Research team of EE Prof. Myoungsoo Jung, Shinhyun Choi, Wanyeong Jung was selected as Samsung’s Future Technology development of 2021
Professors of School of Electrical Engineering Prof. Myoungsoo Jung, Shinhyun Choi, Wanyeong Jung ‘s research project was selected as Samsung’s Future Technology Development of 2021. The project covers a wide range of fields including computer architecture, AI frameworks, operating systems, circuits and devices. It is the first ICT convergence creative project with multidisciplinary project that covers device-circuit-systems in the Future Technology Development .
The details of the selected project is as follows:
Field
|
Project name
|
Project Manager
|
ICT Convergence creative project
|
Heterogeneous new memory-based hardware and system software framework for accelerating graph neural network based machine learning.
|
Myoungsoo Jung
|
The research team focuses on speeding up the popular GNN based machine learning model that uses relationship information in graph data. The project tries to solve the fundamental problems of GNNs by providing solutions from the device, circuit, architecture and operating system level.
The selection of the project is significant in that it suggests a multidisciplinary cooperation between device, circuit and computer science experts and builds a practical solution to the problem.
Samsung Elecgronics has been selecting Future Technology proejcts each year since 2014. Samsung Electronics selects vital technological projects that is necessary for the future of the country from fields of natural science, information communication technology.
Congratuations and a great thanks to the professors.
M.S. student So-Yeon Kim (Advised by Hoi-Jun Yoo) won the Best Design Award at the 2020 IEEE Asian Solid-State Circuits Conference (A-SSCC) Student Design Contest.
Due to COVID-19, the conference was held online from November 9th to 11th.
A-SSCC is an international conference held annually by IEEE. M.S. student So-Yeon Kim has published a paper titled “An Energy-Efficient GAN Accelerator with On-Chip Training for Domain-Specific Optimization” and was selected as a winner for its excellence.
Research Officers for National Defense.
Details are as follows. Congratulations once again to M.S. student So-Yeon Kim and Professor Hoi-Jun Yoo!
Conference: 2020 IEEE Asian Solid-State Circuits Conference (A-SSCC)
Location: Virtual Event
Date: November 9-11, 2020
Award: Student Best Design Award
Authors: So-Yeon Kim, Sang-Hoon Kang, Dong-Hyeon Han, Sang-Yeop Kim, Sang-Jin Kim, and Hoi-Jun Yoo (Advisory Professor)
Paper Title: An Energy-Efficient GAN Accelerator with On-Chip Training for Domain-Specific Optimization
Our department’s professor Youngsoo Shin along with professor Jong-won Yu, Chang Dong Yoo, Jung-Yong Lee, Myoungsoo Jung, Song Min Kim, and Junil Choi have been rewarded the 2020 engineering school technology innovation award.
Each school selects and awards staff members for their contributions, and the engineering school has been awarding staff members who have greatly contributed to technology innovation and academic progress. Special awards are given to members for their development of world class technologies, significant academic achievements and industry-education cooperation.
This year’s award was given to a total of 28 members including one grand award, and 7 professors from our department have received the award.
A total of 3,100,000 KW will be given along with the award.
We congratulate the 7 professors for their achievements and contribution to KAIST.
KAIST ITRC Artificial Intelligence Semiconductor System Research Center (Center Director: Professor Joo-Young Kim) will be launched. It was newly selected as the 2020 university ICT research center project, and the project is managed by the Institute of Information & Communications Technology Planning & Evaluation (IITP) under the Ministry of Science and ICT. Professor Joo-Young Kim plans to lead the research project by 2025 with a total of 5 billion won in research funding with a goal of ‘Development of semiconductor system convergence innovation technology for a non-face-to-face/artificial intelligence society’.
The research center is located in Daejeon as a base research center, connecting Seoul, Daejeon, and Ulsan, and plans to conduct joint research with Yonsei University, Ewha Womans University, and Ulsan National Institute of Science and Technology (UNIST).
The opening ceremony of the research center is scheduled to take place this Friday at 10:30 am. This opening ceremony will be held online to prevent the spread of the coronavirus.
Our department’s professor Yoo Hoi-Jun’s lab has developed a low power GAN (generative Adversarial Network) AI semiconductor chip.
