Ph.D Candidate Jaehong Kim from professor Dongsu Han’s research team has developed a high-quality live video streaming system based on online learning.
The research team improved the quality of live video collected in real time from streamers through deep-learning-based super-resolution technology using the computing resources of the media server. Furthermore, they have overcome the limitations of previous works, performance limit that occurs when applying a previously trained neural network model to a new live video, by incorporating the online learning. In this system, a streamer transmits a patch, a part of a high-definition live video frame, to the media server through a separate transmission bandwidth with the live video. Then, the server optimizes the performance of the neural network model for the live video collected in real time, using the collected patches.
The research team reported that using this technology reduces the dependence on the streaming environment of the streamer and the performance constraints of the terminal in providing live streaming services. The technology also can provide live videos of various resolutions to the viewers of the distribution side. This technology improved user QoE of live video streaming system by 12-69% compared to the previous works.
Meanwhile, the results of this research were accepted and announced by ACM SIGCOMM, the best academic conference in the field of computer networking.
Detailed research information can be found at the link below.
Congratulations on Professor Dongsu Han’s remarkable achievement!
[Link]
Project website: http://ina.kaist.ac.kr/~livenas/
Paper Title: Neural-Enhanced Live Streaming: Improving Live Video Ingest via Online Learning
Paper link: https://dl.acm.org/doi/abs/10.1145/3387514.3405856
Conference presentation video: https://youtu.be/1giVlO6Rumg


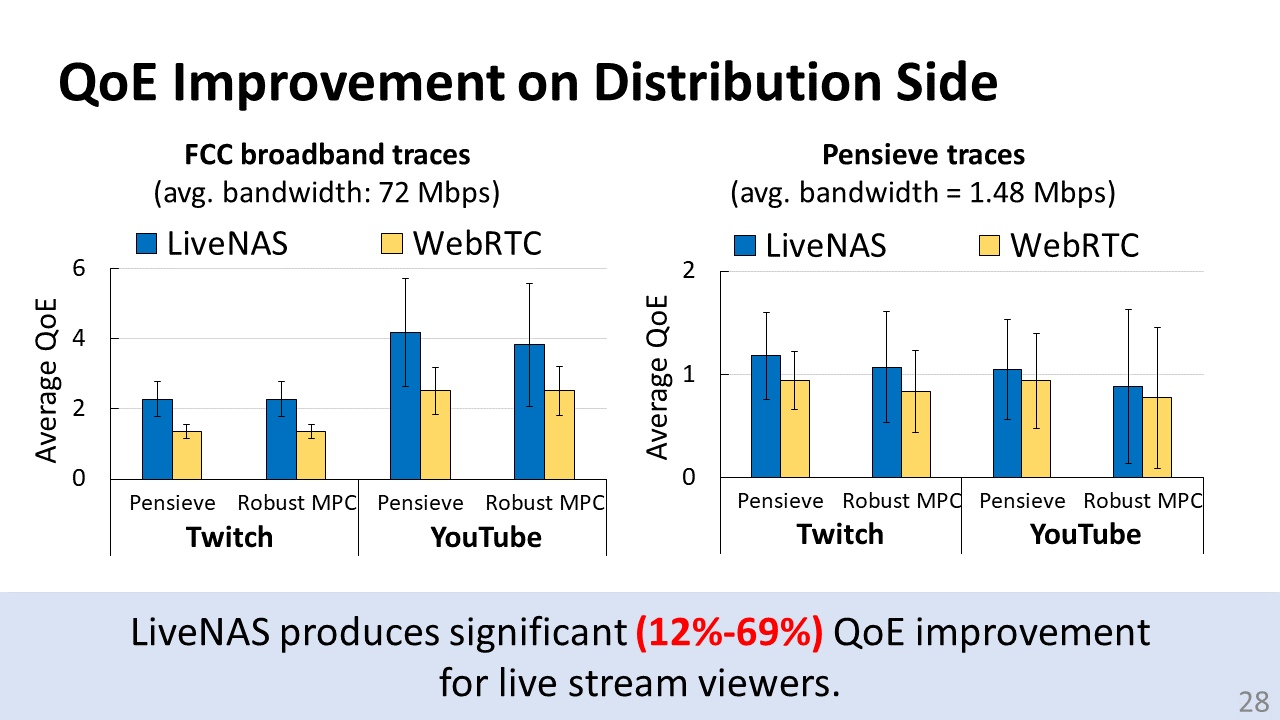
Ph.D Candidate Hyunho Yeo from professor Dongsu Han’s research team has developed a video super-resolution technology based on deep learning on commercial mobile devices.
