Abstract:
Personalized recommendation models (RecSys) are one of the most popular machine learning workload serviced by hyperscalers. A critical challenge of training RecSys is its high memory capacity requirements, reaching hundreds of GBs to TBs of model size. In RecSys, the so-called embedding layers account for the majority of memory usage so current systems employ a hybrid CPU-GPU design to have the large CPU memory store the memory hungry embedding layers. Unfortunately, training embeddings involve several memory bandwidth intensive operations which is at odds with the slow CPU memory, causing performance overheads. Prior work proposed to cache frequently accessed embeddings inside GPU memory as means to filter down the embedding layer traffic to CPU memory, but this paper observes several limitations with such cache design. In this work, we present a fundamentally different approach in designing embedding caches for RecSys. Our proposed ScratchPipe architecture utilizes unique properties of RecSys training to develop an embedding cache that not only sees the past but also the “future” cache accesses. ScratchPipe exploits such property to guarantee that the active working set of embedding layers can “always” be captured inside our proposed cache design, enabling embedding layer training to be conducted at GPU memory speed.
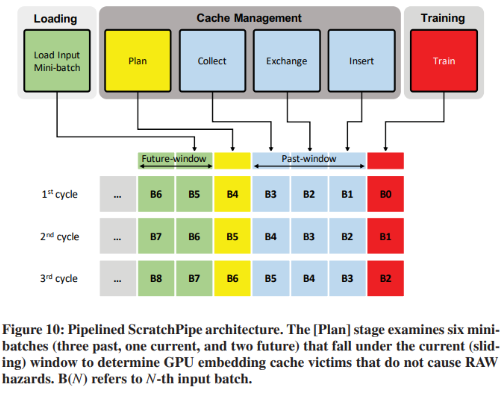
Abstract
Graph neural networks (GNNs) can extract features by learning both the representation of each objects (i.e., graph nodes) and the relationship across different objects (i.e., the edges that connect nodes), achieving state-of-the-art performance in various graph-based tasks. Despite its strengths, utilizing these algorithms in a production environment faces several challenges as the number of graph nodes and edges amount to several billions to hundreds of billions scale, requiring substantial storage space for training. Unfortunately, state-of-the-art ML frameworks employ an in-memory processing model which significantly hampers the productivity of ML practitioners as it mandates the overall working set to fit within DRAM capacity. In this work, we first conduct a detailed characterization on a state-of-the-art, large-scale GNN training algorithm, GraphSAGE. Based on the characterization, we then explore the feasibility of utilizing capacity-optimized NVMe SSDs for storing memory-hungry GNN data, which enables large-scale GNN training beyond the limits of main memory size. Given the large performance gap between DRAM and SSD, however, blindly utilizing SSDs as a direct substitute for DRAM leads to significant performance loss. We therefore develop SmartSAGE, our software/hardware co-design based on an in-storage processing (ISP) architecture. Our work demonstrates that an ISP based large-scale GNN training system can achieve both high capacity storage and high performance, opening up opportunities for ML practitioners to train large GNN datasets without being hampered by the physical limitations of main memory size.
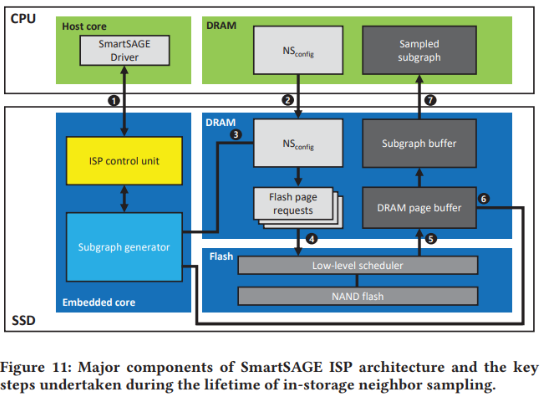
Geon Lee and Kijung Shin
ICDM 2021: IEEE International Conference on Data Mining 2021
Abstract: Group interactions arise in our daily lives (email communications, on-demand ride sharing, comment interactions on online communities, to name a few), and they together form hypergraphs that evolve over time. Given such temporal hypergraphs, how can we describe their underlying design principles? If their sizes and time spans are considerably different, how can we compare their structural and temporal characteristics?