The developed AI semiconductor chip can process multi-deep layer neural networks on low power, making it suitable for mobile platforms. The team has succeeded in performing image combination, style transform, recovery of damaged images and other generative AI technologies on a mobile device with the developed chip.
1st author Ph.D candidate Sanghoon Kang presented the research development on February 17 at the ISSCC conference, where approximately 3000 researchers from around the world gathered in San Francisco(Paper title : GANPU: A 135TFLOPS/W Multi-DNN Training Processor for GANs with Speculative Dual-Sparsity Exploitation). Recent developments are focused on the acceleration and performance of artificial intelligence on mobile platforms, but past researches are limited to single-deep layer neural networks and applicable only at the inference stage, which are not appropriate for mobile platforms. The team has shown that not only single-deep but also multi-deep layer neural networks such as generative adversarial networks can be performed on mobile devices via the GANPU(Generative Adversarial Networks Processing Unit) AI semiconductor chip.
The chip can train the GAN neural network on the processor itself without having to send the data to an external server, which can provide enhanced private data security. A 4.8-fold higher energy efficiency was achieved compared to previous multi-deep layer neural network devices.
The team has performed an automatic face changing application on a mobile tablet PC device. The developed application provides facial correction by designating the addition/transform/delete points of the 17 characteristics of the face, which then the GANPU networks automatically applies the corrections.
[Link]
https://www.ytn.co.kr/_ln/0115_202004070308303992
https://news.kaist.ac.kr/news/html/news/?mode=V&mng_no=6831
Title: 1.32 TOPS/W Energy Efficient Deep Neural Network Learning Processor with Direct Feedback Alignment based Heterogeneous Core Architecture
Authors: Dong-Hyeon Han, Jin-Su Lee, Jin-Mook Lee and Hoi-Jun Yoo
An energy efficient deep neural network (DNN) learning processor is proposed using direct feedback alignment (DFA).
