Title: That’s What I Said: Fully-Controllable Talking Face Generation
Authors: Y. Jang, K. Rho, J. Woo, H. Lee, J. Park, Y. Lim, B. Kim, J. S. Chung
Conference: ACM International Conference on Multimedia
Abstract: The goal of this paper is to synthesise talking faces with controllable facial motions. To achieve this goal, we propose two key ideas. The first is to establish a canonical space where every face has the same motion patterns but different identities. The second is to navigate a multimodal motion space that only represents motion-related features while eliminating identity information. To disentangle identity and motion, we introduce an orthogonality constraint between the two different latent spaces. From this, our method can generate natural-looking talking faces with fully controllable facial attributes and accurate lip synchronisation. Extensive experiments demonstrate that our method achieves state-of-the-art results in terms of both visual quality and lip-sync score. To the best of our knowledge, we are the first to develop a talking face generation framework that can accurately manifest full target facial motions including lip, head pose, and eye movements in the generated video without any additional supervision beyond RGB video with audio.
Main Figure: 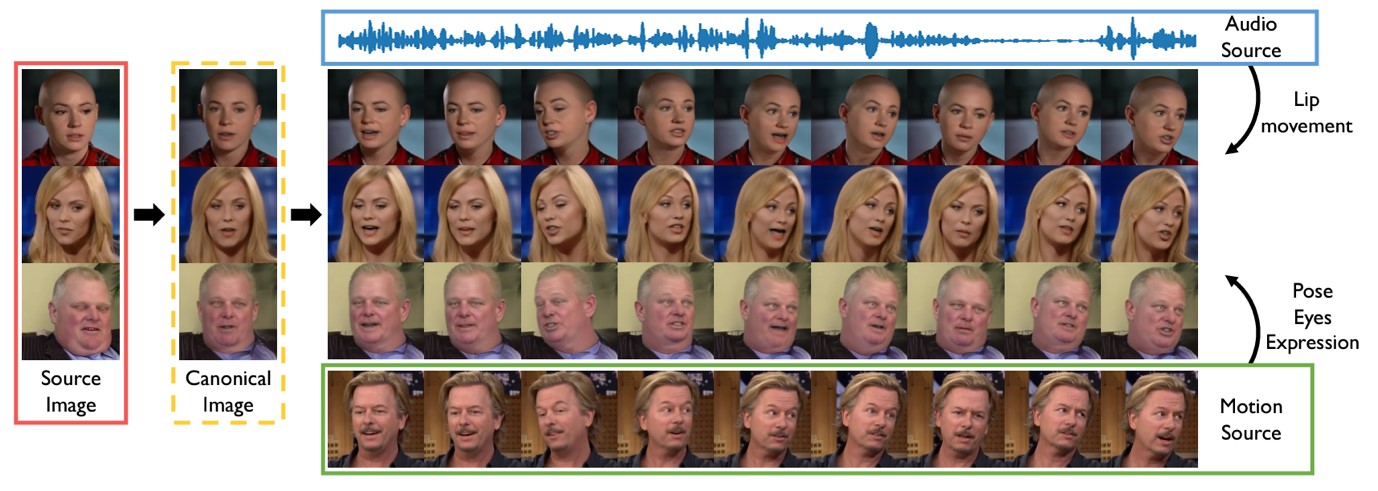
Title: Sound Source Localization is All about Cross-Modal Alignment
Authors: A. Senocak, H. Ryu, J. Kim, T. Oh, H. Pfister, J. S. Chung
Conference: International Conference on Computer Vision
Abstract: Humans can easily perceive the direction of sound sources in a visual scene, termed sound source localization. Recent studies on learning-based sound source localization have mainly explored the problem from a localization perspective. However, prior arts and existing benchmarks do not account for a more important aspect of the problem, cross-modal semantic understanding, which is essential for genuine sound source localization. Cross-modal semantic understanding is important in understanding semantically mismatched audio-visual events, e.g., silent objects, or offscreen sounds. To account for this, we propose a crossmodal alignment task as a joint task with sound source localization to better learn the interaction between audio and visual modalities. Thereby, we achieve high localization performance with strong cross-modal semantic understanding. Our method outperforms the state-of-the-art approaches in both sound source localization and cross-modal retrieval. Our work suggests that jointly tackling both tasks is necessary to conquer genuine sound source localization.
