accelerator with transposable PE array and experience compressor to realize real-time DRL operation of autonomous agents in dynamic environments. It supports on-chip data compression and decompression that ~10,000 of DRL experiences can be compressed by 65%. And it enables adaptive data reuse for inferencing and training, which results in power and peak memory bandwidth reduction by 31% and 41%, respectively. The proposed DRL accelerator is fabricated with 65nm CMOS technology and occupies 4×4 mm2 die area. This is the first fully trainable DRL processor, and it achieves 2.16 TFLOPS/W energy-efficiency at 0.73V with 16b weights@50MHz.
Fig. 1. DRL Accelerator with tPE Array, Implementation & Measurement Results
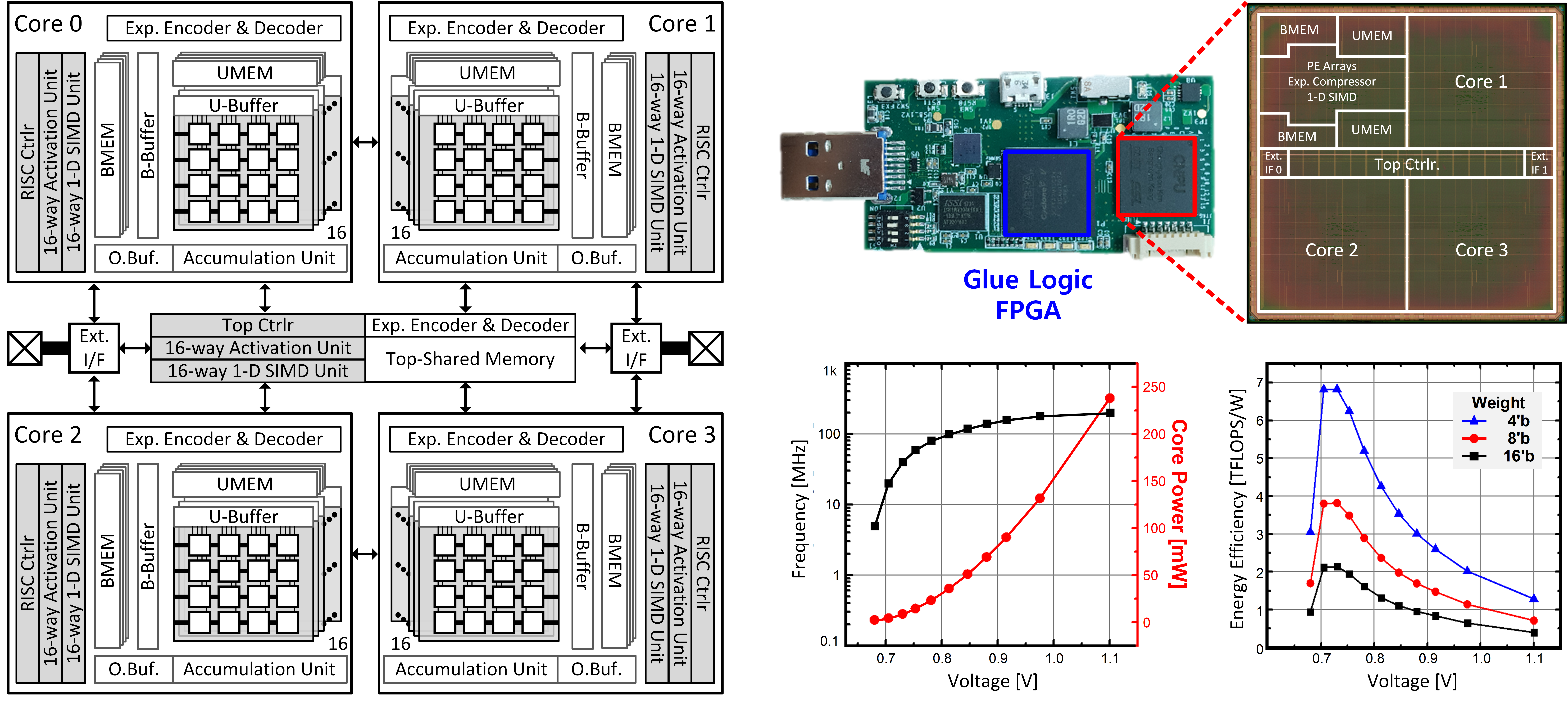