In this paper, sample-aware policy entropy regularization is proposed to enhance the conventional policy entropy regularization for better exploration. Exploiting the sample distribution obtainable from the replay buffer, the proposed sample-aware entropy regularization maximizes the entropy of the weighted sum of the policy action distribution and the sample action distribution from the replay buffer for sample-efficient exploration. A practical algorithm named diversity actor-critic (DAC) is developed by applying policy iteration to the objective function with the proposed sample-aware entropy regularization. Numerical results show that DAC significantly outperforms existing recent algorithms for reinforcement learning.

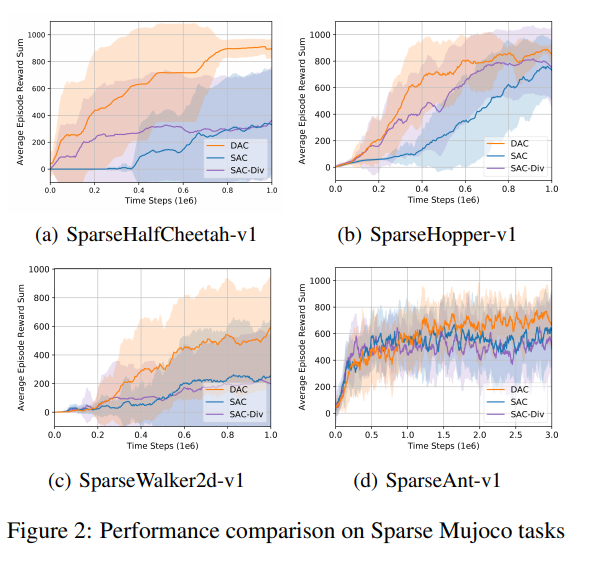
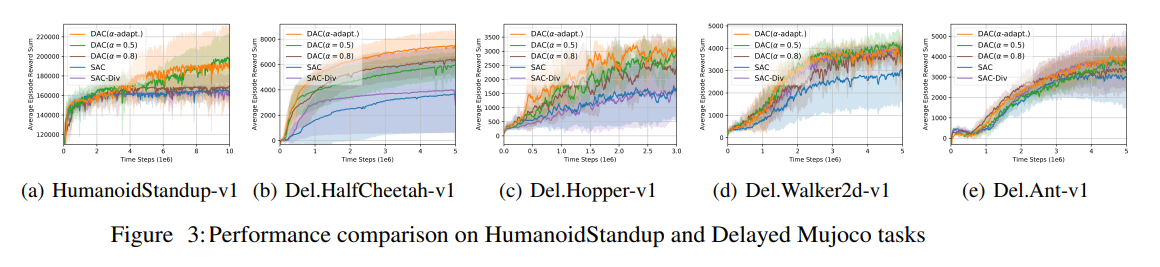