Main Figure:
Title: FlexiAST: Flexibility is What AST Needs
Authors: J. Feng, M. H. Erol, J. S. Chung, A. Senocak
Conference: Interspeech
Abstract: The objective of this work is to give patch-size flexibility to Audio Spectrogram Transformers (AST). Recent advancements in ASTs have shown superior performance in various audio-based tasks. However, the performance of standard ASTs degrades drastically when evaluated using different patch sizes from that used during training. As a result, AST models are typically re-trained to accommodate changes in patch sizes. To overcome this limitation, this paper proposes a training procedure to provide flexibility to standard AST models without architectural changes, allowing them to work with various patch sizes at the inference stage- FlexiAST. This proposed training approach simply utilizes random patch size selection and resizing of patch and positional embedding weights. Our experiments show that FlexiAST gives similar performance to standard AST models while maintaining its evaluation ability at various patch sizes on different datasets for audio classification tasks.
Main Figure: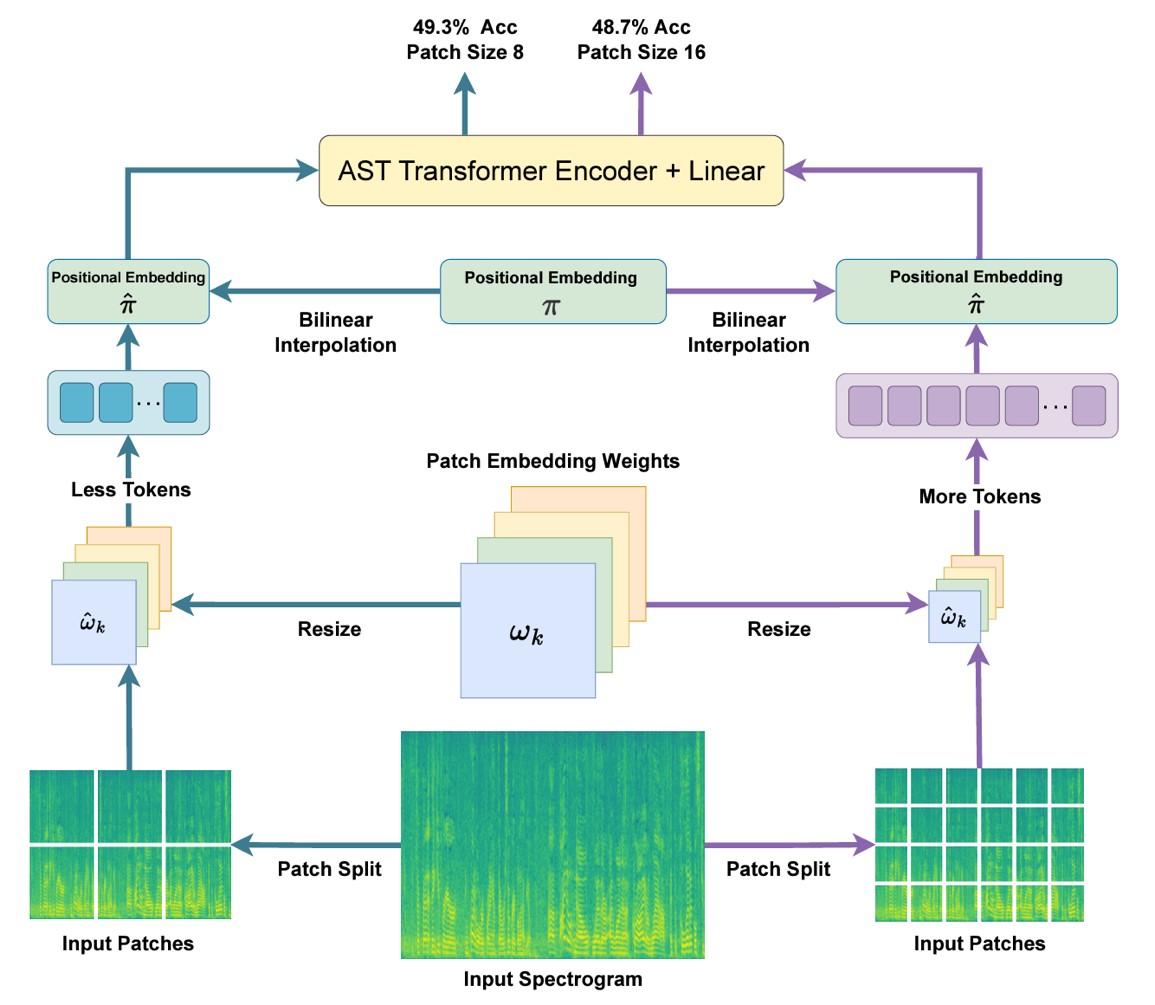
Title: Disentangled Representation Learning for Multilingual Speaker Recognition
Authors: K. Nam, Y. Kim, J. Huh, H. Heo, J. Jung, J. S. Chung
Conference: Interspeech
Abstract: The goal of this paper is to learn robust speaker representation for bilingual speaking scenario. The majority of the world’s population speak at least two languages; however, most speaker recognition systems fail to recognise the same speaker when speaking in different languages. Popular speaker recognition evaluation sets do not consider the bilingual scenario, making it difficult to analyse the effect of bilingual speakers on speaker recognition performance. In this paper, we