The research team remarkably improved the processing speed and drastically reduced the power consumption by utilizing the video dependency. Unlike the conventional technology that applied super-resolution for every single frame of a video, in this study, super-resolution is applied only to a small portion of frames and the results are recycled in the rest of the frame. The quality improvement of images per unit computing resource was maximized by carefully selecting frames to apply super-resolution, and the process of recycling super-resolution results was implemented o operate in real time by utilizing the video dependency information loaded in the compressed video.
The research team reported that the use of this technology can significantly improve the satisfaction of mobile users for video streaming. The technology is expected to be used in various fields related to video transmission/storage. Meanwhile, the results of this research were also announced at ACM Mobicom (Annual International Conference on Mobile Computing and Networking), one of the best conference in the field of mobile.
Detailed research information can be found at the link below.
Congratulations on Professor Dongsu Han’s remarkable achievement!
[Link]
Project website: http://ina.kaist.ac.kr/~nemo
Paper: https://dl.acm.org/doi/10.1145/3372224.3419185
Conference presentation video:
https://www.youtube.com/watch?v=GPHlAUYCk18&ab_channel=ACMSIGMOBILEONLINE
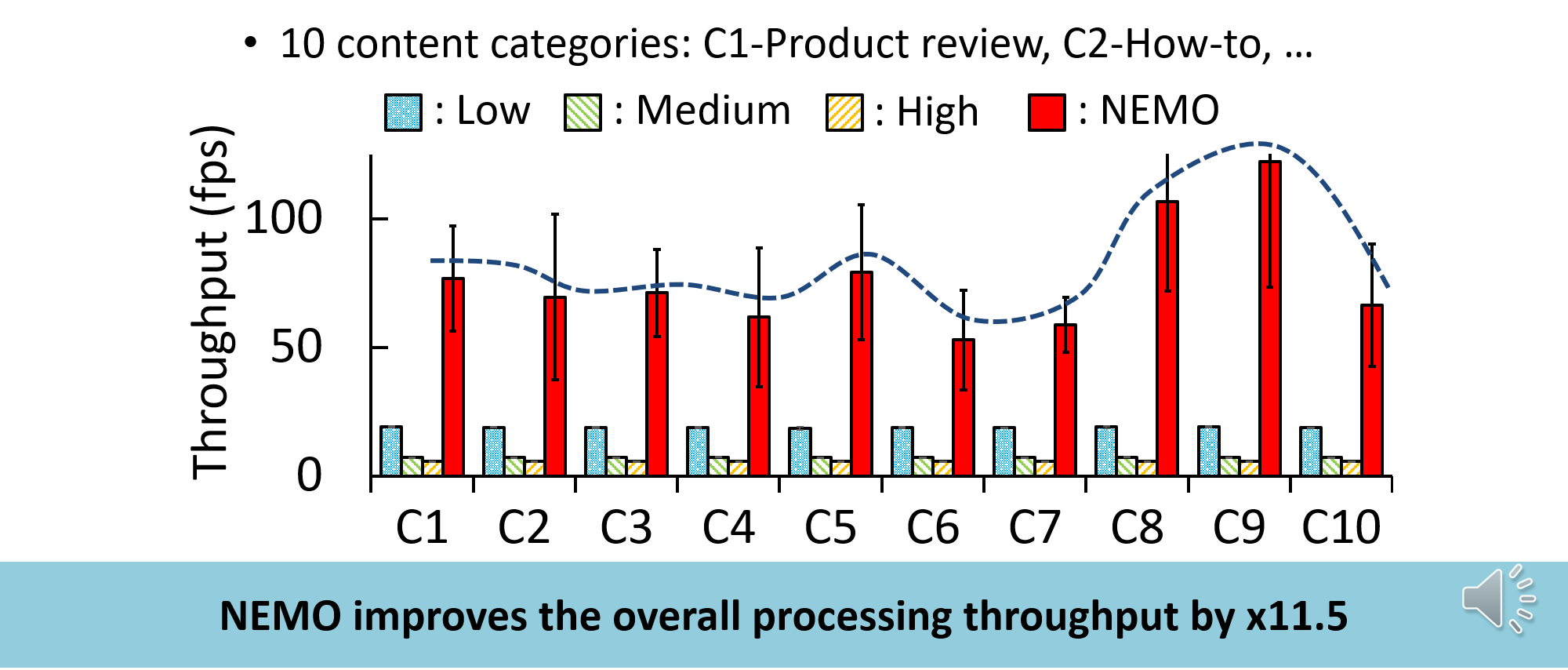
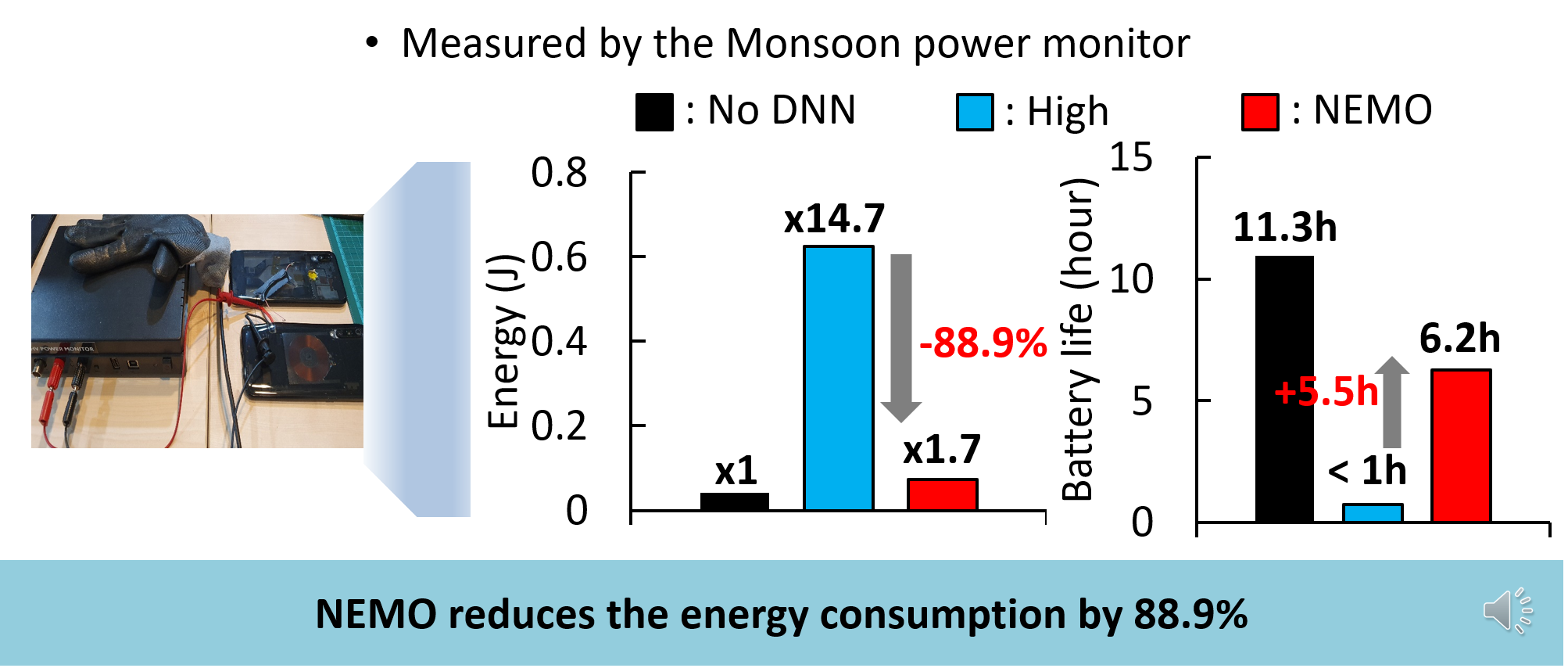
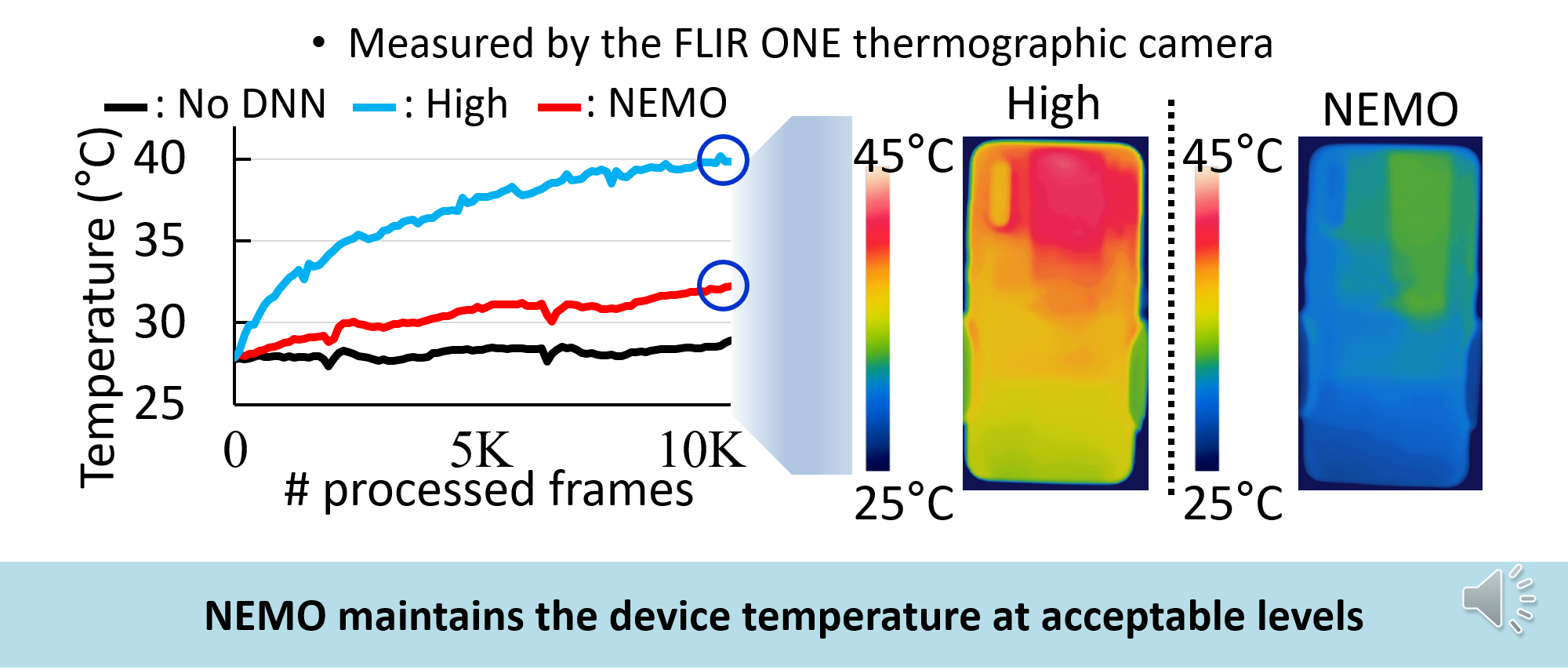
우리 학부 한동구 교수님 연구실 여현호 박사과정생이 주도하여 모바일 기기에서의 딥러닝 기반 비디오 초해상화 기술을 개발했습니다. 본 연구팀은 비디오 종속성을 활용하여 처리속도를 대폭적으로 향상시키고 전력 소모량을 획기적으로 감소시켰습니다. 매 프레임마다 초해상화를 적용하던 종래의 기술과 다르게, 본 연구에서는 일부 프레임에만 초해상화를 적용하고 나머지 프레임에서는 이 결과를 재활용합니다. 또한 초해상화를 적용할 프레임을 신중히 선택하여 단위 컴퓨팅 자원 당 화질 향상을 극대화하였고, 압축된 비디오 내에 탑재되어 있는 비디오 종속성 정보를 활용함으로써 초해상화 결과를 재활용하는 과정을 실시간으로 구현했습니다.
