Journal Name: ACS Photonics (IF 7.529)
Authors : Dongjin Seo, Daniel Wontae Nam, Juho Park, Chan Y. Park, and Min Seok Jang*
Title: Structural Optimization of a One-Dimensional Freeform Metagrating Deflector via Deep Reinforcement Learning
Abstract: The increasing demand on a versatile high-performance metasurface requires a freeform design method that can handle a huge design space, which is many orders f agnitude larger than that of conventional fixed-shape optical structures. In this work, we formulate the designing process of one-dimensional freeform Si metasurface beam deflectors as a reinforcement learning problem to find their optimal structures consistently without requiring any prior metasurface data. During training, a deep Q-network-based agent stochastically explores the device design space around the learned trajectory optimized for deflection efficiency. The devices discovered by the agents show over all improvements in maximum efficiency compared to the ones that state of-the-art baseline methods find at various wavelengths and deflection angles. Furthermore, the efficiencies of the devices generated by agents trained from different neural network initializations have a small variance, demonstrating the robustness of the proposed design method.
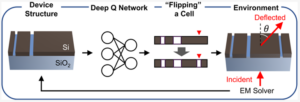
Journal Name: Review Article, Nanophotonics (IF 8.449)
Authors : Juho Park, Sanmun Kim, Daniel Wontae Nam, Haejun Chung*, Chan Y. Park* and Min Seok Jang*
Title: Free-form optimization of nanophotonic devices: from classical methods to deep learning
Abstract: Nanophotonic devices have enabled microscopic control of light with an unprecedented spatial resolution by employing subwavelength optical elements that can strongly interact with incident waves. However, to date, most nanophotonic devices have been designed based on fixed-shape optical elements, and a large portion of their design potential has remained unexplored. It is only recently that free-form design schemes have been spotlighted in nanophotonics, offering routes to make a break from conventional design constraints and utilize the full design potential. In this review, we systematically overview the nascent yet rapidly growing field of free-form nanophotonic device design. We attempt to define the term “free-form” in the context of photonic device design, and survey different strategies for free-form optimization of nanophotonic devices spanning from classical methods, adjoint-based methods, to contemporary machine-learning-based approaches.
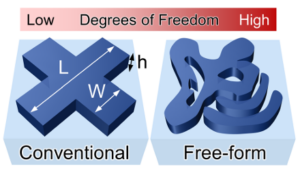
Journal Name: Nanophotonics (IF 8.449)
Authors : Sanmun Kim, Jeong Min Shin, Jaeho Lee, Chanhyung Park, Songju Lee, Juho Park, Dongjin Seo, Sehong Park, Chan Y. Park and Min Seok Jang*
Title: Inverse design of organic light-emitting diode structure based on deep neural networks
Abstract: The optical properties of thin-film light emitting diodes (LEDs) are strongly dependent on their structures due to light interference inside the devices. However, the complexity of the design space grows exponentially with the number of design parameters, making it challenging to optimize the optical properties of multilayer LEDs with rigorous electromagnetic simulations. In this work, we demonstrate an artificial neural network that can predict the light extraction efficiency of an organic LED structure in 30 ms, which is ∼103 times faster than the rigorous simulation in a single-treaded execution with root-mean squared error of 1.86×10−3. The effective inference time per structure is brought down to ∼0.6 μs with unaltered error rate with parallelization. We also show that our neural networks can efficiently solve the inverse problem finding a device design that exhibits the desired light extraction spectrum– within the similar time scale. We investigate the one-to-many mapping issue of the inverse problem and find that the degeneracy can be lifted by incorporating additional emission spectra at different observing angles. Furthermore, the forward neural network is combined with a conventional genetic algorithm to address additional large-scale optimization problems including maximization of light extraction efficiency and minimization of angle dependent color shift. Our approach establishes a platform for tackling computation-heavy optimization tasks with one-time computational cost.
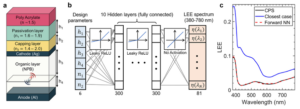
제목: Quantum amplitude-amplification operators
논문지, 연도: Physical Review A, 2021
저자: Hyeokjea Kwon and Joonwoo Bae
초록: In this work, we show the characterization of quantum iterations that would generally construct quantum amplitude-amplification algorithms with a quadratic speedup, namely, quantum amplitude-amplification operators (QAAOs). Exact quantum search algorithms that find a target with certainty and with a quadratic speedup can be composed of sequential applications of QAAOs: existing quantum amplitude-amplification algorithms thus turn out to be sequences of QAAOs. We show that an optimal and exact quantum amplitude-amplification algorithm corresponds to the Grover algorithm together with a single iteration of QAAO. We then realize three-qubit QAAOs with current quantum technologies via cloud-based quantum computing services, IBMQ and IonQ. Finally, our results show that the fixed-point quantum search algorithms known so far are not a sequence of QAAOs; for example, the amplitude of a target state may decrease during quantum iterations.