publish a large-scale evaluation set named VoxCeleb1-B derived from VoxCeleb that considers bilingual scenarios. We introduce an effective disentanglement learning strategy that combines adversarial and metric learning-based methods. This approach addresses the bilingual situation by disentangling language-related information from speaker representation while ensuring stable speaker representation learning. Our languagedisentangled learning method only uses language pseudo-labels without manual information.
Main Figure: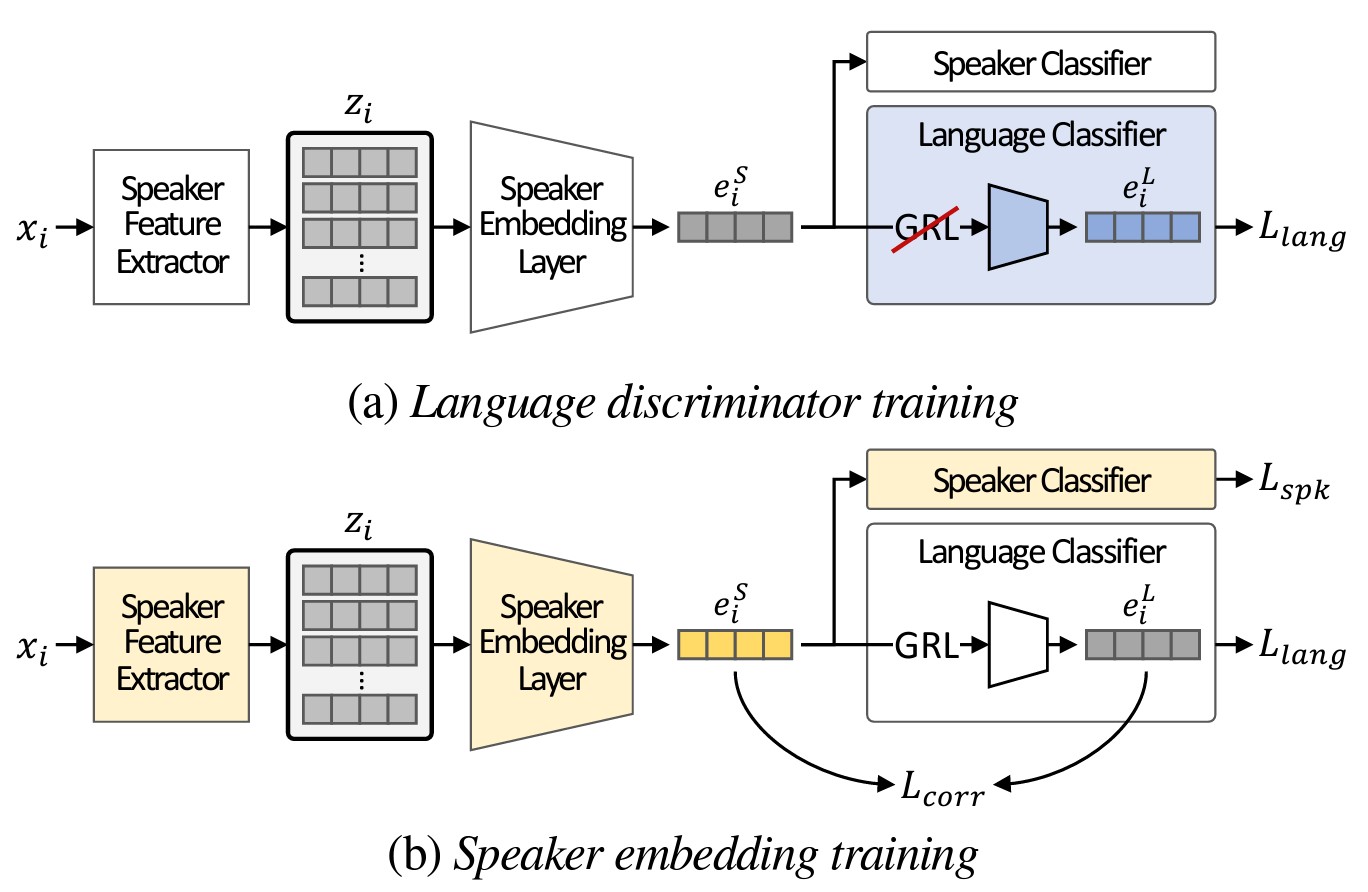
Title: Curriculum learning for self-supervised speaker verification
Authors: H. Heo, J. Jung, J. Kang, Y. Kwon, B. Lee, Y. J. Kim, J. S. Chung
Conference: Interspeech
Abstract: The goal of this paper is to train effective self-supervised speaker representations without identity labels. We propose two curriculum learning strategies within a self-supervised learning framework. The first strategy aims to gradually increase the number of speakers in the training phase by enlarging the used portion of the train dataset. The second strategy applies various data augmentations to more utterances within a mini-batch as the training proceeds. A range of experiments conducted using the DINO self-supervised framework on the VoxCeleb1 evaluation protocol demonstrates the effectiveness of our proposed curriculum learning strategies. We report a competitive equal error rate of 4.47% with a single-phase training, and we also demonstrate that the performance further improves to 1.84% by f ine-tuning on a small labelled dataset.
Main Figure: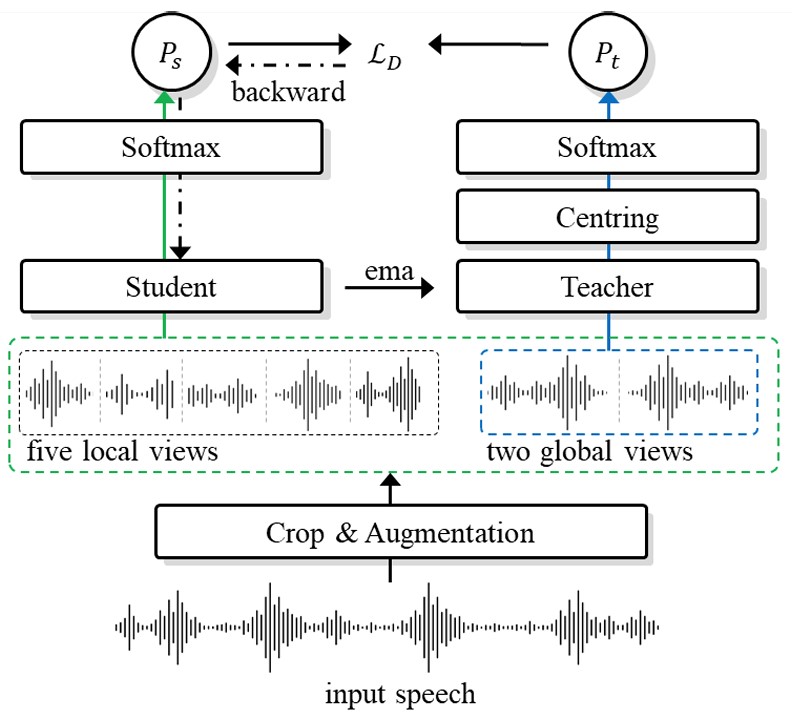
Title: Self-sufficient framework for continuous sign language recognition
Authors: Y. Jang, Y. Oh, J. W. Cho, M. Kim, D. Kim, I. S. Kweon, J. S. Chung