연구팀은 해당 기술을 이용하면 모바일 유저의 비디오 스트리밍 만족도를 대폭적으로 향상시킬 수 있다고 밝혔습니다. 또한 해당 기술은 비디오 전송/저장과 관련한 다양한 분야에서 활용될 것으로 예측됩니다. 한편, 이번 연구 성과는 모바일 최고 권위 학회인 ACM 모비콤 (Annual International Conference on Mobile Computing and Networking)에서 발표되기도 했습니다.
자세한 연구 내용은 아래의 링크에서 확인하실 수 있습니다.
컴퓨터 디비젼 한동수 교수님의 성과에 박수를 보내드립니다.
[논문정보]
학회명: The 26th Annual International Conference on Mobile Computing and Networking
논문명: NEMO: enabling neural-enhanced video streaming on commodity mobile devices
저자: 여현호 박사과정생, 정찬주 학부과정생, 정영목 박사과정생, 예준철 석사과정생
논문 링크: http://ina.kaist.ac.kr/~nemo
e research team of Professors Song-Min Kim and Yung Yi succeeded in developing IoT technology that can communicate with ultra-low power. The news was reported to the leading media in Korea. The research was conducted in cooperation with Professor Ji-Hoon Ryoo, Department of Computer Science at the State University of New York State Korea.
Existing technology had a problem that the gateway power consumption is too large so that a wired power supply is needed. The research team tackled this problem through “backscattering technology.” The “backscattering technology” does not directly generate the device’s wireless signal, but transmits information by reflecting the radiated signal existing in the air. Therefore, it does not consume power to generate the wireless signal. Researchers have found that using the technology can significantly reduce the cost of installation and maintenance. The technology expands the efficient connectivity of the Internet of Things. It is expected to be utilized in various fields in the future. Meanwhile, the results of this study were also presented at “ACM Mobisys 2020” held in Toronto, Canada in June.
We would like to applaud the achievements of Computer Division Professors Song-Min Kim and Yung Yi.
Professor Min-Soo Rhu’s research team at our faculty has developed the world’s first mix-type artificial intelligence (AI) accelerator based on the ‘Multi-chip module’ (MCM) technology to speed up the recommendation system.
An article on the research results was reported in a Korea IT newspaper on July 6th.
This study corresponds to a technology that significantly enhances the performance of AI-based recommendation systems while minimizing the structural changes of existing server systems. This increased the performance by up to 17 times compared to the existing recommendation system.
The research team carried out this study with the support of Samsung Electronics Future Technology Promotion Center and also received help from Intel Labs on MCM-based chips.
Related research papers were presented at ACM/IEEE (ISCA) last month, which is one of the best international academic conferences in the field of the computer system architecture.
Professor Min-Soo Rhu expressed his ambition to promote active educational-industrial cooperation to help domestic memory semiconductor companies lead the AI accelerator market.