In this work, we define 96 temporal hypergraph motifs (TH-motifs), and propose the relative occurrences of their instances as an answer to the above questions. TH-motifs categorize the relational and temporal dynamics among three connected hyperedges that appear within a short time. For scalable analysis, we develop THyMe+, a fast and exact algorithm for counting the instances of TH-motifs in massive hypergraphs, and show that THyMe+ is at most 2,163X faster while requiring less space than baseline. Using it, we investigate 11 real-world temporal hypergraphs from various domains. We demonstrate that TH-motifs provide important information useful for downstream tasks and reveal interesting patterns, including the striking similarity between temporal hypergraphs from the same domain.

Hyeonjeong Shin, Taehyung Kwon, Neil Shah, and Kijung Shin
WSDM 2022: International Conference on Web Search and Data Mining 2022
Abstract: A variety of tasks on dynamic graphs, including anomaly detection, community detection, compression, and graph understanding, have been formulated as problems of identifying constituent (near) bi-cliques (i.e., complete bipartite graphs). Even when we restrict our attention to maximal ones, there can be exponentially many near bi-cliques, and thus finding all of them is practically impossible for large graphs. Then, two questions naturally arise: (Q1) What is a “good” set of near bi-cliques? That is, given a set of near bi-cliques in the input dynamic graph, how should we evaluate its quality? (Q2) Given a large dynamic graph, how can we rapidly identify a high-quality set of near bi-cliques in it? Regarding Q1, we measure how concisely, precisely, and exhaustively a given set of near bi-cliques describes the input dynamic graph. We combine these three perspectives systematically on the Minimum Description Length principle. Regarding Q2, we propose CutNPeel, a fast search algorithm for a high-quality set of near bi-cliques. By adaptively re-partitioning the input graph, CutNPeel reduces the search space and at the same time improves the search quality. Our experiments using six real-world dynamic graphs demonstrate that CutNPeel is (a) High-quality: providing near bi-cliques of up to 51.2% better quality than its state-of-the-art competitors, (b) Fast: up to 68.8x faster than the next-best competitor, and (c) Scalable: scaling to graphs with 134 million edges. We also show successful applications of CutNPeel to graph compression and pattern discovery.
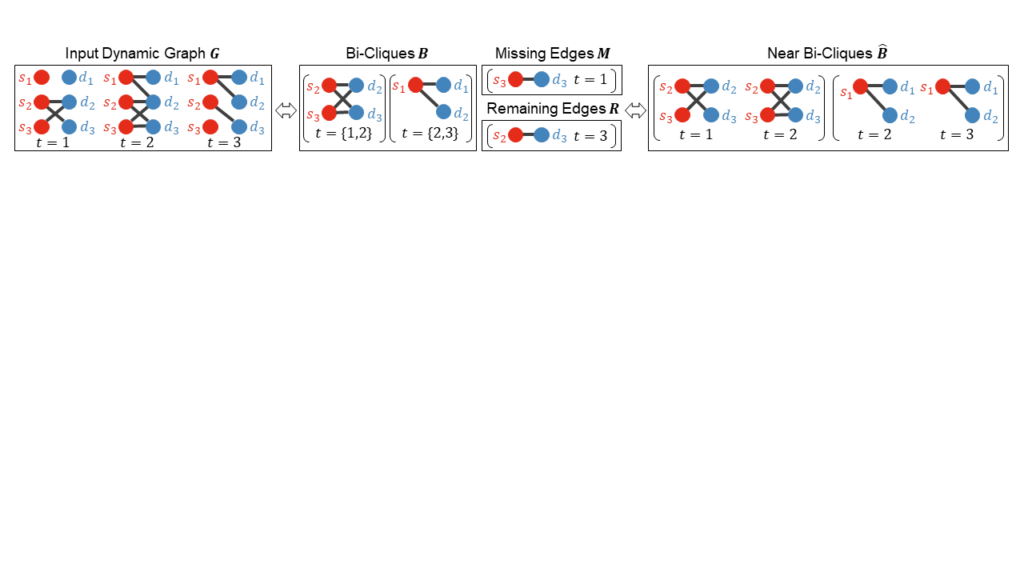
Hyunjin Choo and Kijung Shin
SDM 2022: SIAM International Conference on Data Mining 2022
Abstract: A hypergraph, which generalizes an ordinary graph, naturally represents group interactions as hyperedges (i.e., arbitrary-sized subsets of nodes). Such group interactions are ubiquitous: the sender and receivers of an email, the co-authors of a publication, and the items co-purchased by a customer, to name a few. A higher-order interaction (HOI) in a hypergraph is defined as the co-appearance of a set of nodes in any hyperedge. Our focus is the persistence of HOIs repeated over time, which is naturally interpreted as the strength of group relationships, aiming at answering three questions: (a) How do HOIs in real-world hypergraphs persist over time? (b) What are the key factors governing the persistence? (c) How accurately can we predict the persistence? In order to answer these questions, we investigate the persistence of HOIs in 13 real-world hypergraphs. First, we define how to measure the persistence of HOIs. Then, we examine global patterns and anomalies in the persistence, revealing a power-law relationship. After that, we study the relations between the persistence and 16 structural features of HOIs, some of which are closely related to the persistence. Lastly, based on the 16 structural features, we assess the predictability of the persistence under various settings and find strong predictors. Note that predicting the persistence of HOIs has many potential applications, such as recommending items to be purchased together and predicting missing recipients of emails.

