Title: Weak Detection of Signal in the Spiked Wigner Model
Authors: Hye Won Chung, Ji Oon Lee
We consider the problem of detecting the presence of the signal in a rank-one signal-plus-noise data matrix. In case the signal-to-noise ratio is under the threshold below which a reliable detection is impossible, we propose a hypothesis test based on the linear spectral statistics of the data matrix. When the noise is Gaussian, the error of the proposed test is optimal as it matches the error of the likelihood ratio test that minimizes the sum of the Type-I and Type-II errors. The test is data-driven and does not depend on the distribution of the signal or the noise. If the density of the noise is known, it can be further improved by an entrywise transformation to lower the error of the test.
One of the fundamental questions in machine learning is to detect signals from given data. If the data is of ‘signal-plus-noise’ type, the model is often referred to as a ‘spiked model.’ If the strength of the signal is considerably stronger than that of the noise, we can reliably detect the signal and also recover the signal from the noisy data. On the other hand, if the noise dominates the signal, it is impossible to detect the presence of the signal from the data, which is indistinguishable from pure noise.
In this paper, we consider the case that the strengths of the signal and of the noise are comparable. It was known that there is a certain threshold for the signal-to-noise ratio (SNR) above which the reliable detection, or the strong detection is available, whereas the strong detection is impossible if SNR is below the threshold. In the latter case, we try the weak detection to determine whether the signal is present in the given data. More precisely, we propose a hypothesis test with low computational complexity whose probability of error is minimal. The test is based on state-of-the-art techniques from random matrix theory.
If the noise is non-Gaussian, the test can be further improved by suitably processing the given data. Such a procedure, which we call an entrywise transformation in our work, effectively increases SNR. In case the noise has exponential decay, the entrywise transformation corresponds to applying a function similar to the hyperbolic tangent function (tanh) to each data entry. Our test is expected to be used in various problems with noisy high dimensional data such as community detection and angular synchronization.
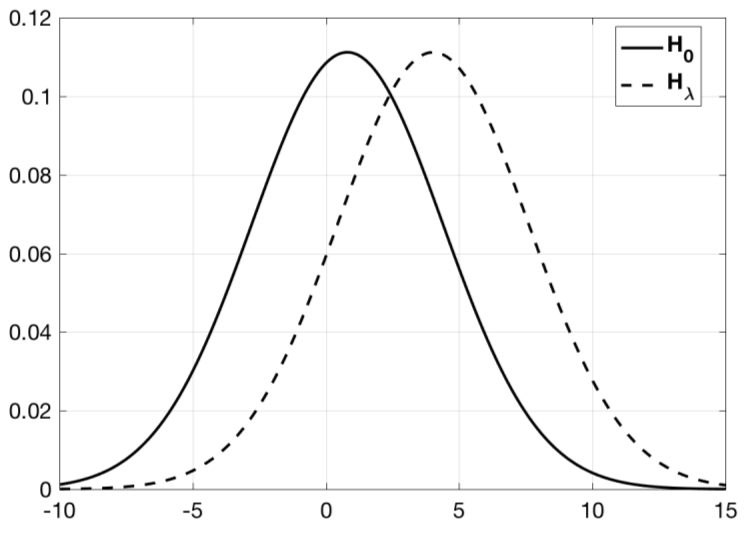
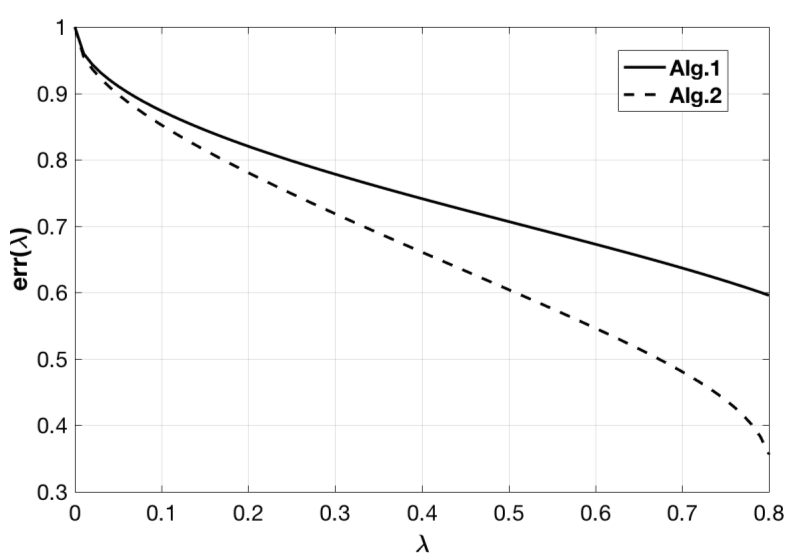