The proposed processor achieves 2.2 × faster learning speed compared with the previous learning processors by the pipelined DFA (PDFA). Since the computation direction of the back-propagation (BP) is reversed from the inference, the gradient of the 1st layer cannot be generated until the errors are propagated from the last layer to the 1st layer. On the other hand, the proposed processor applies DFA which can propagate the errors directly from the last layer. This means that the PDFA can propagate errors during the next inference computation and that weight update of the 1st layer doesn’t need to wait for error propagation of all the layers. In order to enhance the energy efficiency by 38.7%, the heterogeneous learning core (LC) architecture is optimized with the 11-stage pipeline data-path. It show 2 × longer data reusing compared with the conventional BP. Furthermore, direct error propagation core (DEPC) utilizes random number generators (RNG) to remove external memory access (EMA) caused by error propagation (EP) and improve the energy efficiency by 19.9%.
The proposed PDFA based learning processor is evaluated on the object tracking (OT) application, and as a result, it shows 34.4 frames-per-second (FPS) throughput with 1.32 TOPS/W energy efficiency.
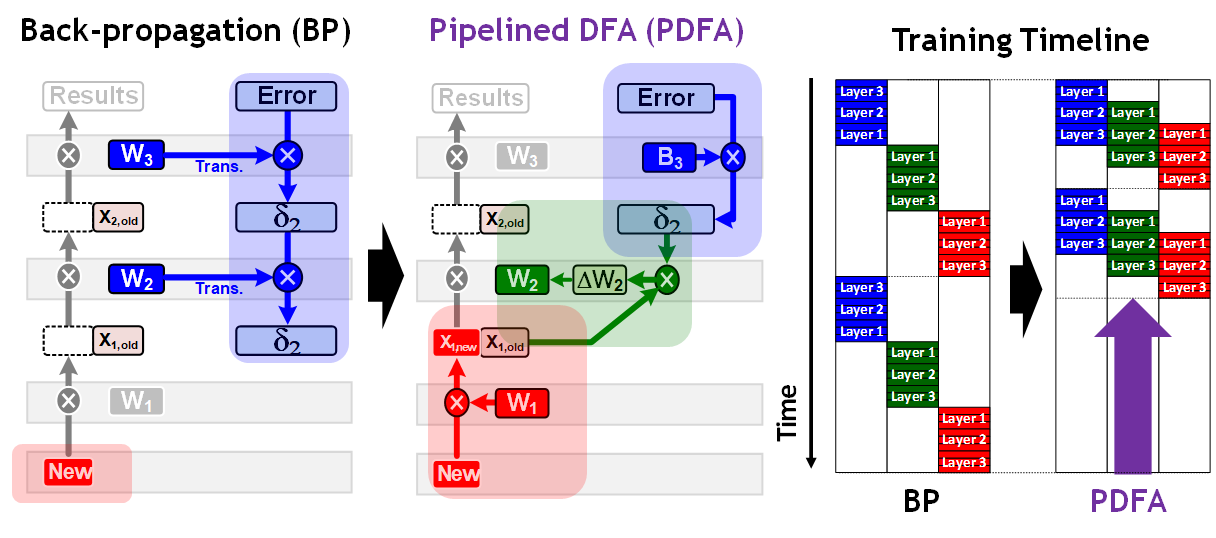
Figure 1. Back-propagation vs Pipelined DFA
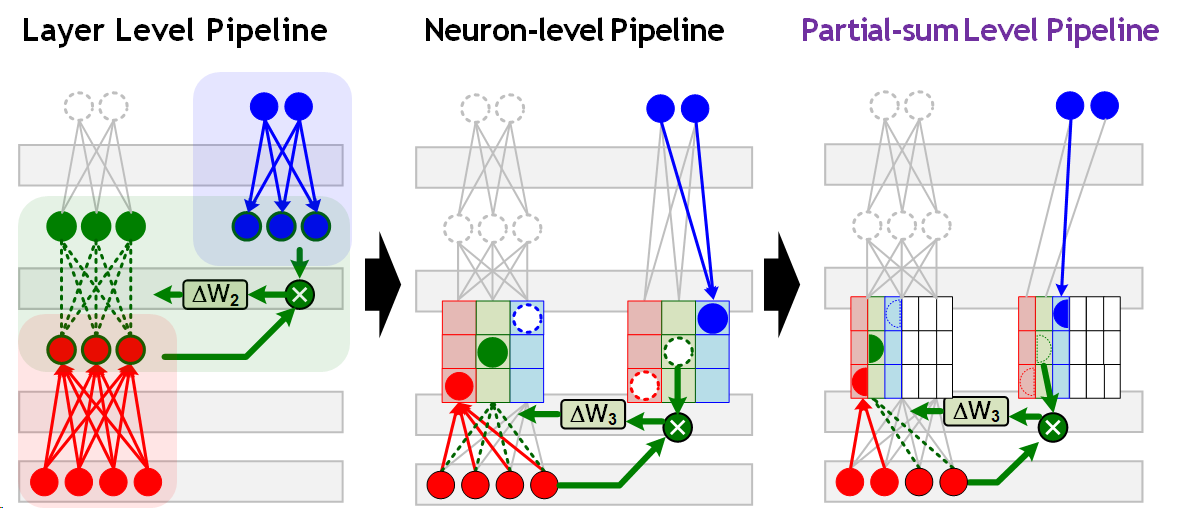
Figure 2. Layer Level vs Neuron-level vs Partial-sum Level Pipeline
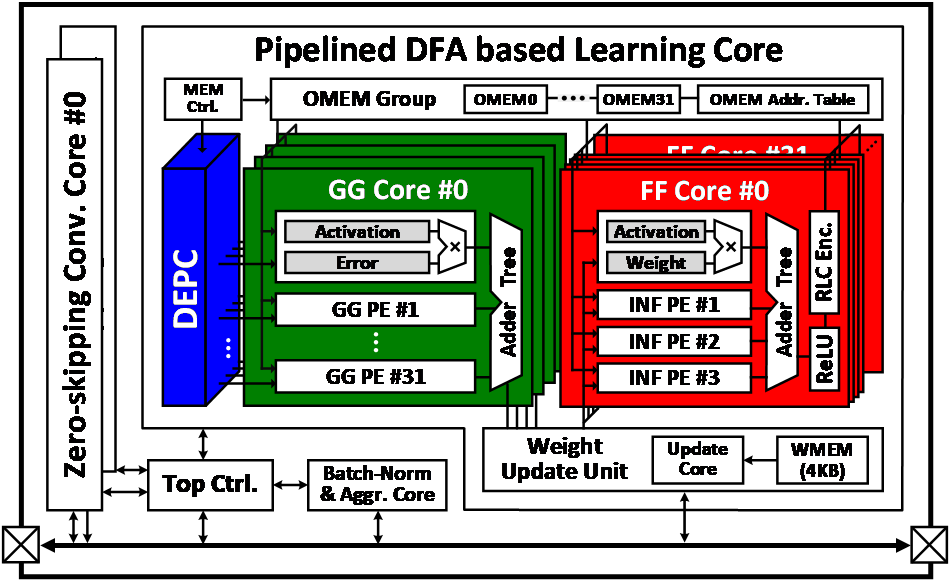
Figure 3. Overall Architecture of Proposed Processor
Title: LNPU: A 25.3TFLOPS/W Sparse Deep-Neural-Network Learning Processor with Fine-Grained Mixed Precision of FP8-FP16
Authors: Jin-Su Lee, Ju-Hyoung Lee, Dong-Hyeon Han, Jin-Mook Lee, Gwang-Tae Park, and Hoi-Jun Yoo
Recently, deep neural network (DNN) hardware accelerators have been reported for energy-efficient deep learning (DL) acceleration. Most of the previous DNN inference accelerators trained their DNN parameters at the cloud server using public datasets and downloaded the parameters to them to implement AI. However, the local DNN learning with domain-specific and private data is required to adapt to various user’s preferences on the edge or mobile devices. Since the edge and mobile devices contain only limited computation capability with battery power, energy-efficient DNN learning processor is necessary. In