Conference: International Conference on Acoustics, Speech, and Signal Processing
Abstract: The goal of this work is to develop self-sufficient framework for Continuous Sign Language Recognition (CSLR) that addresses key issues of sign language recognition. These include the need for complex multi-scale features such as hands, face, and mouth for understanding, and absence of frame-level annotations. To this end, we propose (1) Divide and Focus Convolution (DFConv) which extracts both manual and non-manual features without the need for additional networks or annotations, and (2) Dense Pseudo-Label Refinement (DPLR) which propagates non-spiky frame-level pseudo-labels by combining the ground truth gloss sequence labels with the predicted sequence. We demonstrate that our model achieves state-of-the-art performance among RGB-based methods on large-scale CSLR benchmarks, PHOENIX-2014 and PHOENIX-2014-T, while showing comparable results with better efficiency when compared to other approaches that use multi-modality or extra annotations.
Main Figure:
Title: Metric learning for user-defined keyword spotting
Authors: J. Jung, Y. Kim, J. Park, Y. Lim, B. Kim, Y. Jang, J. S. Chung
Conference: International Conference on Acoustics, Speech, and Signal Processing
Abstract: The goal of this work is to detect new spoken terms defined by users. While most previous works address Keyword Spotting (KWS) as a closed-set classification problem, this limits their transferability to unseen terms. The ability to define custom keywords has advantages in terms of user experience. In this paper, we propose a metric learning-based training strategy for user-defined keyword spotting. In particular, we make the following contributions: (1) we construct a large-scale keyword dataset with an existing speech corpus and propose a filtering method to remove data that degrade