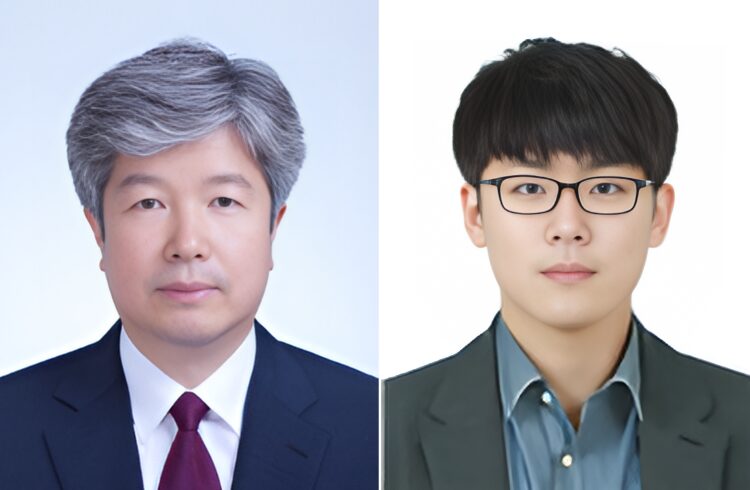
Professor Chan-Hyun Youn’s research team has developed a novel approach to Machine Unlearning, a critical aspect of AI safety, by introducing the past weight prediction model InvWNN. This model aims to selectively remove the influence of problematic data from AI models trained on such data. Existing methods often require access to the entire training dataset or face challenges with performance degradation. To address these issues, the team proposed a new method that leverages weight history to predict past weights and iteratively applies this process to progressively eliminate the influence of the data.
<Figure 1: Machine Unlearning Process of the Proposed InvWNN and Unlearning Trajectory>
This method gradually removes the problematic data’s influence through iterative fine-tuning on the problematic data and the past weight prediction. Notably, this approach operates effectively without access to the remaining data and can be applied to various datasets and architectures. Compared to existing methods, the proposed technique excels at accurately removing unnecessary knowledge from training data while minimizing side effects. Furthermore, it has been validated that this method can be directly applied to a variety of tasks without requiring additional procedures.
The research team demonstrated the high performance of their method across various benchmarks, showcasing its potential to significantly expand the practical applicability of machine unlearning technology. These findings will be presented at the 39th Annual AAAI Conference on Artificial Intelligence (AAAI 2025), one of the premier international conferences in the field of artificial intelligence, to be held in the United States in February next year, with the title “Learning to Rewind via Iterative Prediction of Past Weights for Unlearning.”