Title: Wireless, AI-enabled wearable thermal comfort sensor for energy-efficient, human-in-the-loop control of indoor temperature
Abstract: The conventional heating, ventilation, and air conditioning (HVAC) systems are based on a set-point control approach that only considers the temperature of the environment without reflecting the thermophysiological status of the occupant. This approach not only fails to fully satisfy individual thermal preferences, but it also makes an HVAC operation energy-inefficient. One possible solution is to control the indoor thermal condition based on an accurate prediction of the occupant’s thermal comfort to prevent any unnecessary energy consumption. Here, we present an artificial intelligence (AI) wearable sensor-based human-in-the-loop HVAC control system that is operated on a real-time basis reflecting the thermophysiological condition of the occupant to automatically improve their thermal comfort while reducing the energy consumption of the building. The wristband-type, AI-based, three-point wearable temperature sensor offers excellent thermal comfort prediction accuracy (93.9%), enabling a human-centric HVAC control operation. A proof-of-concept demonstration of closed human-in-the-loop HVAC control using the AI-enabled wearable sensor system confirms both the accuracy of the thermal comfort prediction and the energy-efficiency of this approach, demonstrating its potential as a new solution that improves the occupant’s thermal comfort and provides building energy savings.
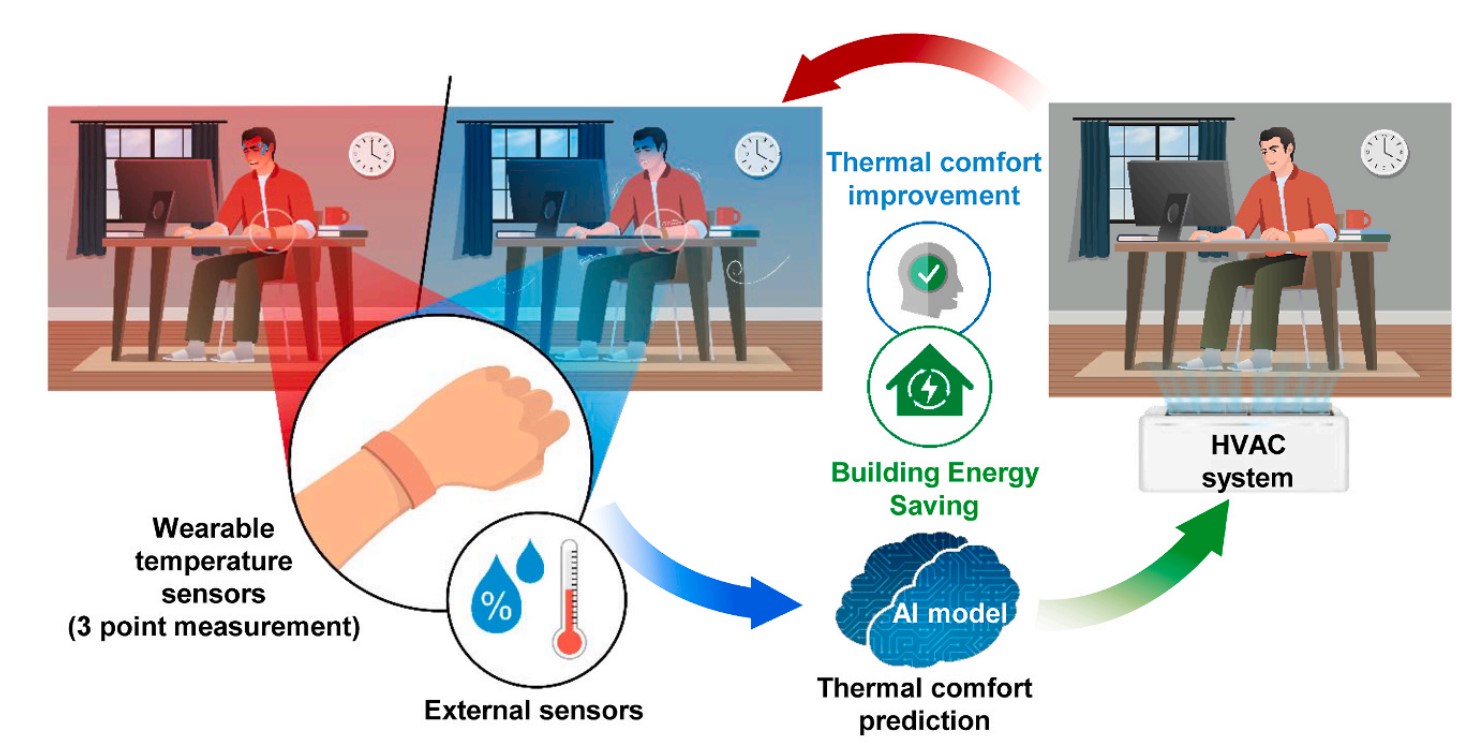
Title: 3D Neuromorphic Hardware with Single Thin-Film Transistor Synapses Over Single Thin-Body Transistor Neurons by Monolithic Vertical Integration
Abstract: Neuromorphic hardware with a spiking neural network (SNN) can significantly enhance the energy efficiency for artificial intelligence (AI) functions owing to its event-driven and spatiotemporally sparse operations. However, an artificial neuron and synapse based on complex complementary metal-oxide-semiconductor (CMOS) circuits limit the scalability and energy efficiency of neuromorphic hardware. In this work, a neuromorphic module is demonstrated composed of synapses over neurons realized by monolithic vertical integration. The synapse at top is a single thin-film transistor (1TFT-synapse) made of poly-crystalline silicon film and the neuron at bottom is another single transistor (1T-neuron) made of single-crystalline silicon. Excimer laser annealing (ELA) is applied to activate dopants for the 1TFT-synapse at the top and rapid thermal annealing (RTA) is applied to do so for the 1T-neuron at the bottom. Internal electro-thermal annealing (ETA) via the generation of Joule heat is also used to enhance the endurance of the 1TFT-synapse without transferring heat to the 1T-neuron at the bottom. As neuromorphic vision sensing, classification of American Sign Language (ASL) is conducted with the fabricated neuromorphic module. Its classification accuracy on ASL is ≈92.3% even after 204 800 update pulses.
Main Figure:
Title: An artificial olfactory sensory neuron for selective gas detection with in-sensor computing