model training; (2) we propose a metric learning-based two-stage training strategy, and demonstrate that the proposed method improves the performance on the user-defined keyword spotting task by enriching their representations; (3) to facilitate the fair comparison in the user-defined KWSfield, we propose unified evaluation protocol and metrics. Our proposed system does not require an incremental training on the user-defined keywords, and outperforms previous works by a significant margin on the Google Speech Commands dataset using the proposed as well as the existing metrics.
Main Figure: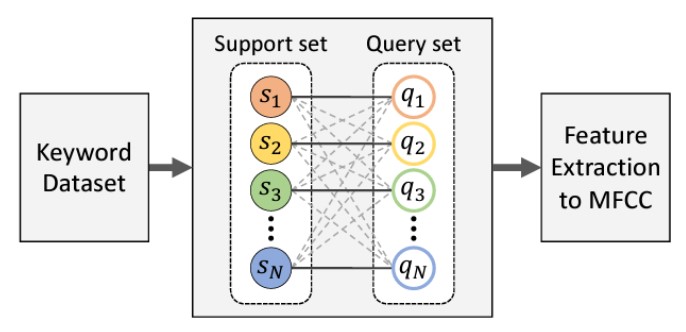
Title: Hindi as a second language: improving visually grounded speech with semantically similar samples
Authors: H. Ryu, A. Senocak, I. S. Kweon, J. S. Chung
Conference: International Conference on Acoustics, Speech, and Signal Processing
Abstract: The objective of this work is to explore the learning of visually grounded speech models (VGS) from multilingual perspective. Bilingual VGS models are generally trained with an equal number of spoken captions from both languages. However, in reality, there can be an imbalance among the languages for the available spoken captions. Our key contribution in this work is to leverage the power of a high-resource language in a bilingual visually grounded speech model to improve the performance of a low-resource language. We introduce two methods to distill the knowledge of high-resource language into low-resource languages: (1) incorporating a strong pre-trained high-resource language encoder and (2) using semantically similar spoken captions. Our experiments show that combining these two approaches effectively enables the low-resource language to surpass the performances of monolingual and bilingual counterparts for cross-modal retrieval tasks.