this paper, we present an energy-efficient on-chip learning accelerator. Its data precision is optimized while maintaining the training accuracy with fine-grained mixed precision (FGMP) of FP8-FP16 to reduce external memory access (EMA) and to enhance throughput with high accuracy. In addition, sparsity is exploited with intra-channel accumulation as well as inter-channel accumulation to support 3 DNN learning steps with higher throughput to enhance energy-efficiency. Also, the input load balancer (ILB) is integrated to improve PE utilization under the unbalanced amount of input data caused by irregular sparsity. The external memory access is reduced by 38.9% and energy-efficiency is improved 2.08 times for ResNet-18 training. The fabricated chip occupies 16mm2 in 65nm CMOS and the energy efficiency is 3.48TFLOPS/W (FP8) for 0.0% sparsity and 25.3TFLOPS/W (FP8) for 90% sparsity.
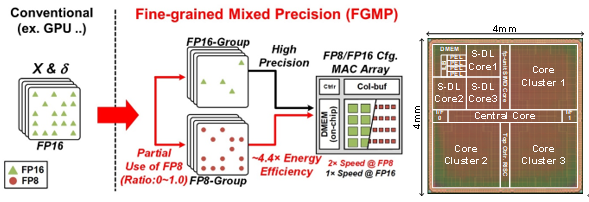
Title: A 2.1TFLOPS/W Mobile Deep RL Accelerator with Transposable PE Array and Experience Compression
Authors: Chang-Hyeon Kim, Sang-Hoon Kang, Don-Joo Shin, Sung-Pill Choi, Young-Woo Kim and Hoi-Jun Yoo
Recently, deep neural networks (DNNs) are actively used for action control so that an autonomous system, such as the robot, can perform human-like behaviors and operations. Unlike recognition tasks, the real-time operation is essential in action control, and it is too slow to use remote learning on a server communicating through a network. New learning techniques, such as reinforcement learning (RL), are needed to determine and select the correct robot behavior locally. In this paper, We propose DRL accelerator with transposable PE array and experience compressor to realize real-time DRL operation of autonomous agents in dynamic environments. It supports on-chip data compression and decompression that ~10,000 of DRL experiences can be compressed by 65%. And it enables adaptive data reuse for inferencing and training, which results in power and peak memory bandwidth reduction by 31% and 41%, respectively. The proposed DRL accelerator is fabricated with 65nm CMOS technology and occupies 4×4 mm2 die area. This is the first fully trainable DRL processor, and it achieves 2.16 TFLOPS/W energy-efficiency at 0.73V with 16b weights@50MHz.
