Conference/Journal, Year: WACV 2021
The performance of DNNs trained on clean images has been shown to decrease when the test images have common corruptions. In this work, we interpret corruption robustness as a domain shift and propose to rectify batch normalization (BN) statistics for improving model robustness. This is motivated by perceiving the shift from the clean domain to the corruption domain as a style shift that is represented by the BN statistics. We find that simply estimating and adapting the BN statistics on a few (32 for instance) representation samples, without retraining the model, improves the corruption robustness by a large margin on several benchmark datasets with a wide range of model architectures. For example, on ImageNet-C, statistics adaptation improves the top1 accuracy of ResNet50 from 39.2% to 48.7%. Moreover, we find that this technique can further improve state-of-the-art robust models from 58.1% to 63.3%.
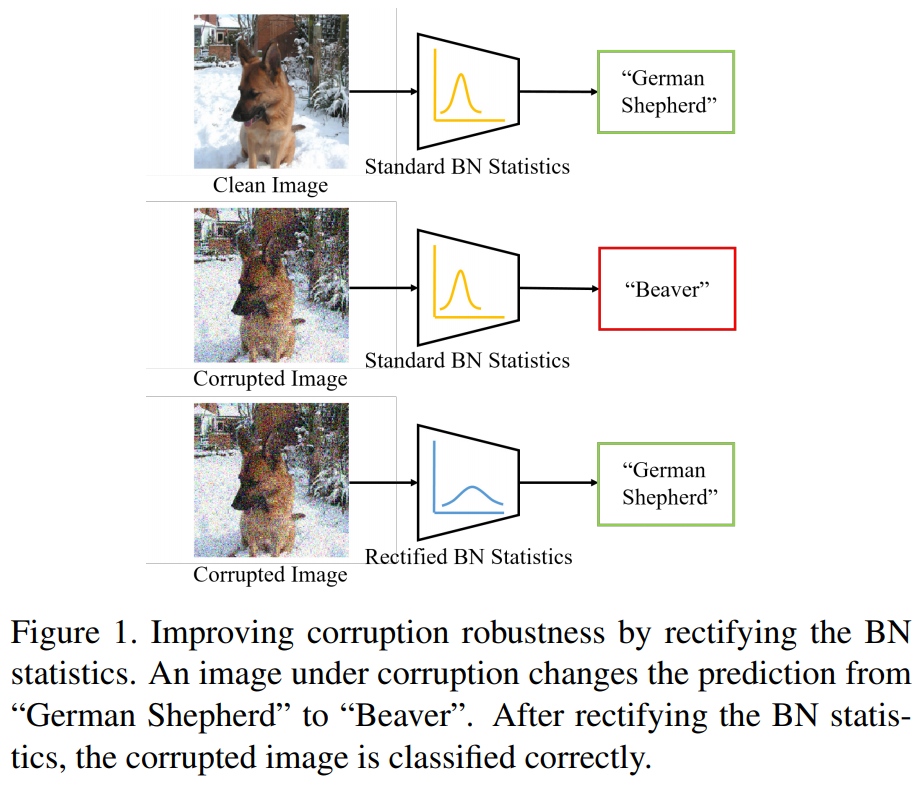