Main Figure: 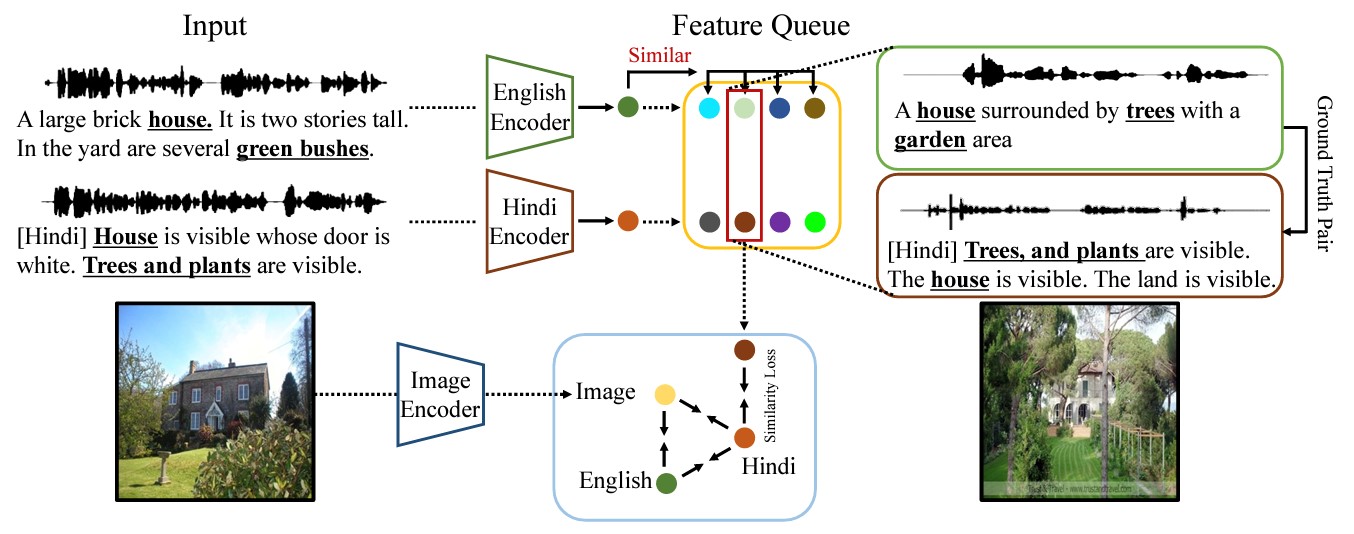
Title: MarginNCE: Robust Sound Localization with a Negative Margin
Authors: S. Park, A. Senocak, J. S. Chung
Conference: International Conference on Acoustics, Speech, and Signal Processing
Abstract: The goal of this work is to localize sound sources in visual scenes with a self-supervised approach. Contrastive learning in the context of sound source localization leverages the natural correspondence between audio and visual signals where the audio-visual pairs from the same source are assumed as positive, while randomly selected pairs are negatives. However, this approach brings in noisy correspondences; for example, positive audio and visual pair signals that may be unrelated to each other, or negative pairs that may contain semantically similar samples to the positive one. Our key contribution in this work is to show that using a less strict decision boundary in contrastive learning can alleviate the effect of noisy correspondences in sound source localization. We propose a simple yet effective approach by slightly modifying the contrastive loss with a negative margin. Extensive experimental results show that our approach gives on-par or better performance than the state-of-the-art methods. Furthermore, we demonstrate that the introduction of a negative margin to existing methods results in a consistent improvement in performance.