You can check the detailed research achievements of Professor Min-Soo Rhu’s research team in the press release link below and the blog post contributed by the professor.
[Link]
https://www.etnews.com/20200706000286 (Korea IT newspaper)
https://www.sigarch.org/building-performance-scalable-and-composable-mac…
(Article by Professor Min-Soo Rhu, ACM SIGARCH Blog)
The research team of Professor Hyunchul Shim accomplished the 3rd place in the final of AlphaPilot Orlando Race held by Lockheed Martin on last Dec. 6th.
Lockheed Martin held AlphaPilot Orlando Race at the beginning of this year and awarded a million USD (1.2 billion KRW) to the race winner-drone which cruises fast on the intricate course. Over 420 teams challenged to participate in the final of the race, but only nine teams were selected for the final.
The research team of Prof. Shim was chosen confidently for the final as one of the nine teams among 420 teams who attended last April.
A drone race is already popular enough to be shown live by such as ESPN in the US. A participant wears a first-person view (FPV) goggle, which shows the real-time image transferred from the camera on the drone, for control.
Congratulations to Prof. Shim and his research team for the great accomplishment against distinguished teams.
Information related to the race and detail can be found through the link below.
[Link]
https://www.lockheedmartin.com/en-us/news/events/ai-innovation-challenge.html
Professor Min-Soo Rhu’s lab has succeeded in developing an “AI-based recommendation technology” acceleration system and their work has been reported in the media. The system accelerates AI-based recommendation algorithms from 6x up to 17x.
The AI-based recommendation service refers to advertisement recommendations that can be found easily on portal sites such as Google and Naver. This is a service based on a deep learning system and recommends personalized information by using a user’s search history through AI technology. The key element of such service is the “computation time of the algorithm”. Since it is based on real-time information, the quality of the service is determined by how quickly the recommendation results are derived. Besides, this is directly related to user satisfaction and also the profit generation of the company.
Professor Min-Soo Rhu’s research team has devised a solution to effectively reduce execution time by developing an AI accelerator computing system based on memory, which improves the so-called ‘memory bottleneck’. The system proposed by the team is a ‘Processing in Memory (PI614M)’ technology that places an AI accelerator close to the memory. This is noteworthy in that it effectively reduces data transfers and memory access times.
The developed technology is receiving positive reviews and can be utilized in various fields. Professor Min-Soo Rhu has expressed his position to cooperate with domestic companies to win the leadership of the AI accelerator market.
The results of this research are globally recognized for their excellence, including to be listed on the 2019 IEEE Micro Top Picks – Honorable Mention List, which was presented in 26 of the most influential results among the hundreds of papers published in computer system architecture in 2019.
In the meantime, this research was carried out with the support from Samsung Electronics Future Technology Foundation. Congratulations on your achievements.
Title: TensorDIMM: A Practical Near-Memory Processing Architecture for Embeddings and Tensor Operations in Deep Learning
Authors: Youngeun Kwon, Yunjae Lee, and Minsoo Rhu
Recent studies from several hyper scalars pinpoint to embedding layers as the most memory-intensive deep learning (DL) algorithm being deployed in today’s data centers. This paper addresses the memory capacity and bandwidth challenges of embedding layers and the associated tensor operations. We present our vertically integrated hardware/software co-design, which includes a custom DIMM module enhanced with near-memory processing cores tailored for DL tensor operations. These custom DIMMs are populated inside a GPU-centric system interconnect as a remote memory pool, allowing GPUs to utilize for scalable memory bandwidth and capacity expansion. A prototype implementation of our proposal on real DL systems shows an average 6.2−17.6× performance improvement on state-of-the-art DNN-based recommender systems.