Minyoung Choe, Jaemin Yoo, Geon Lee, Woonsung Baek, U Kang, and Kijung Shin
WWW 2022: The Web Conference 2022
Abstract: Graphs are widely used for representing pairwise interactions in complex systems. Since such real-world graphs are large and often evergrowing, sampling a small representative subgraph is indispensable for various purposes: simulation, visualization, stream processing, representation learning, crawling, to name a few. However, many complex systems consist of group interactions (e.g., collaborations of researchers and discussions on online Q&A platforms), and thus they can be represented more naturally and accurately by hypergraphs (i.e., sets of sets) than by ordinary graphs.
Motivated by the prevalence of large-scale hypergraphs, we study the problem of representative sampling from real-world hypergraphs, aiming to answer (Q1) what a representative sub-hypergraph is and (Q2) how we can find a representative one rapidly without an extensive search. Regarding Q1, we propose to measure the goodness of a sub-hypergraph by comparing it with the entire hypergraph in terms of ten graph-level, hyperedge-level, and node-level statistics. Regarding Q2, we first analyze the characteristics of six intuitive approaches in 11 real-world hypergraphs. Then, based on the analysis, we propose MiDaS, which draws hyperedges with a bias towards those with high-degree nodes. Through extensive experiments, we demonstrate that MiDaS is (a) Representative: finding overall the most representative samples among 13 considered approaches, (b) Fast: several orders of magnitude faster than the strongest competitors, which performs an extensive search, and (c) Automatic: rapidly searching a proper degree of bias.
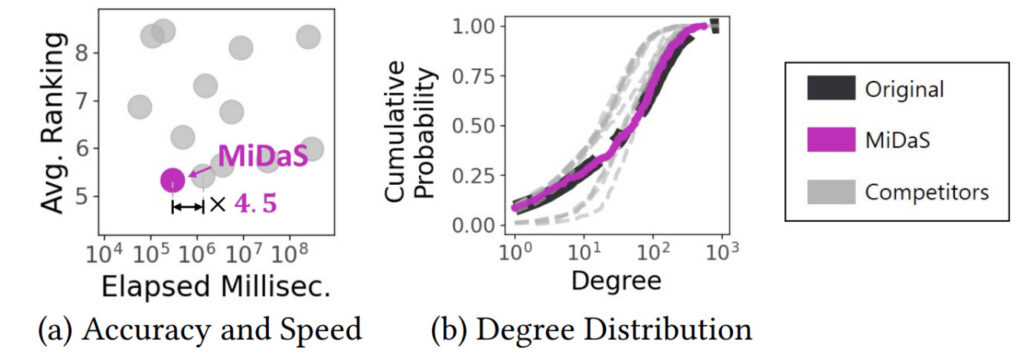
Kyuhan Lee*, Jihoon Ko*, and Kijung Shin
ICDE 2022: IEEE International Conference on Data Engineering 2022
Abstract: Given a massive graph, how can we exploit its hierarchical structure for concisely but exactly summarizing the graph? By exploiting the structure, can we achieve better compression rates than state-of-the-art graph summarization methods?
The explosive proliferation of the Web has accelerated the emergence of large graphs, such as online social networks and hyperlink networks. Consequently, graph compression has become increasingly important to process such large graphs without expensive I/O over the network or to disk. Among a number of approaches, graph summarization, which in essence combines similar nodes into a supernode and describe their connectivity concisely, protrudes with several advantages. However, we note that it fails to exploit pervasive hierarchical structures of real-world graphs as its underlying representation model enforces supernodes to be disjoint.