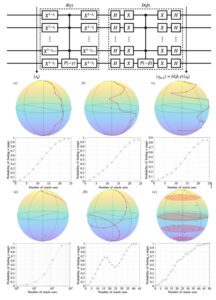
Title : Deep Reinforcement Learning-based Interconnection Design for 3D X-Point Array Structure Considering Signal Integrity
Abstract : In this paper, we, for the first time, proposed the Reinforcement Learning (RL) based interconnection design for 3D X-Point array structure considering crosstalk and IR drop. We applied the Markov Decision Process (MDP) to correspond to finding the optimal interconnection design problem to RL problem. We defined interconnection state to the vector, design to the action and the number of bits, crosstalk and IR drop are considered as the reward. The Proximal Policy Optimization (PPO) and Long Short-Term Memory (LSTM) are used to RL algorithms. The proposed interconnection design model is well trained and shows convergence of reward score in 16×16, 32×32 and 64×64 cases. We verified that the trained model finds out optimal interconnection design considering both memory size and signal integrity issues
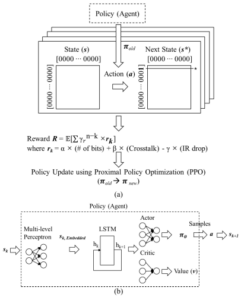
Title : Reinforcement-Learning-Based Signal Integrity Optimization and Analysis of a Scalable 3-D X-Point Array Structure
Abstract : In this article, we, for the first time, propose a reinforcement learning (RL) model to design an optimal 3-D cross-point (X-Point) array structure considering signal integrity issues. The interconnection design problem is modeled to the Markov decision process (MDP). The proposed RL model designs the 3-D X-Point array structure based on three reward factors: the number of bits, the crosstalk, and the IR drop. We applied multilayer perceptron (MLP) and long short-term memory (LSTM) to parameterize the policy. Proximal policy optimization (PPO) is used to optimize the parameters to train the policy. The reward of the proposed RL model is well-converged with variations in the array structure size and hyperparameters of the reward factors. We verified the scalability and sensitivity of the proposed RL model. With the optimal 3-D X-Point array structure design, we analyzed the reward factor and signal integrity issues. The optimal design of the 3-D X-Point array structure shows 17%–26.5% better signal integrity performance than the conventional design in finer process technology. In addition, we suggest a range of possible directions for improvement of the proposed model with variations in MDP tuples, reward factors, and learning algorithms, among other factors. Using the proposed model, we can easily design an optimal 3-D X-Point array structure with a certain size, performance capabilities, and specifications based on reward factors and hyperparameters.
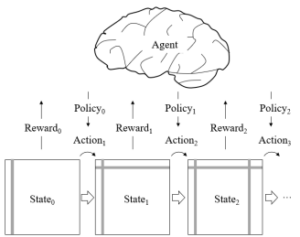
Conference : DesignCon 2022
Title : Learning Super-scale Microbump Pin Assignment Optimization for Real-world PCB Design with Graph Representation
Authors: Joonsang Park, Minsu Kim, Seonguk Choi, Jihun Kim, Haeyeon Kim, Hyunwook Park, Seongguk Kim, Taein Shin, Joungho Kim
Abstract: The requirement for higher bandwidth in computing systems has increased. Hence, the number of I/Os of 2.5D/3D ICs is also increasing for dense interconnections. Accordingly, the pin count of the microbump package is getting larger along with its signal integrity issues. In this paper, we propose a deep reinforcement learning (DRL)-based pin assignment optimization method that represents microbumps on graphs to minimize signal integrity degradation. The pin assignment task of microbumps is formulated by modifying the maximum independent set (MIS) problem which is a graph combinatorial optimization task. The proposed method is designed by making adjustments to a state-of-the-art DRL-based MIS solver. The graph-based learning method brings advantages in that it can assign pins to pin maps of any shape on a very large scale. We verify that the proposed DRL-based method is effective by comparing it with a meta-heuristic method, a conventional method for solving optimization tasks, called genetic algorithm.
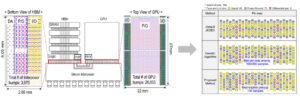
Conference : EPEPS 2021
Title: Deep Reinforcement learning-based Pin Assignment Optimization of BGA Packages considering Signal Integrity with Graph Representation
Authors: Joonsang Park, Minsu Kim, Seongguk Kim, Keeyoung Son, Taein Shin, Hyunwook Park, Jihun Kim, Seonguk Choi, Haeyeon Kim, Keunwoo Kim, and Joungho Kim
Abstract: In this paper, we propose a novel deep reinforcement learning (DRL)-based pin assignment method by representing ball grid array (BGA) packages on graphs to minimize signal integrity issues. The proposed method represents the pin arrangement of BGAs in graphs to formulate the pin assignment task to a variant of the maximum independent set (MIS). Then, a state-of-the-art DRL-based MIS solver was introduced to solve our task. Unlike previous methods of BGA optimization, the proposed graph representation of pins makes it possible to assign pins of any shape. Moreover, the significant scaling performance enables us to handle BGA with high pin count. We verify that the proposed DRL-based method with graph representation is effective by comparing it with conventional meta-heuristic methods including genetic algorithm (GA).