Link: https://arxiv.org/pdf/1908.03072.pdf
The research study authored by Dae-Woo Kim, Sang-Woo Moon, David Hostallero, Wan-Ju Kang, Tae-Young Lee, Kyunng-Hwan Son, and Yung Yi was accepted at 7th International Conference on Learning Representations (ICLR 2019)
Title: Learning to Schedule Communication in Multi-agent Reinforcement Learning
Authors: Dae-Woo Kim, Sang-Woo Moon, David Hostallero, Wan-Ju Kang, Tae-Young Lee, Kyung-Hwan Son and Yung Yi
We present a first-of-its-kind study of communication-aided cooperative multi-agent reinforcement learning tasks with two realistic constraints on communication: (i) limited bandwidth and (ii) medium contention. Bandwidth limitation restricts the amount of information that can be exchanged by any agent accessing the medium for inter-agent communication, whereas medium contention confines the number of agents to access the channel itself, as in the state-of-the-art wireless networking standards, such as 802.11. These constraints call for a certain form of scheduling. In that regard, we propose a multi-agent deep reinforcement learning framework, called SchedNet, in which agents learn how to schedule themselves, how to encode messages, and how to select actions based on received messages and on its own observation of the environment. SchedNet enables the agents to decide, in a distributed manner, among themselves who should be entitled to broadcasting their encoded messages, by learning to gauge the importance of their (partially) observed information. We evaluate SchedNet against multiple baselines under two different applications, namely, cooperative communication and navigation, and predator-prey. Our experiments show a non-negligible performance gap between SchedNet and other mechanisms such as the ones without communication with vanilla scheduling methods, e.g., round robin, ranging from 32% to 43%.
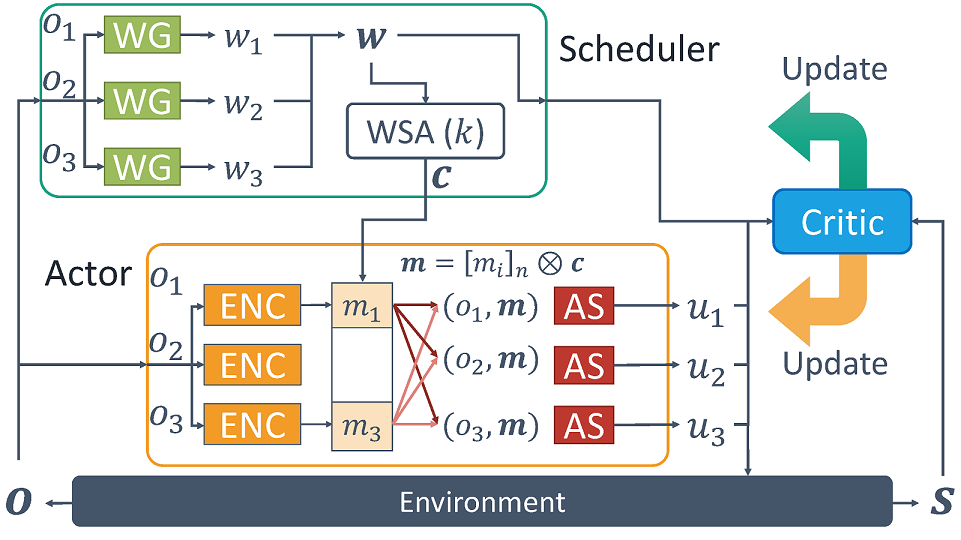

