Authors: Tomer Raviv, Sangwoo Park, Nir Shlezinger, Osvaldo Simeone, Yonina C Eldar, Joonhyuk Kang
Conference: 2021 IEEE International Conference on Communications Workshops (ICC Workshops) – Emerging6G-Com
Abstract: While meta-learning reduces communication overhead via learning good inductive bias among related tasks, model-based learning solves this overhead issue by available communication-theory-based algorithms, e.g., Viterbi algorithm. We combine these two approaches, meta-learning and model-based learning, to further reduce communication overhead, namely Meta-ViterbiNet.
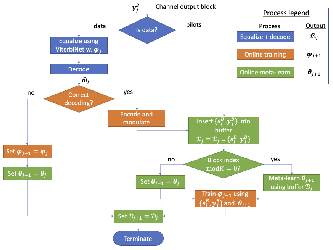
Fig. 5: Illustration of the operation of Meta-ViterbiNet