In sparse-reward reinforcement learning settings, extrinsic rewards from the environment are sparsely given to the agent. In this work, we consider intrinsic reward generation based on model prediction error. In typical model-prediction-error-based intrinsic reward generation, the agent has a learning model for the target value or distribution. Then, the intrinsic reward is designed as the error between the model prediction output and the target value or distribution, based on the fact that for less- or un-visited state-action pairs, the learned model yields larger prediction errors. This paper generalizes this model-prediction-error-based intrinsic reward generation method to the case of multiple prediction models. We propose a new adaptive fusion method relevant to the multiple-model case, which learns optimal prediction-error fusion across the learning phase to enhance the overall learning performance. Numerical results show that for representative locomotion tasks, the proposed intrinsic reward generation method outperforms most of the previous methods, and the gain is significant in some tasks.
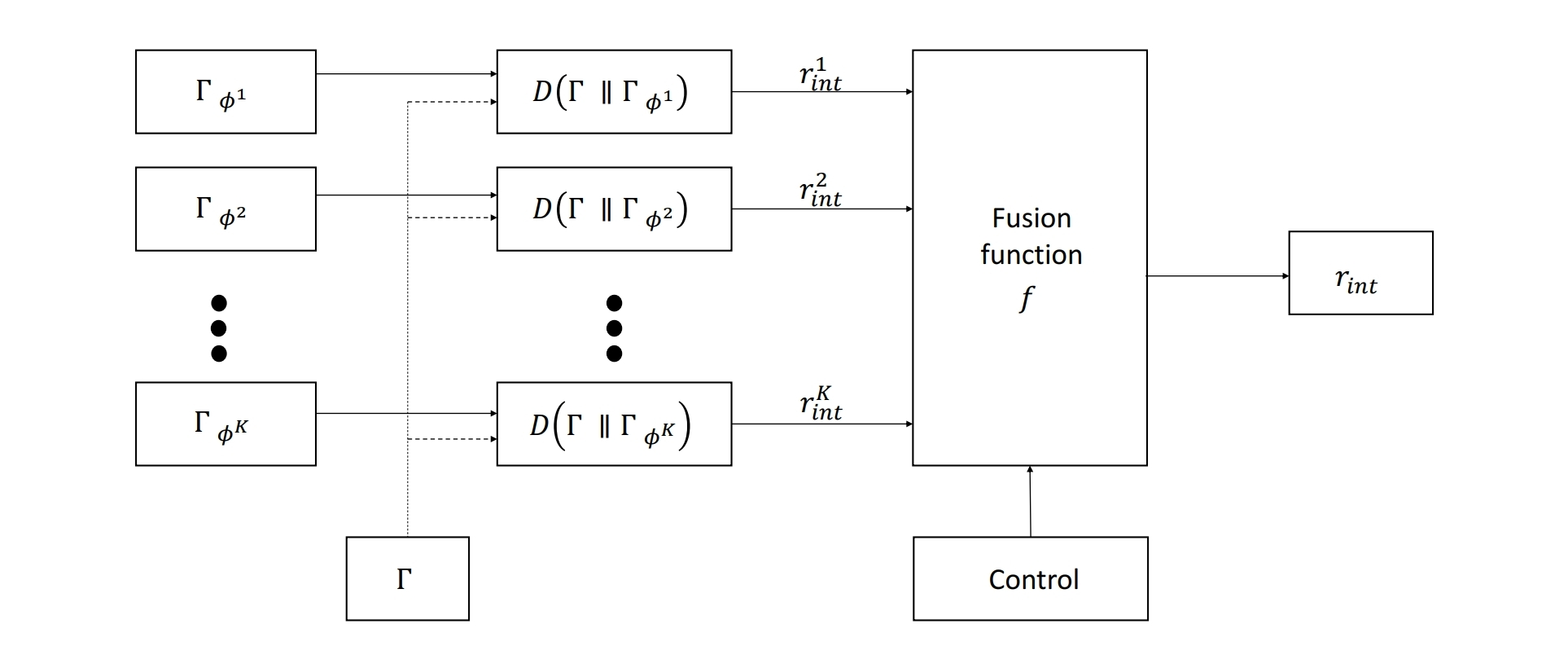
Fig. 1. Adaptive fusion of $K$ prediction errors from the multiple models.

Fig. 2. Mean number of visited states in continuous 4-room maze environment over ten random seeds (the blue curves represent performance of the proposed method).
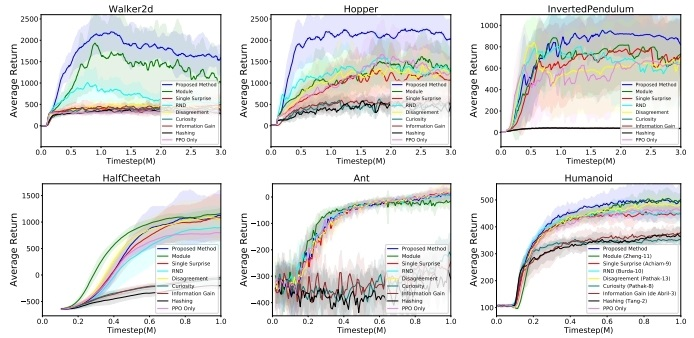
Fig. 3. Performance comparison in delayed Mujoco environments over ten random seeds (the blue curves represent performance of the the proposed method).