Professor YongMan Ro’s research team develops a multimodal large language model that surpasses the performance of GPT-4V
On June 20, 2024, Professor YongMan Ro’s research team announced that they have developed and released an open-source multimodal large language model that surpasses the visual performance of closed commercial models like OpenAI’s ChatGPT/GPT-4V and Google’s Gemini-Pro. A multimodal large language model refers to a massive language model capable of processing not only text but also image data types.
The recent advancement of large language models (LLMs) and the emergence of visual instruction tuning have brought significant attention to multimodal large language models. However, due to the support of abundant computing resources by large overseas corporations, very large models with parameters similar to the number of neural networks in the human brain are being created.
These models are all developed in private, leading to an ever-widening performance and technology gap compared to large language models developed at the academic level. In other words, the open-source large language models developed so far have not only failed to match the performance of closed large language models like ChatGPT/GPT-4V and Gemini-Pro, but also show a significant performance gap.
To improve the performance of multimodal large language models, existing open-source large language models have either increased the model size to enhance learning capacity or expanded the quality of visual instruction tuning datasets that handle various vision language tasks. However, these methods require vast computational resources or are labor-intensive, highlighting the need for new efficient methods to enhance the performance of multimodal large language models.
Professor YongMan Ro’s research team has announced the development of two technologies that significantly enhance the visual performance of multimodal large language models without significantly increasing the model size or creating high-quality visual instruction tuning datasets.
The first technology developed by the research team, CoLLaVO, verified that the primary reason existing open-source multimodal large language models perform significantly lower compared to closed models is due to a markedly lower capability in object-level image understanding. Furthermore, they revealed that the model’s object-level image understanding ability has a decisive and significant correlation with its ability to handle visual-language tasks.
To efficiently enhance this capability and improve performance on visual-language tasks, the team introduced a new visual prompt called Crayon Prompt. This method leverages a computer vision model known as panoptic segmentation to segment image information into background and object units. Each segmented piece of information is then directly fed into the multimodal large language model as input.
Additionally, to ensure that the information learned through the Crayon Prompt is not lost during the visual instruction tuning phase, the team proposed an innovative training strategy called Dual QLoRA.
This strategy trains object-level image understanding ability and visual-language task processing capability with different parameters, preventing the loss of information between them.
Consequently, the CoLLaVO multimodal large language model exhibits superior ability to distinguish between background and objects within images, significantly enhancing its one-dimensional visual discrimination ability.
The team pointed out that existing multimodal large language models use vision encoders that are semantically aligned with text, leading to a lack of detailed and comprehensive real-world scene understanding at the pixel level.
By combining the simple and efficient approach of CoLLaVO’s Crayon Prompt + DualQLoRA with MoAI’s array of computer vision models, the research team verified that their models outperformed closed commercial models like OpenAI’s ChatGPT/GPT-4V and Google’s Gemini-Pro.
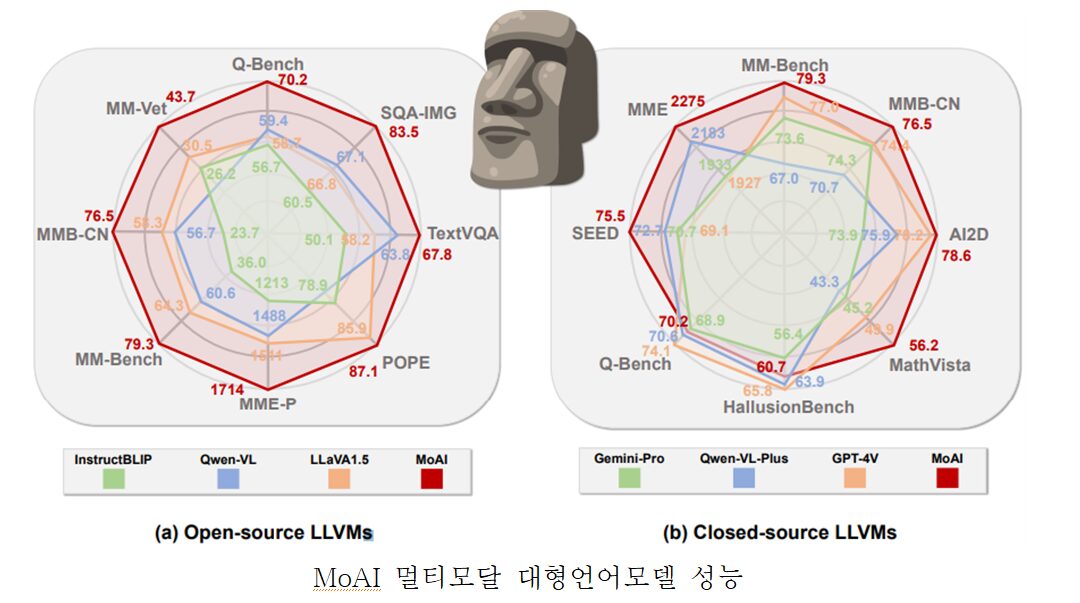
Accordingly, Professor YongMan Ro stated, “The open-source multimodal large language models developed by our research team, CoLLaVO and MoAI, have been recommended on Huggingface Daily Papers and are being recognized by researchers worldwide through various social media platforms. Since all the models have been released as open-source large language models, these research models will contribute to the advancement of multimodal large language models.”
This research was conducted at the Future Defense Artificial Intelligence Specialization Research Center and the School of Electrical Engineering of Korea Advanced Institute of Science and Technology (KAIST).
[1] CoLLaVO Demo GIF Video Clip https://github.com/ByungKwanLee/CoLLaVO
< CoLLaVO Demo GIF >
[2] MoAI Demo GIF Video Clip https://github.com/ByungKwanLee/MoAI
< MoAI Demo GIF >