Accurate and fast lithography model is necessary for computational lithography applications such as optical proximity correction (OPC) and lithography rule check. In lithography model, optical model calculates image intensity followed by resist model that outputs a resist contour. Resist model is an empirical model, in which images are convolved with resist kernels and their weighted sum is used to derive a resist model signal that is compared with some threshold. Conventional resist model use a simple form of resist kernels such as Gaussian kernels, therefore it requires many kernels to achieve high accuracy. We propose to use free-form resist kernels. Resist model has the same structure as convolutional neural network (CNN), thus, we represent resist model with free-form kernels in CNN and train the network. To avoid overfitting of the proposed model, we initialize the model with conventional Gaussian kernels. Training data is carefully selected so that resist contour is accurately predicted. A conventional resist model with 9 Gaussian kernels is converted into a model with 2 free-form kernels, which achieves 35% faster lithography simulation. In addition, simulation accuracy in CD is improved by 15%.
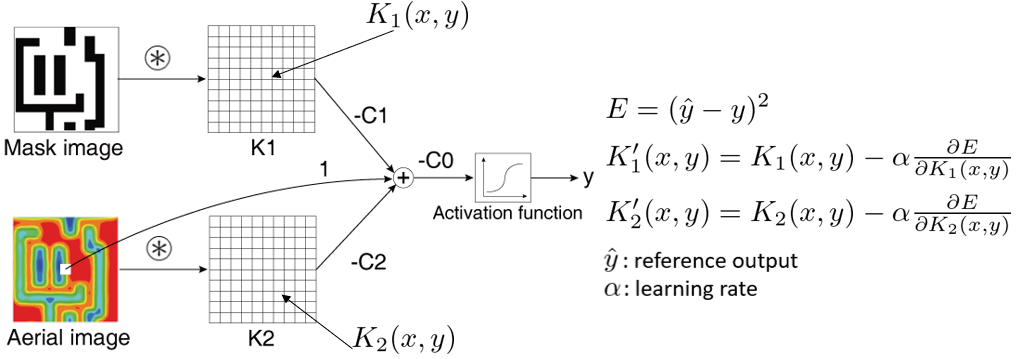