The research study authored by Joon-Ki Hong* (KAIST EE), Soon-Il Kwon* (SNUH), Eue-Keun Choi (SNUH), Eui-Jae Lee (SNUH), David Earl Hostallero (KAIST EE), Wan-Ju Kang (KAIST EE), Byung-Hwan Lee (Skylabs), Eui-Rim Jeong (Hanbat University), Bon-Kwon Koo (SNUH), Se-Il Oh (SNUH), Yung Yi (KAIST EE) was accepted at JMIR mHealth and uHealth 7.6(2019)
Title: Deep Learning Approaches to Detect Atrial Fibrillation Using Photoplethysmographic Signals: Algorithms Development Study
Authors: Joon-Ki Hong*, Soon-Il Kwon*, Eue-Keun Choi, Eui-Jae Lee, David Earl Hostallero, Wan Ju Kang, Byung-Hwan Lee, Eui-Rim Jeong, Bon-Kwon Koo, Se-Il Oh, Yung Yi (* these authors contributed equally)
Wearable devices have evolved as screening tools for atrial fibrillation (AF). A photoplethysmographic (PPG) AF detection algorithm was developed and applied to a convenient smartphone-based device with good accuracy. However, patients with paroxysmal AF frequently exhibit premature atrial complexes (PACs), which result in poor unmanned AF detection, mainly because of rule-based or handcrafted machine learning techniques that are limited in terms of diagnostic accuracy and reliability. We developed deep learning (DL) based AF classifiers based on 1-dimensional convolutional neural network (1D-CNN) and recurrent neural network (RNN) architectures and examined 75 patients with AF who underwent successful elective direct-current cardioversion (DCC). New DL classifiers could detect AF using PPG monitoring signals with high diagnostic accuracy (97.58 %) even with frequent PACs and could outperform previously developed AF detectors. Although diagnostic performance decreased as the burden of PACs increased, performance improved when samples from more patients were trained. Moreover, the reliability of the diagnosis could be indicated by the confidence level (CL). Wearable devices sensing PPG signals with DL classifiers should be validated as tools to screen for AF.
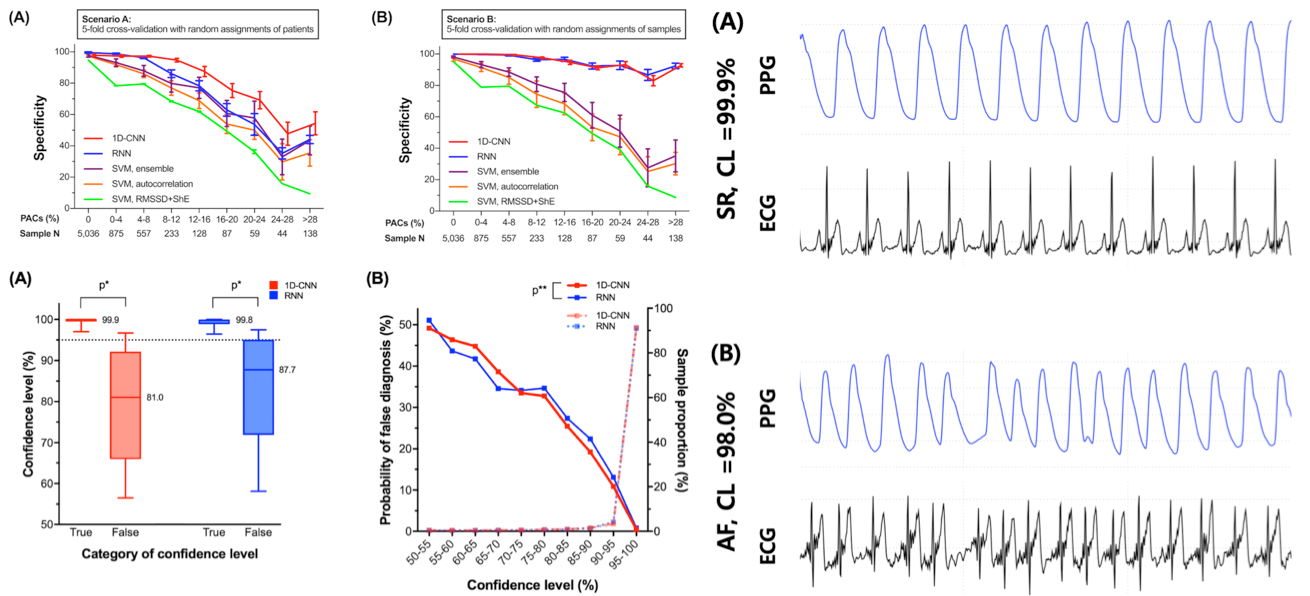