Fig. 1. DRL Accelerator with tPE Array, Implementation & Measurement Results
Title: CNNP-v2: An Energy Efficient Memory-Centric Convolutional Neural Network Processor Architecture
Authors: Sung-Pill Choi, Kyeong-Ryeol Bong, Dong-Hyeon Han, and Hoi-Jun Yoo
An energy efficient memory-centric convolution-al neural network (CNN) processor architecture is proposed for smart devices such as wearable devices or internet of things (IoT) devices. To achieve energy-efficient processing, it has 2 key features: First, 1-D shift convolution PEs with fully distributed memory architecture achieve 3.1TOPS/W energy efficiency. Compared with conventional architecture, even though it has massively parallel 1024 MAC units, it achieve high energy efficiency by scaling down voltage to 0.46V due to its fully local routed design. Next, fully configurable 2-D mesh core-to-core interconnection support various size of input features to maximize utilization. The proposed architecture is evaluated 16mm2 chip which is fabricated with 65nm CMOS process and it performs real-time face recognition with only 9.4mW at 10MHz and 0.48V.
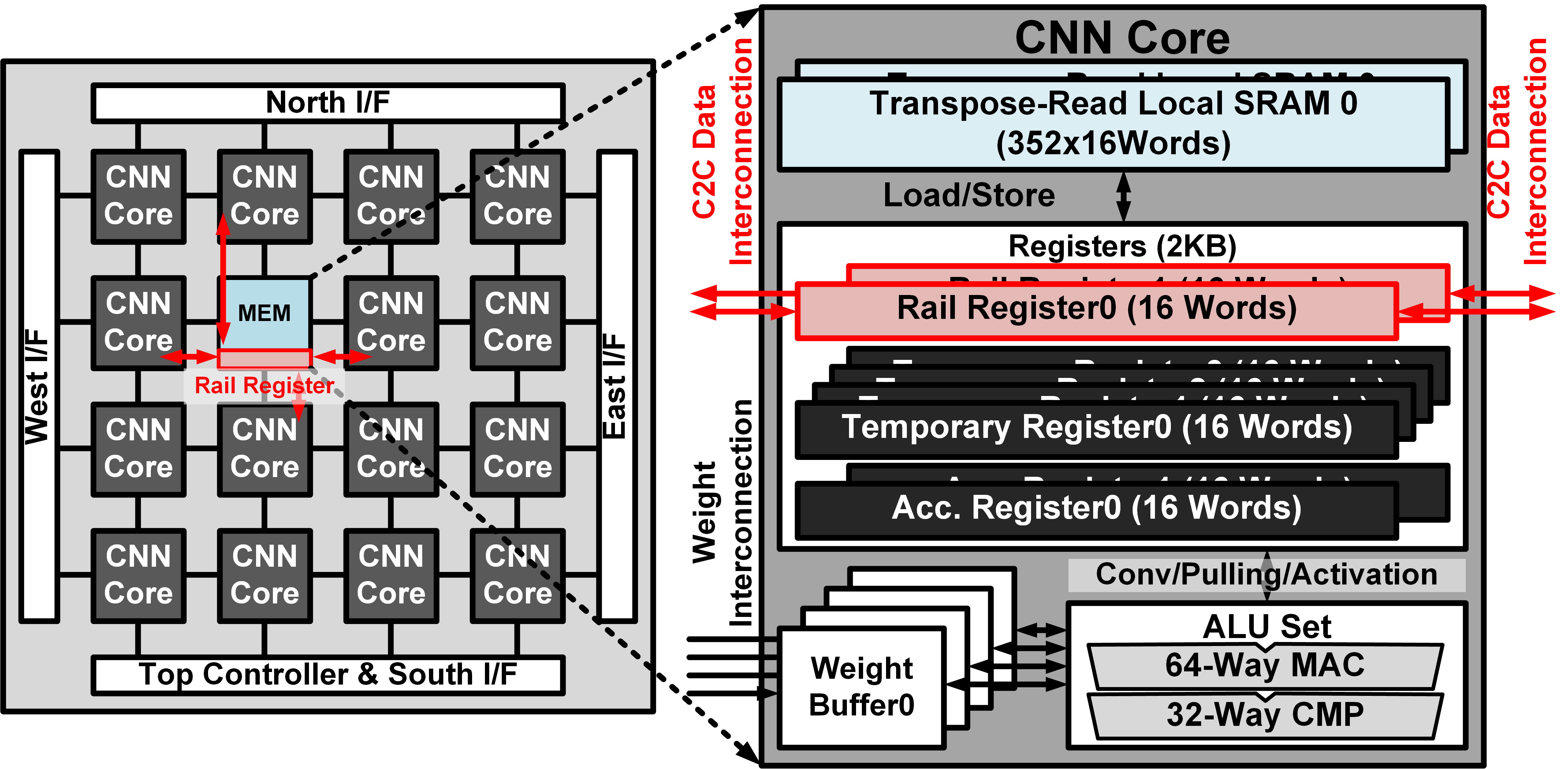
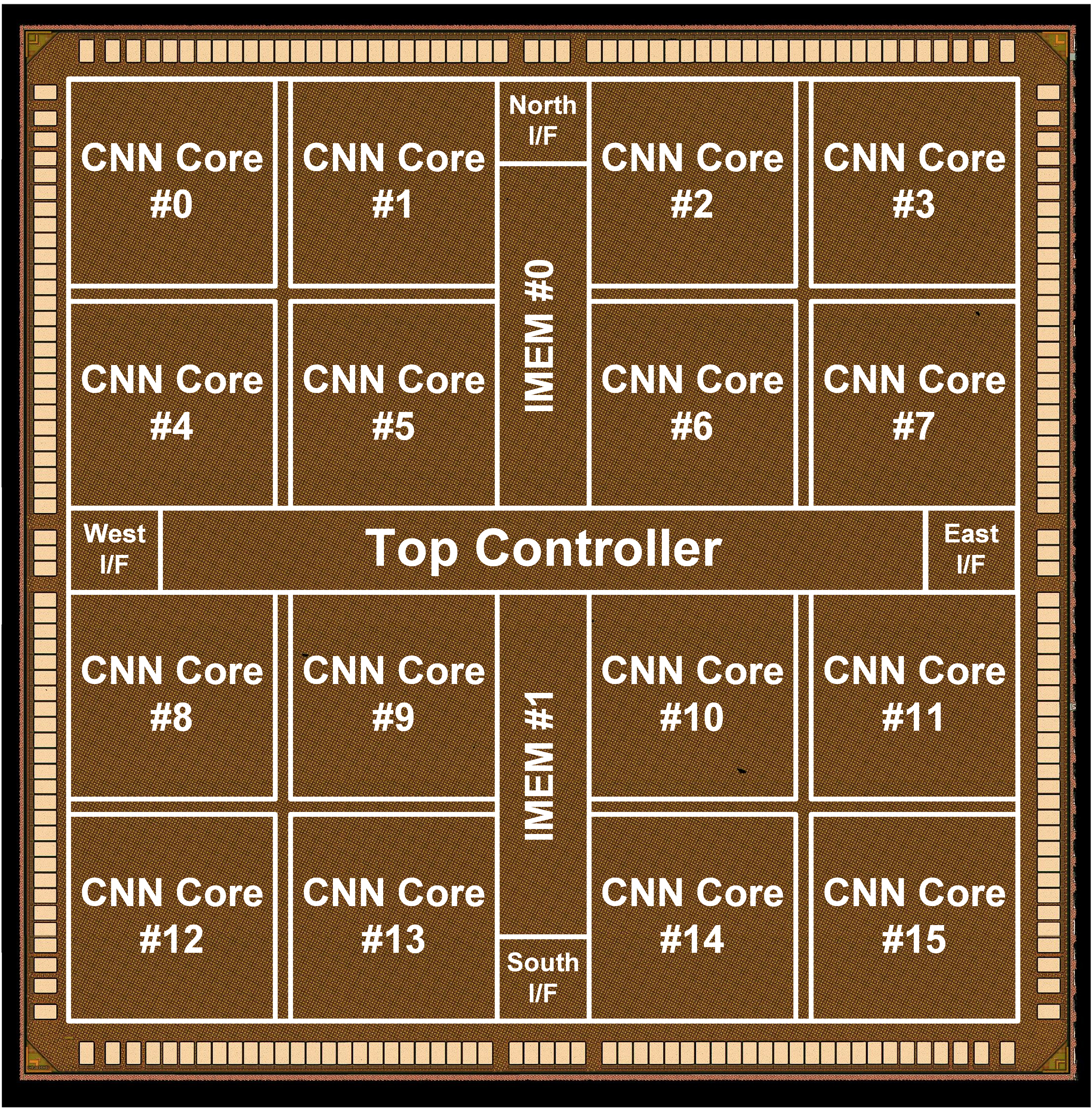
Title: NAND-Net: Minimizing Computational Complexity of In-Memory Processing for Binary Neural Networks
Authors: Hyeon-Uk Kim, Jae-Hyeong Sim, Yeong-Jae Choi, Lee-Sup Kim
Popular deep learning technologies suffer from memory bottlenecks, which significantly degrade the energy-efficiency, especially in mobile environments. In-memory processing for binary neural networks (BNNs) has emerged as a promising solution to mitigate such bottlenecks, and various relevant works have been presented accordingly. However, their performances are severely limited by the overheads induced by the modification of the conventional memory architectures. To alleviate the