In this work, we propose the hierarchical graph summarization model, which is an expressive graph representation model that includes the previous one proposed by Navlakha et al. as a special case. The new model represents an unweighted graph using positive and negative edges between hierarchical supernodes, each of which can contain others. Then, we propose Slugger, a scalable heuristic for concisely and exactly representing a given graph under our new model. Slugger greedily merges nodes into supernodes while maintaining and exploiting their hierarchy, which is later pruned. Slugger significantly accelerates this process by sampling, approximation, and memoization. Our experiments on 16 real-world graphs show that Slugger is (a) Effective: yielding up to 29.6% more concise summary than state-of-the-art lossless summarization methods, (b) Fast: summarizing a graph with 0.8 billion edges in a few hours, and (c) Scalable: scaling linearly with the number of edges in the input graph.
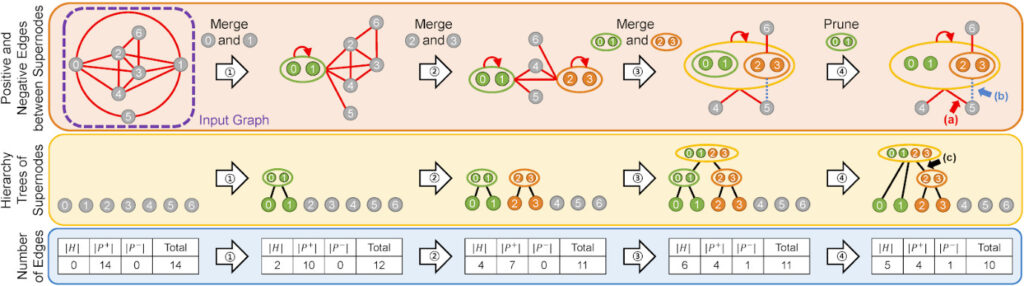
Shinhwan Kang, Kyuhan Lee, and Kijung Shin
ICDE 2022: IEEE International Conference on Data Engineering 2022
Abstract: Are users of an online social network interested equally in all connections in the network? If not, how can we obtain a summary of the network personalized to specific users? Can we use the summary for approximate query answering? As massive graphs (e.g., online social networks, hyperlink networks, and road networks) have become pervasive, graph compression has gained importance for the efficient processing of such graphs with limited resources. Graph summarization is an extensively-studied lossy compression method. It provides a summary graph where nodes with similar connectivity are merged into supernodes, and a variety of graph queries can be answered approximately from the summary graph. In this work, we introduce a new problem, namely personalized graph summarization, where the objective is to obtain a summary graph where more emphasis is put on connections closer to a given set of target nodes. Then, we propose PeGaSus, a linear-time algorithm for the problem. Through experiments on six real-world graphs, we demonstrate that PeGaSus is (a) Effective: node-similarity queries for target nodes can be answered significantly more accurately from personalized summary graphs than from non-personalized ones of similar size, (b) Scalable: it summarizes graphs with up to one billion edges, and (c) Applicable to distributed multi-query answering: it successfully replaces graph partitioning for communication-free multi-query processing.
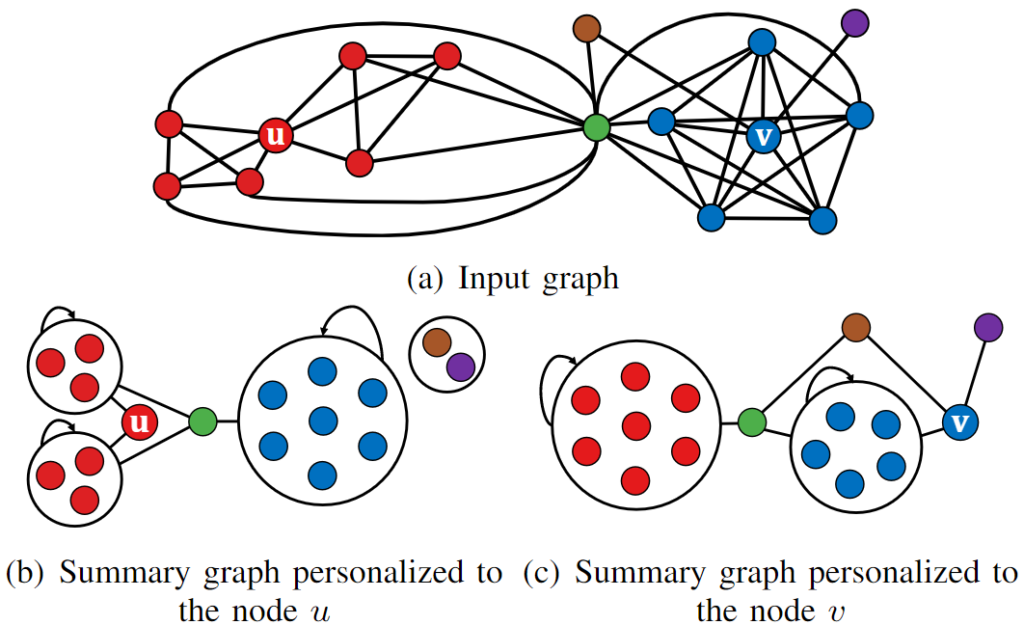
Shinhwan Kang, Kyuhan Lee, and Kijung Shin
PAKDD 2022: Pacific-Asia Conference on Knowledge Discovery and Data Mining 2022