Main Figure:
Main Figure: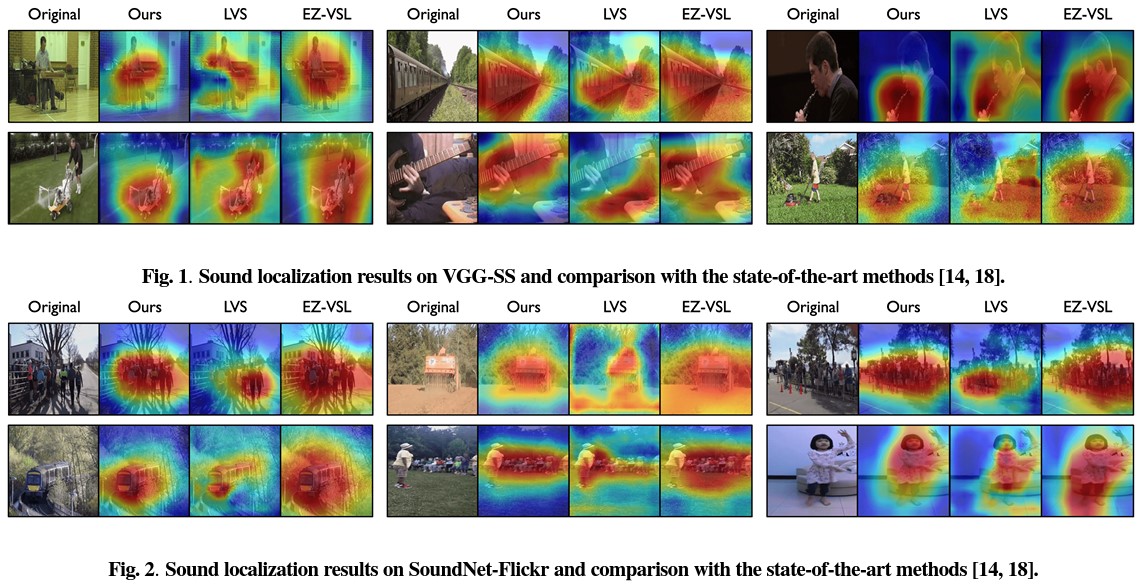
Title: Advancing the dimensionality reduction of speaker embeddings for speaker diarisation: disentangling noise and informing speech activity
Authors: Y. J. Kim, H. Heo, J. Jung, Y. Kwon, B. Lee, J. S. Chung
Conference: International Conference on Acoustics, Speech, and Signal Processing
Abstract: The objective of this work is to train noise-robust speaker embeddings adapted for speaker diarisation. Speaker embeddings play a crucial role in the performance of diarisation systems, but they often capture spurious information such as noise, adversely affecting performance. Our previous work has proposed an auto-encoder-based dimensionality reduction module to help remove the redundant information. However, they do not explicitly separate such information and have also been found to be sensitive to hyper-parameter values. To this end, we propose two contributions to overcome these issues: (i) a novel dimensionality reduction framework that can disentangle spurious information from the speaker embeddings; (ii) the use of speech activity vector to prevent the speaker code from representing the background noise. Through a range of experiments conducted on four datasets, our approach consistently demonstrates the state-of-the-art performance among models without system fusion.
Main Figure:
Main Figure: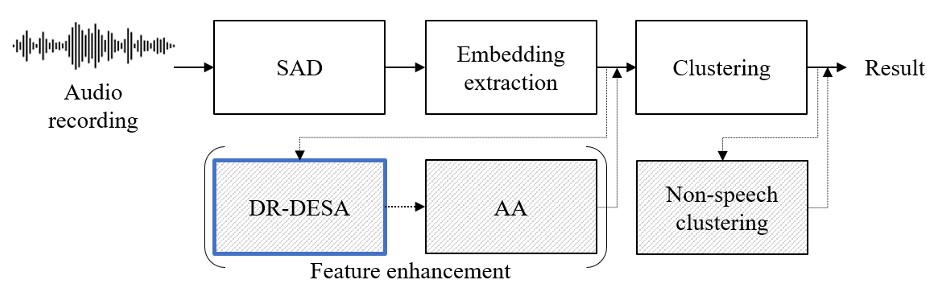