Dynamic IR drop analysis is very time consuming, so it is only applied in signoff stage before tapeout. U-net model, which is an image-to-image translation neural network, is employed for quick analysis of dynamic IR drop. A number of feature maps are used for u-net input: a map of effective PDN resistance seen from each gate, a map of current consumption of each gate (in particular time instance), and a map of relative distance to nearest power supply pad. A layout is partitioned into a grid of regions and IR drop is predicted region-by-region. For fast prediction, (1) analysis is performed only in time windows which are estimated to cause high IR drop, and (2) effective PDN resistance is approximated through a proposed simplification method. Experiments with a few test circuits demonstrate that dynamic IR drop is predicted 20 times faster than commercial analysis package with 15% error.
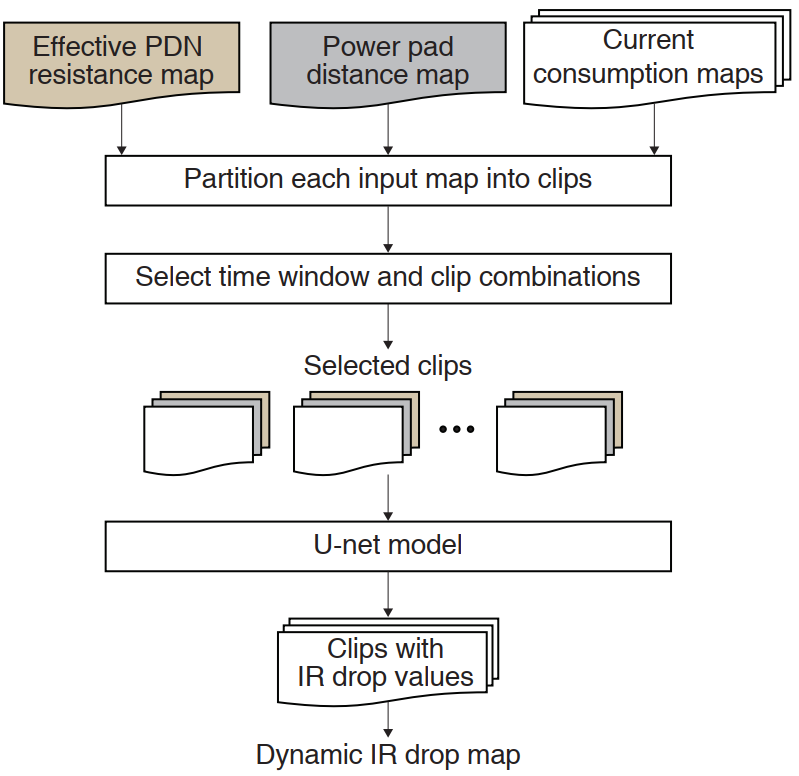