This paper proposes Weakly-supervised Moment Retrieval Network (WMRN) for Video Corpus Moment Retrieval (VCMR), which retrieves pertinent temporal moments related to natural language query in a large video corpus. Previous methods for VCMR require full supervision of temporal boundary information for training, which involves a labor-intensive process of annotating the boundaries in a large number of videos. To leverage this, the proposed WMRN performs VCMR in a weakly-supervised manner, where WMRN is learned without ground-truth labels but only with video and text queries. For weakly-supervised VCMR, WMRN addresses the following two limitations of prior methods: (1) Blurry attention over video features due to redundant video candidate proposals generation, (2) Insufficient learning due to weak supervision only with video-query pairs. To this end, WMRN is based on (1) Text Guided Proposal Generation (TGPG) that effectively generates text guided multi-scale video proposals in the prospective region related to query, and (2) Hard Negative Proposal Sampling (HNPS) that enhances video-language alignment via extracting negative video proposals in positive video sample for contrastive learning. Experimental results show that WMRN achieves state-of-the-art performance on TVR and DiDeMo benchmarks in the weakly-supervised setting. To validate the attainments of proposed components of WMRN, comprehensive ablation studies and qualitative analysis are conducted.
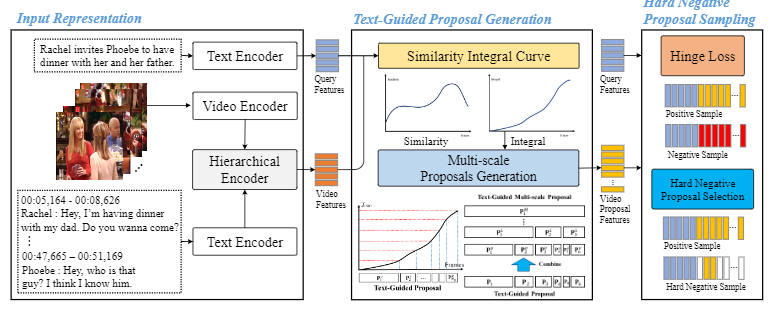
Figure 10. Weakly-supervised Moment Retrieval Network (WMRN) composed of two main modules: Text Guided Proposal Generation (TGPG) and Hard Negative Proposal Sampling (HNPS). The WMRN is designed based on following two insights: (1) Effectively generate multi-scale proposals pertinent to given query reduces the redundant computations and enhances attention performance and, (2) extract hard negative samples in contrastive learning to contribute to high-level interpretability. TGPG increases the number of candidate proposals in proximity of moments related to query, while decreases proposals in unrelated moments. HNPS selects negative samples in positive videos, which contribute to enhanced contrastive learning