Abstract: We present a neuromorphic sensory module for gas detection using a two-in-one typed olfactory neuron for in-sensor computing. The module integrates a sensor for gas detection and a neuron for generating spike signals and delivering them into the post-synapse. The sensing ability is enabled by catalytic metal particles on a silicon nanowire field-effect transistor (Si-NW FET), while the neuronal ability is also realized by the Si-NW FET itself, which encodes spike signals for a spiking neural network (SNN). By mounting palladium (Pd) and platinum (Pt) nanoparticles on the Si-NW FET, we demonstrate the module to classify H2 and NH3 using a single-layer perceptron (SLP) with the sensory neurons and FET-based synapses. Power demand and manufacturing cost efficiency are important considerations in mobile applications and edge computing in the Internet-of-Things era. This in-sensor module-based SNN hardware provides a cost-effective solution that is inherently more power and form-factor efficient over existing designs.
Main Figure:
Title: Self-aware artificial auditory neuron with a triboelectric sensor for spike-based neuromorphic hardware
Abstract: Auditory organs can detect and process sounds with the help of the organ of Corti located in the cochlea. The energy efficiency of biological processing for an input sound signal is very high due to the spike-based auditory neurons in auditory organs. Here, inspired by the biological auditory system, a self-aware artificial auditory neuron module is constructed by serially connecting a triboelectric nanogenerator (TENG) and a bi-stable resistor (biristor). The TENG serves as a sound sensor and a current source to feed current input so as to awaken the biristor, which acts as a device-level neuron that is dissimilar to a traditional circuit-based neuron. The proposed self-aware artificial neuron module simultaneously detects the sound pressure level (SPL) and encodes it into a spike form, after which it transmits these data to an artificial synapse as an input neuron for a spiking neural network (SNN). Like an auditory organ, the spiking frequency of the bio-inspired artificial neuron increases when the SPL increases. In addition, the self-aware artificial auditory system with a single-layer perceptron (SLP) for SNN is demonstrated for musical pitch classification. This artificial auditory system is configured by combining two artificial auditory neuron modules and four metal-oxide-semiconductor field-effect transistor (MOSFET) synapses. The biristor neuron and the MOSFET synapse are structurally identical but electrically different. Two artificial auditory neuron modules correspond to two different frequencies. This artificial auditory system distinguishes two sounds from a piano. It also identifies two similar sounds from a cello and a violin. The proposed artificial SNN-based auditory system is advantageous for low power consumption due to the event-driven spiking transmission scheme. The improved energy efficiency with the SNN as described here is attributed to the self-aware sensing capability for sound signals. Therefore, the self-aware SNN auditory system given its low power consumption is promising for a remote sensor, a wireless sensor, and for an Internet of Things (IoT) sensor system.
