Abstract
Fairness and robustness are critical elements of Trustworthy AI that need to be addressed together. Fairness is about learning an unbiased model while robustness is about learning from corrupted data, and it is known that addressing only one of them may have an adverse affect on the other. In this work, we propose a sample selection-based algorithm for fair and robust training. To this end, we formulate a combinatorial optimization problem for the unbiased selection of samples in the presence of data corruption. Observing that solving this optimization problem is strongly NP-hard, we propose a greedy algorithm that is efficient and effective in practice. Experiments show that our algorithm obtains fairness and robustness that are better than or comparable to the state-of-the-art technique, both on synthetic and benchmark real datasets. Moreover, unlike other fair and robust training baselines, our algorithm can be used by only modifying the sampling step in batch selection without changing the training algorithm or leveraging additional clean data.
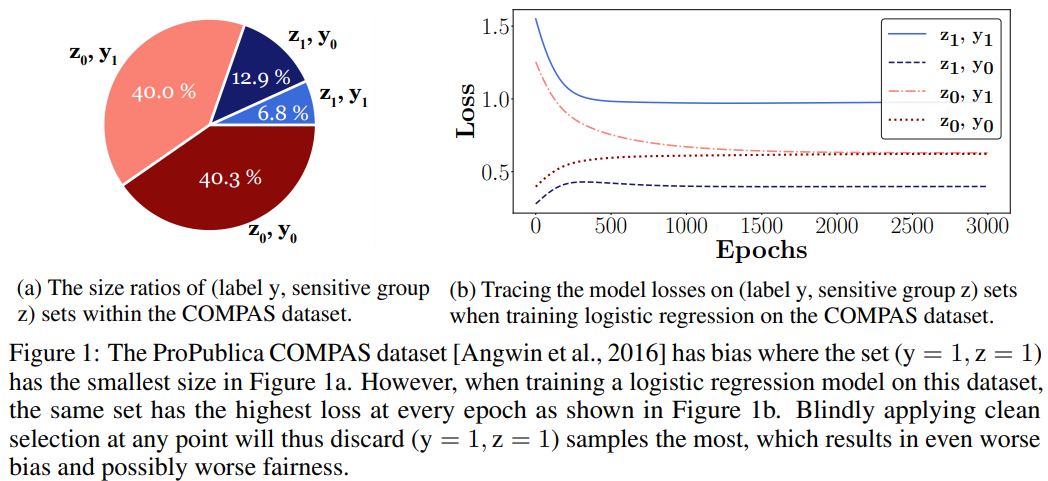
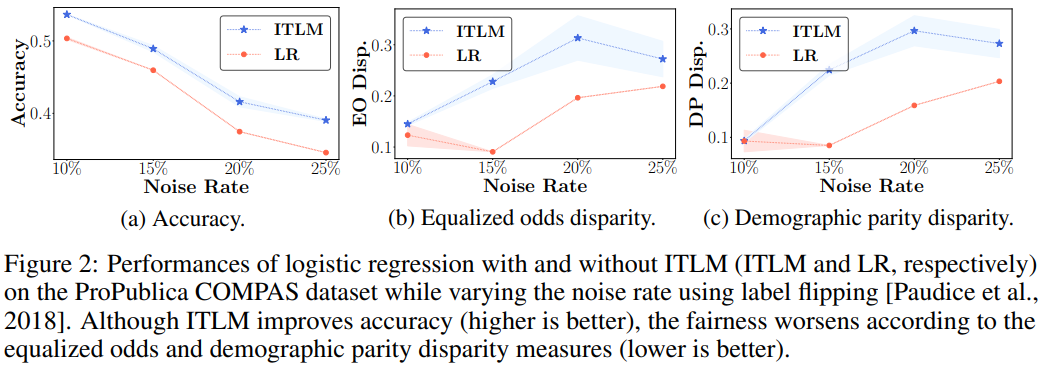