performance degradation, we propose NAND-Net, an efficient architecture to minimize the computational complexity of in-memory processing for BNNs. Based on the observation that BNNs contain many redundancies, we decomposed each convolution into sub-convolutions and eliminated the unnecessary operations. In the remaining convolution, each binary multiplication (bitwise XNOR) is replaced by a bitwise NAND operation, which can be implemented without any bit cell modifications. This NAND operation further brings an opportunity to simplify the subsequent binary accumulations (popcounts). We reduced the operation cost of those popcounts by exploiting the data patterns of the NAND outputs. Compared to the prior state-of-the-art designs, NAND-Net achieves 1.04-2.4x speedup and 34-59% energy saving, thus making it a suitable solution to implement efficient in-memory processing for BNNs.
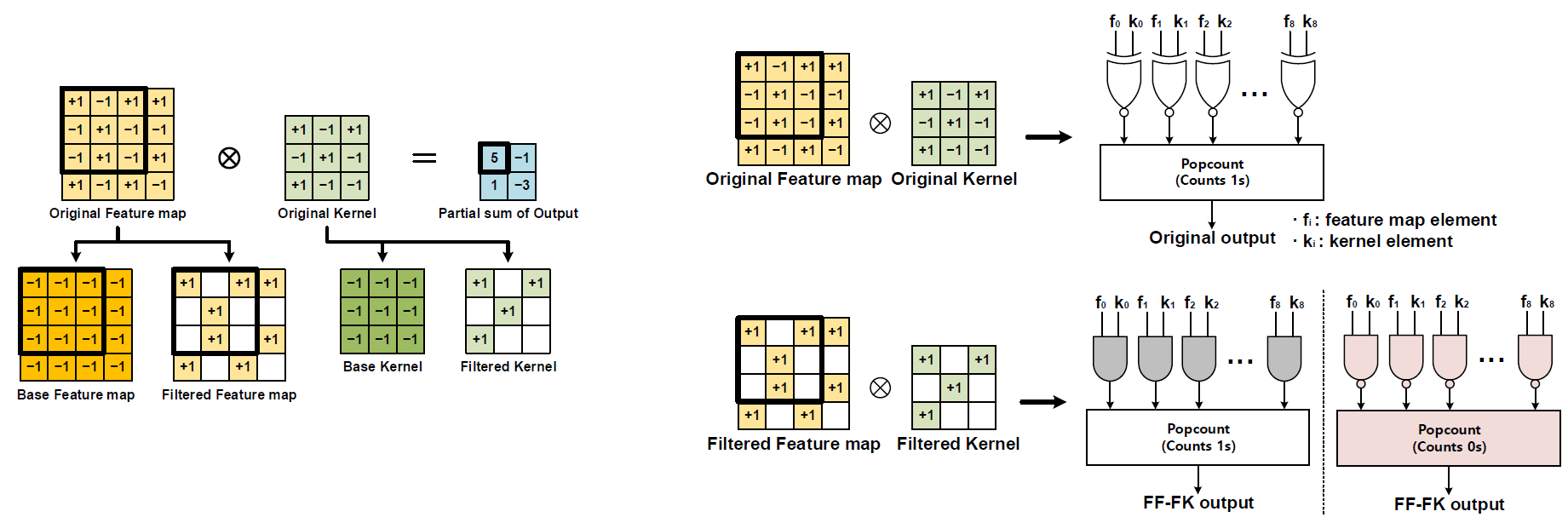