Conference/Journal, Year: CVPR, 2020
In this paper, we propose a novel and efficient reference feature extraction module referred to as the Similarity Search and Extraction Network (SSEN) for referencebased super-resolution (RefSR) tasks. The proposed module extracts aligned relevant features from a reference image to increase the performance over single image superresolution (SISR) methods. In contrast to conventional algorithms which utilize brute-force searches or optical flow estimations, the proposed algorithm is end-to-end trainable without any additional supervision or heavy computation, predicting the best match with a single network forward operation. Moreover, the proposed module is aware of not only the best matching position but also the relevancy of the best match. This makes our algorithm substantially robust when irrelevant reference images are given, overcoming the major cause of the performance degradation when using existing RefSR methods. Furthermore, our module can be utilized for self-similarity SR if no reference image is available. Experimental results demonstrate the superior performance of the proposed algorithm compared to previous works both quantitatively and qualitatively.
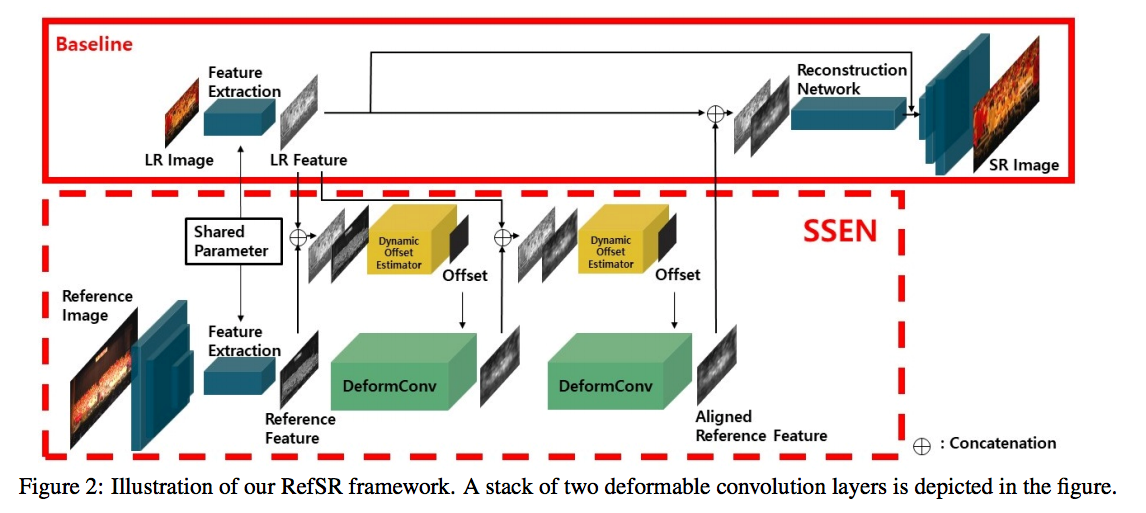