Professor Sung-Ju Lee’s research team develops a smarthphone AI system that diagnoses mental health based on user’s voice and text input
A research team led by Professor Sung-Ju Lee of the Department of Electrical and Electronic Engineering has developed an artificial intelligence (AI) technology that automatically analyzes users’ language usage patterns on smartphones without personal information leakage, thereby monitoring users’ mental health status.
This technology allows smartphones to analyze and diagnose a user’s mental health state simply by carrying and using the phone in everyday life.
The research team focused on the fact that clinical diagnosis of mental disorders is often done through language use analysis during patient consultations.
The new technology uses (1) keyboard input content such as text messages written by the user and (2) voice data collected in real-time from the smartphone’s microphone for mental health diagnosis.
This language data, which may contain sensitive user information, has previously been challenging to utilize.
The solution to this issue in this technology involves the application of federated learning AI, which trains the AI model without data leakage outside the user’s device, thus eliminating privacy invasion concerns.
The AI model is trained on datasets based on everyday conversation content and the speaker’s mental health. It analyzes the conversations into the smartphone in real-time and predicts the user’s mental health scale based on the learned content.
Furthermore, the research team developed a methodology to effectively diagnose mental health from the large amount of user language data provided on smartphones.
Recognizing that users’ language usage patterns vary in different real-life situations, they designed the AI model to focus on relatively important language data based on the current situation indicated by the smartphone.
For example, the AI model may prioritize analyzing conversations with family or friends in the evening over work-related discussions, as they may provide more clues for monitoring mental health.
This research was conducted in collaboration with Jaemin Shin(CS), HyungJun Yoon (EE Ph.d course) , Seungjoo Lee (EE master’s course), Sung-Joon Park, CEO of Softly AI (KAIST alumnus), Professor Yunxin Liu of Tsinghua University in China, and Professor Jin-Ho Choi of Emory University in the USA.
The paper, titled “FedTherapist: Mental Health Monitoring with User-Generated Linguistic Expressions on Smartphones via Federated Learning,” was presented at the EMNLP (Conference on Empirical Methods in Natural Language Processing), the most prestigious conference in the field of natural language processing, held in Singapore from December 6th to 10th.
Professor Sung-Ju Lee commented, “This research is significant as it is a collaboration of experts in mobile sensing, natural language processing, artificial intelligence, and psychology. It enables early diagnosis of mental health conditions through smartphone use without worries of personal information leakage or privacy invasion. We hope this research can be commercialized and benefit society.”
This research was funded by the government (Ministry of Science and ICT) and supported by the Institute for Information & Communications Technology Planning & Evaluation (No. 2022-0-00495, Development of Voice Phishing Detection and Prevention Technology in Mobile Terminals, No. 2022-0-00064, Development of Human Digital Twin Technology for Predicting and Managing Mental Health Risks of Emotional Laborers).
<picture 1. A smartphone displaying an app interface for mental health diagnosis. The app shows visualizations of user’s voice and keyboard input analysis, with federated learning technology>
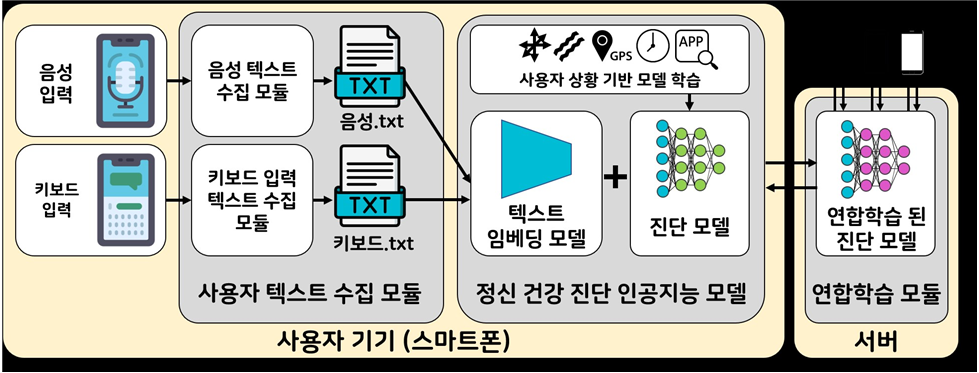
<picture 2. A schematic diagram of the mental health diagnosis technology using federated learning, based on user voice and keyboard input on a smartphone>