Main Figure:
Title: Artificial Multisensory Neuron with a Single Transistor for Multimodal Perception through Hybrid Visual and Thermal Sensing
Abstract: An artificial multisensory device applicable to in-sensor computing is demonstrated with a single-transistor neuron (1T-neuron) for multimodal perception. It simultaneously receives two sensing signals from visual and thermal stimuli. The 1T-neuron transforms these signals into electrical signals in the form of spiking and then fires them for a spiking neural network at the same time. This feature makes it feasible to realize input neurons for multimodal sensing. Visual and thermal sensing is achieved due to the inherent optical and thermal behaviors of the 1T-neuron. To demonstrate a neuromorphic multimodal sensing system with the artificial multisensory 1T-neuron, fingerprint recognition, widely used for biometric security, is implemented. Owing to the simultaneous sensing of heat as well as light, the proposed fingerprint recognition system composed of multisensory 1T-neurons not only identifies a genuine pattern but also judges whether or not it is forged.
Main Figure:
Title: Triple-Node FinFET With Non-Ohmic Schottky Junctions for Synaptic Devices
Abstract: A triple-node FinFET (TriNo-FinFET) with non-ohmic Schottky junctions is demonstrated for an artificial synapse. The three mechanisms of thermionic emission in a subthreshold region, tunneling in a transition region, and drift transport in an inversion region are utilized in the TriNo-FinFET with non-ohmic Schottky junctions. The transition region dominated by tunneling with non-ohmic Schottky junctions improves the linearity of potentiation and depression. An average recognition rate of 90 % for handwritten digits in the MNIST dataset is achieved. Moreover, the TriNo-FinFET with the double-layered charge trap layer (CTL) shows enhanced weight-update speed by up to 48-fold compared to that with a single-layered CTL.
Main Figure:
Journal: Advanced Intelligent Systems
Abstract: Internet-of-things (IoT) edge devices with a memristive neuromorphic system can more effectively enhance daily lives. However, cyberattacks remain critical concerns for smart IoT edge devices that process a vast body of information via networks. Herein, a highly secure neuromorphic system is reported, which can be implemented using a physically unclonable function (PUF) that exploits the high entropy achieved via the stochastic switching of a poly(1,3,5-trivinyl-1,3,5-trimethyl cyclotrisiloxane) (pV3D3)-based memristor. The excellent insulating property of pV3D3 enhances the stochasticity of the tunneling distance for randomly ruptured Cu filaments. The pV3D3 memristor-based PUF (pV3D3-PUF) achieves near-ideal 50% averages for uniformity and uniqueness, excellent reliability under conditions of mechanical stress and water immersion, and reconfigurability-bolstering security without additional hardware. Using stochastic in-memory computing, the pV3D3-PUF shows resilience to machine learning attacks. Furthermore, a cryptography protocol is demonstrated, which enables artificial intelligence service implementation without security issues for PUF-integrated pV3D3 memristor-based neuromorphic systems.
