Data augmentation can impact the generalization performance of an image classification model in a significant way. However, it is currently conducted on the basis of trial and error, and its impact on the generalization performance cannot be predicted during training. This paper considers an influence function that predicts how generalization performance, in terms of validation loss, is affected by a particular augmented training sample. The influence function provides an approximation of the change in validation loss without actually comparing the performances that include and exclude the sample in the training process. Based on this function, a differentiable augmentation network is learned to augment an input training sample to reduce validation loss. The augmented sample is fed into the classification network, and its influence is approximated as a function of the parameters of the last fully-connected layer of the classification network. By backpropagating the influence to the augmentation network, the augmentation network parameters are learned. Experimental results on CIFAR-10, CIFAR-100, and ImageNet show that the proposed method provides better generalization performance than conventional data augmentation methods do.
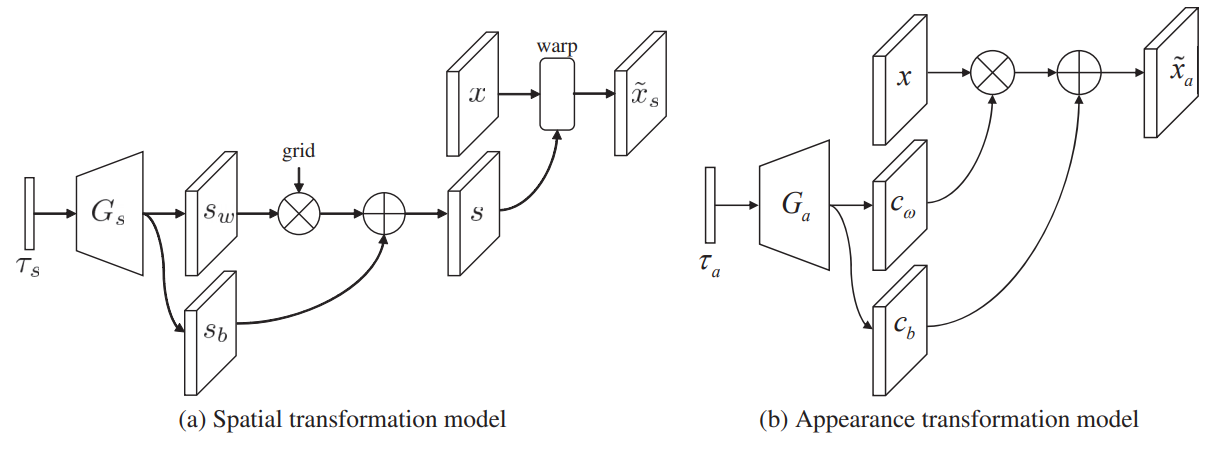
Figure 2. The proposed spatial and appearance transformation models for data augmentation.