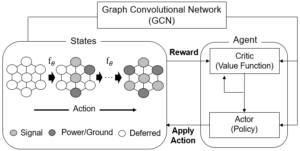
Imitation Learning with Bayesian Exploration (IL-BE) for Signal Integrity (SI) of PAM-4 based High-speed Serial Link: PCIe 6.0 (DesignCon 2022)
Authors: Jihun Kim, Minsu Kim, Hyunwook Park, Jiwon Yoon, Seonguk Choi, Joonsang Park, Haeyeon Kim, Keeyoung Son, Seongguk Kim, Daehwan Lho, Keunwoo Kim, Jinwook Song, Kyungsuk Kim, Jongkyu Park and Joungho Kim
Abstract: This paper proposes a novel imitation learning with Bayesian exploration (IL-BE) method to optimize via parameters of any given channel parameters for signal integrity (SI) of PAM-4 based high-speed serial link on PCIe 6.0. PCIe 6.0. is a crucial interconnect link for highspeed communication of processors, and PAM-4 signaling is a major component of PCIe 6.0 that can double bandwidth. However, the design space of PAM-4 based PCIe 6.0 is extremely complex. Moreover, because PAM-4 signaling reduces eye-margin 1/3 compared with NRZ signaling, it is more sensitive to optimize. Bayesian optimization (BO) is a candidate method because it shows powerful searching abilities on black-box continuous optimization space. However, BO has a significant limitation to apply via optimization for any given channel parameters because BO needs massive iterations to solve each problem (i.e., no adaptation to new tasks). Deep reinforcement learning is a promising method that deep neural network (DNN) agents learn to capture meta-features among problems interacting with the real-world environment. Therefore, learned DNN agents can adapt to a new problem by optimization with small iterations. However, DNN agents must learn through massive iterative trial and error; it is extremely complex to train DNN. We blend the benefit of the BO and DRL method. Firstly, we collect high-quality expert data using BO rather than relying on poor exploration of the initial DNN agent. Then we use the collected high-quality data to train DNN agents by using an imitation learning scheme. For verification, we target one pair differential PCIe 6.0 (64Gbps) interconnection of SSD board and three-layer transition; a task is formulated to optimize via given channel parameters. The statistical simulation method is used to evaluate SI performances, including PAM-4 eye-diagram. Proposed IL-BE shows 100× faster training speed than conventional DRL method, 16× reduced iterations for the via parameter optimization than BO having state-of-the-art SI performances.
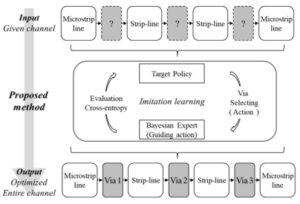
Title: PAM-4 based PCIe 6.0 Channel Design Optimization Method using Bayesian Optimization
(EPEPS 2021)
Authors: JihunKim, Hyunwook Park, Minsu Kim, Seonguk Choi, Keeyoung Son, Joonsang Park, Haeyeon Kim, Jinwook Song, Youngmin Ku, Jonggyu Park and Joungho Kim.
Abstract: This paper, for the first time, proposes a pulse amplitude modulation-4 (PAM-4) based peripheral component interconnect express (PCIe) 6.0 channel design optimization method using Bayesian Optimization (BO). The proposed method provides a sub-optimal channel design with PAM-4 signaling that maximizes target function considering signal integrity (SI). We formulate the target function of BO as a linear combination of the channel insertion loss (IL) and crosstalk (FEXT, NEXT) considering characteristics of PAM-4 signaling. To consider the trade-off between insertion loss and crosstalk in PAM-4 signaling, we obtain reasonable coefficients for formulating target function via ablation study. For verification, an eye diagram simulation with PAM-4 signaling is conducted. We compare the channel performance of the proposed method and random search method (RS). Also, the proposed method is compared with the only IL- considered BO method to verify the impact of crosstalk in PAM-4 signaling. As a result, the channel optimized by the proposed method only obtains eye height and eye width of PAM-4 eye diagram, and the PAM-4 eyes of other comparison methods are closed.
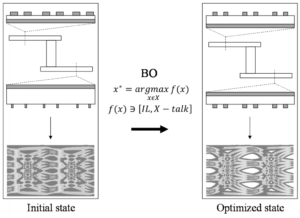