Abstract: Which one is better between two representative graph summarization models with and without edge weights? From web graphs to online social networks, large graphs are everywhere. Graph summarization, which is an effective graph compression technique, aims to find a compact summary graph that accurately represents a given large graph. Two versions of the problem, where one allows edge weights in summary graphs and the other does not, have been studied in parallel without direct comparison between their underlying representation models. In this work, we conduct a systematic comparison by extending three search algorithms to both models and evaluating their outputs on eight datasets in five aspects: (a) reconstruction error, (b) error in node importance, (c) error in node proximity, (d) the size of reconstructed graphs, and (e) compression ratios. Surprisingly, using unweighted summary graphs leads to outputs significantly better in all the aspects than using weighted ones, and this finding is supported theoretically. Notably, we show that a state-of-the-art algorithm can be improved substantially (specifically, 8.2X, 7.8X, and 5.9X in terms of (a), (b), and (c), respectively, when (e) is fixed) based on the observation.
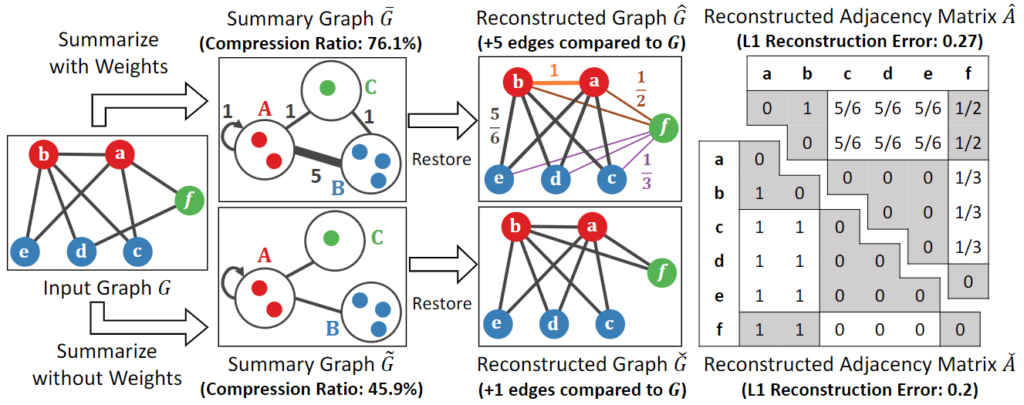
Hyunjin Hwang*, Seungwoo Lee*, Chanyoung Park, and Kijung Shin
SIGIR 2022: International ACM SIGIR Conference on Research and Development in Information Retrieval 2022
Abstract: Hypergraphs (i.e., sets of hyperedges) naturally represent group relations (e.g., researchers co-authoring a paper and ingredients used together in a recipe), each of which corresponds to a hyperedge (i.e., a subset of nodes). Predicting future or missing hyperedges bears significant implications for many applications (e.g., collaboration and recipe recommendation). What makes hyperedge prediction particularly challenging is the vast number of non-hyperedge subsets, which grows exponentially with the number of nodes. Since it is prohibitive to use all of them as negative examples for model training, it is inevitable to sample a very small portion of them, and to this end, heuristic sampling schemes have been employed. However, trained models suffer from poor generalization capability for examples of different natures. In this paper, we propose AHP, an adversarial training-based hyperedge-prediction method. It learns to sample negative examples without relying on any heuristic schemes. Using six real hypergraphs, we show that AHP generalizes better to negative examples of various natures. It yields up to 28.2% higher AUROC than the best existing methods and often even outperforms its variants with sampling schemes tailored to test sets.
