Professor Yoon Young-Gyu’s research team develops AI imageing analysis technology ”SUPPORT” which enables high-precision measurement of biological fluorescence signals
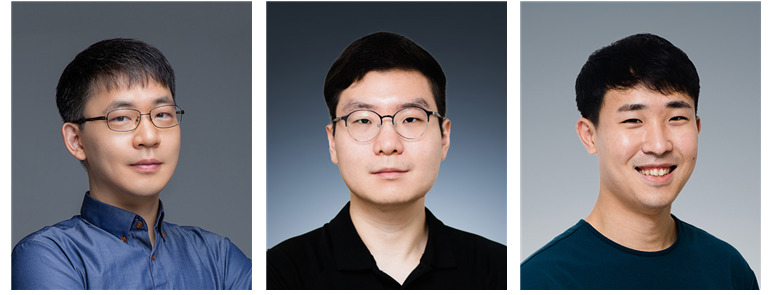
< (From the left) Professor Young-Gyu Yoon from the School of Electrical Engineering, Ph.D. student Minho Eom, and Ph.D. student Seungjae Han.>
KAIST (President Kwang-Hyung Lee) announced on the 19th that a research team led by Professor Young-Gyu Yoon from the School of Electrical Engineering has developed an AI imaging analysis technology that can measure biological fluorescence signals with over 10 times the precision of existing technologies.
With the recent advancement of genetic engineering technology, it has become possible to convert various biological signals, such as specific ion concentrations or voltages within living biological tissues, into fluorescence signals. Technologies that utilize fluorescence microscopy to capture time-lapse images of biological tissues and rapidly measure these signals have been developed and are in use.
However, because the fluorescence signals emitted from biological tissues are weak, measuring rapidly changing signals results in a very low signal-to-noise ratio, making precise measurements difficult. In particular, the accuracy of measurements becomes extremely low when measuring signals that change on a millisecond scale, such as the action potentials of neurons.
In response to this technical challenge, Professor Yoon’s research team developed an AI image analysis technology that enables measurements with over 10 times the precision of existing technologies.
This technology can autonomously learn the statistical distribution of data from fluorescence microscope images with a low signal-to-noise ratio and improve the signal-to-noise ratio of the images by more than tenfold even without the use of training data.
Utilizing this method, the measurement precision of various biological signals can be significantly enhanced. It is anticipated that this technology will be broadly applicable in the overall field of biological sciences and in the development of treatments for brain disorders.
Professor Yoon stated, “We named this technology SUPPORT (Statistically Unbiased Prediction utilizing sPatiOtempoRal information in imaging daTa) in the hope that it will support various neuroscience and biological science research.”
He added, “This is a technology that researchers using various fluorescence imaging devices can easily utilize without the need for separate training data. It has the potential to be broadly applied in uncovering new biological phenomena.”
Co-first author Minho Eom stated, “Through SUPPORT, we succeeded in precisely measuring rapid changes in biological signals that were difficult to observe. In particular, it’s now possible to optically measure the action potentials of neurons that change on a millisecond scale, which will be very useful for neuroscience research.” Co-first author Seungjae Han added, “While SUPPORT was developed for precise measurements of biological signals within fluorescence microscopy images, it can also be widely used to enhance the quality of general time-lapse images.”
This technology was developed under the supervision of Professor Young-Gyu Yoon’s team from the School of Electrical Engineering at KAIST, in multidisciplinary and multinational collaboration with researchers from the Department of Materials Science Engineering at KAIST (Professor Jae-Byum Chang), the Graduate School of Medical Science and Engineering at KAIST (Professor Pilhan Kim), Chungnam National University, Seoul National University, Harvard University, Boston University, the Allen Institute, and Westlake University.
This research was conducted with the support of the National Research Foundation of Korea and was published online in the international journal “Nature Methods” on September 19th. It was also selected as the cover article for the October issue.
1. Fluorescence signal: The brightness of light (fluorescence) changes in proportion to specific biological signal variations.
2. Timelapse: A video that continuously captures the subject at regular intervals.
Figure 1. Concept of SUPPORT technology:
(a) For each pixel in the image, the artificial neural network removes noise without separate training data by utilizing the surrounding pixel information within the current frame and information from adjacent frames.
(b) Impulse response of the designed artificial neural network.

Figure 2. Ultra-precise neural cell voltage measurement using SUPPORT:
(Top) In the original fluorescence image, it’s impossible to observe the action potentials of neurons due to the low signal-to-noise ratio.
(Bottom) By enhancing the signal-to-noise ratio using SUPPORT, it is possible to precisely observe the action potentials of each neural cell.
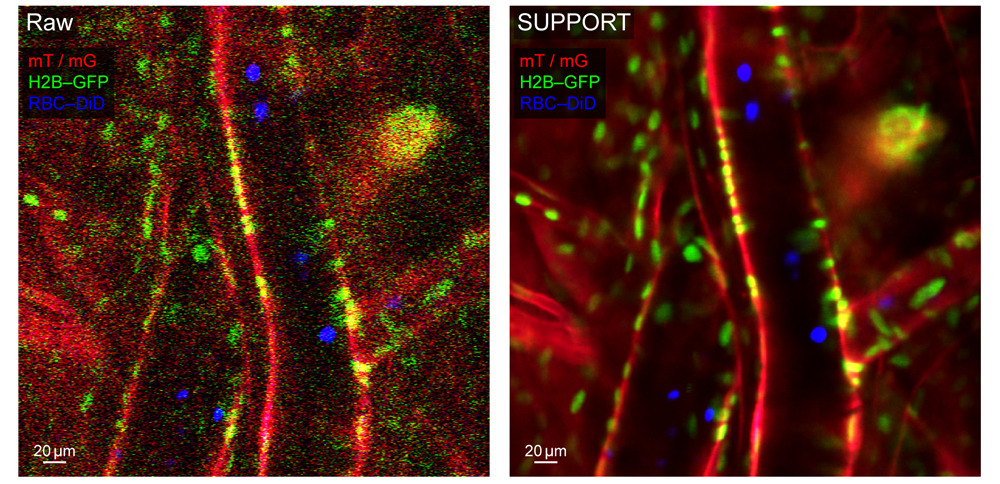
Figure 3. Improvement of in vivo ear tissue fluorescence images of mice using SUPPORT:
(Left) In the original fluorescence image, it’s impossible to observe the detailed structure of the tissue due to the low signal-to-noise ratio.
(Right) By enhancing the signal-to-noise ratio using SUPPORT, it is possible to observe the detailed structure and rapidly moving red blood cells.
Figure 4. Improvement of in vivo muscle tissue fluorescence images of mice using SUPPORT:
(Left) In the original fluorescence image, it’s impossible to observe the detailed structure of the tissue due to the low signal-to-noise ratio.
(Right) By enhancing the signal-to-noise ratio using SUPPORT, it is possible to observe the detailed structure of muscle fibers and rapidly moving red blood cells.