Title: Massive MIMO Channel Prediction: Kalman Filtering Vs. Machine Learning
Authors: Hwanjin Kim, Sucheol Kim, Hyeongtaek Lee, Junil Choi.
Journal: IEEE Transactions on Communications (published: January 2021)
Accurate channel state information (CSI) at the base stations (BSs) is crucial to fully exploit massive multiple-input multiple-output (MIMO) systems. The CSI at the BS can be outdated in the time-varying channel due to the mobility of user equipment (UE). The best way to solve the outdated CSI problem is to predict channels based on the prior CSI. In this paper, we develop the vector Kalman filter (VKF)-based predictor and the machine learning (ML)-based predictor using the spatial channel model (SCM), which is the realistic channels adopted in the 3GPP standard. First, we develop the VKF-based predictor using the autoregressive (AR) parameters from the SCM data based on the Yule-Walker equations. Then, we develop the ML-based channel predictor exploiting the linear minimum mean-square error (LMMSE)-based noise pre-processed data. Numerical results show that both channel predictors have significant gain over the outdated channel with regard to the prediction accuracy and data rate.
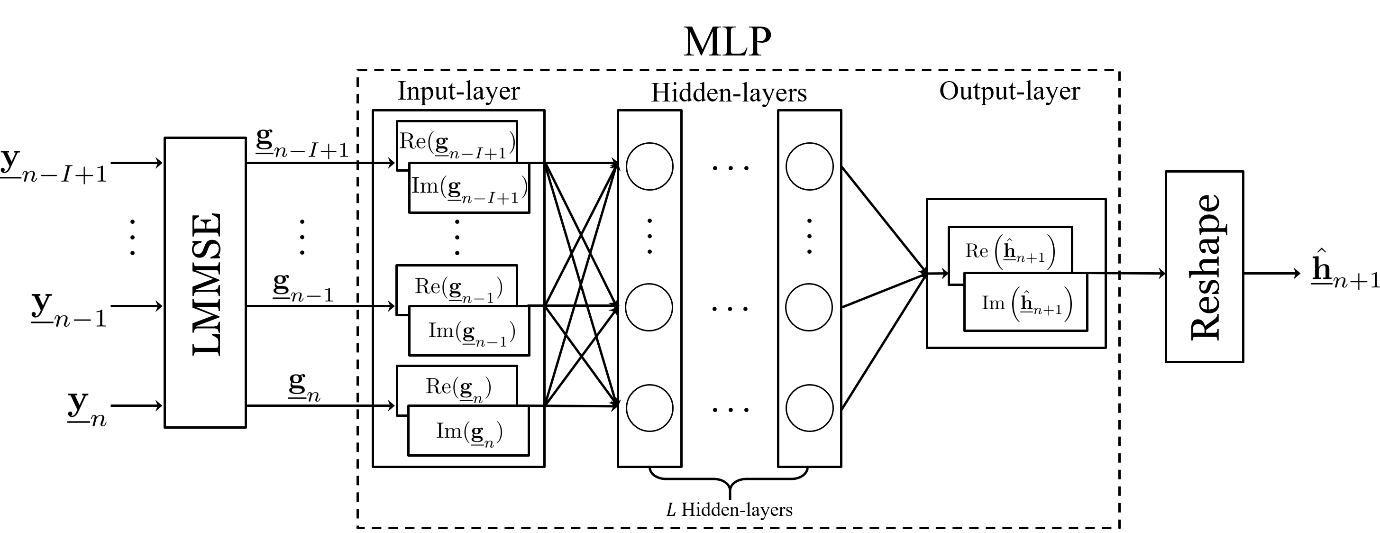
Figure 1. Multi-layer perceptron (MLP) structure with LMMSE pre-processing.