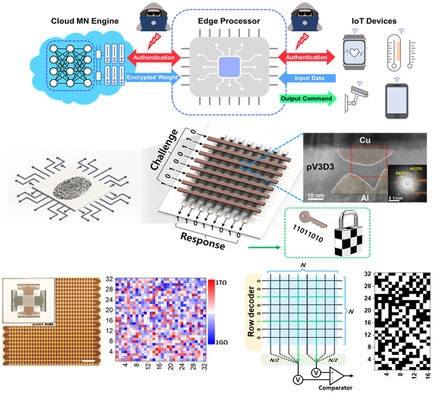
Journal: Advanced Intelligent Systems
Abstract: As the use of artificial intelligence (AI) soars, the development of novel neuromorphic computing is demanding because of the disadvantages of the von Neumann architecture. Furthermore, extensive research on electrochemical metallization (ECM) memristors as synaptic cells have been carried out toward a linear conductance update for online learning applications. In most cases, however, a conductance distribution change over time has not been studied as a major issue, giving less consideration to inference-only computing accelerators based on offline learning. Herein, organic–inorganic bilayer stacking for synaptic unit cells using poly(1,3,5-trivinyl-1,3,5-trimethyl cyclotrisiloxane) (pV3D3) and Al2O3 thin films is suggested, showing highly enhanced reliability for offline learning. The bilayer structure achieves better reliability and control of the analog resistive switching and synaptic functions, respectively, through the guided formation of conductive filaments via tip-enhanced electric fields. In addition, 5-bit multilevel states achieve long-term stability (>104 s) following an in-depth study on conductance-level stability. Finally, a device-to-system-level simulation is performed by building a convolutional neural network (CNN) based on the hybrid devices. This highlighted the significance of multilevel states in fully connected layers. It is believed that the study provides a practical approach to using ECM-based memristors for inference-only neural network accelerators.
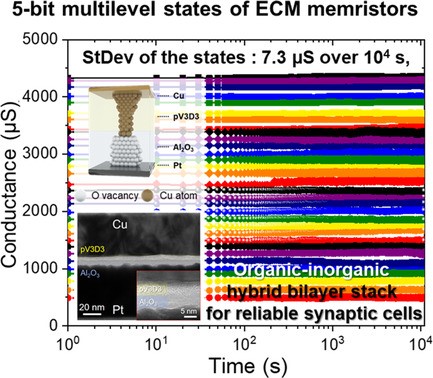
Abstract
A neuromorphic module of an electronic nose (E-nose) is demonstrated by hybridizing a chemoresistive gas sensor made of a semiconductor metal oxide (SMO) and a single transistor neuron (1T-neuron) made of a metal-oxide-semiconductor field-effect transistor (MOSFET). By mimicking a biological olfactory neuron, it simultaneously detects a gas and encoded spike signals for in-sensor neuromorphic functioning. It identifies an odor source by analyzing the complicated mixed signals using a spiking neural network (SNN). The proposed E-nose does not require conversion circuits, which are essential for processing the sensory signals between the sensor array and processors in the conventional bulky E-nose. In addition, they do not have to include a central processing unit (CPU) and memory, which are required for von Neumann computing. The spike transmission of the biological olfactory system, which is known to be the main factor for reducing power consumption, is realized with the SNN for power savings compared to the conventional E-nose with a deep neural network (DNN). Therefore, the proposed neuromorphic E-nose is promising for application to Internet of Things (IoT), which demands a highly scalable and energy-efficient system. As a practical example, it is employed as an electronic sommelier by classifying different types of wines.
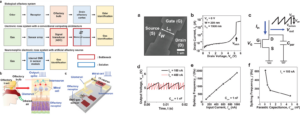
Abstract
An artificial synapse is an essential element to construct a hardware-based artificial neural network (ANN). While various synaptic devices have been proposed along with studies on electrical characteristics and proper applications, a small number of conductance states with nonlinear and asymmetric conductance changes have been problematic and imposed limits on computational performance. Their applications are thus still limited to the classification of simple images or acoustic datasets. Herein, a polymer electrolyte-gated synaptic transistor (pEGST) is demonstrated for video-based learning and inference using transfer learning. In particular, abnormal car detection (ACD) is attempted with video-based learning and inference to avoid traffic accidents. The pEGST showed multiple states of 8,192 (=13 bits) for weight modulation with linear and symmetric conductance changes and helped reduce the error rate to 3% to judge whether a car in a video is abnormal.
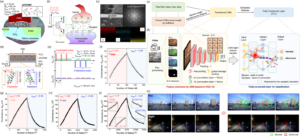