Title of the paper: SoftGroup for 3D Instance Segmentation on Point Clouds
Conference: The IEEE / CVF Computer Vision and Pattern Recognition Conference (CVPR) 2022
Date & Location: June 21, 2022 (Tue) / New Orleans, Louisiana, USA
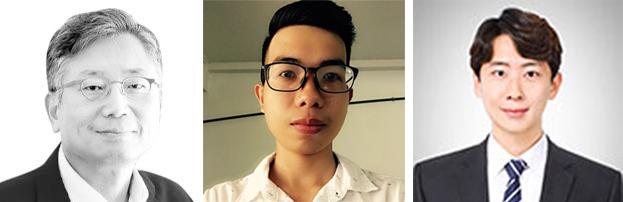
[(from the left) Professor Chang D. Yoo, Vu Van Thang (Ph.D candidate), Kookhoi Kim (Master’s candidate)]
3D datasets are being utilized in various fields recently such as autonomous driving, robotics, and AR. 3D point clouds are data comprised of sets of 3D points and this study developed SoftGroup, a precision object segmentation technology based on 3D point cloud. SoftGroup allows each point to be associated with multiple classes to mitigate problems stemming from semantic prediction errors and surpasses prior state-of-the-art methods by more than 8% in terms of performance. Allowing for 3D instance segmentation of point clouds that contain more precise information of 3D space compared to traditional photographs, SoftGroup shows high potential for utilization in fields that leverage 3D point clouds.
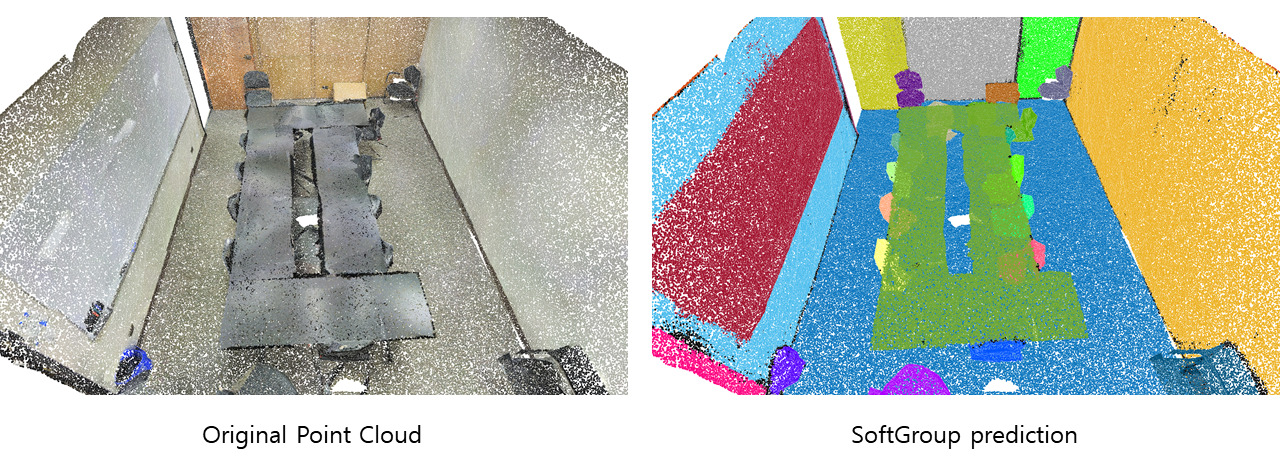
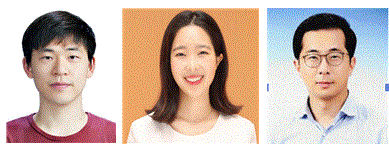
[Prof. Yoon, Young-Gyu, Kim, Jeewon (PH.D candidate), Prof. Chang, Jae-Byum From Left: ]
KAIST EE Professor Yoon, Young-Gyu’s and Materials Science and Engineering Professor Chang, Jae-Byum’s team conducted a joint research published as PICASSO, a simultaneous detector of multiple markers capable of capturing five times as many protein markers in comparison with existing techniques.
Recent studies have shown that protein markers in the cancer tissues manifest differently across cancer patients. Related research findings indicate that such a difference determines the cancer progress as well as reactivity to anti-cancer drugs. This is why detecting multiple markers, also known as multiplexed imaging, from cancer tissues is deemed essential.
The research team’s development, PICASSO, is capable of detecting 15 – and at most 20 – protein markers at once via fluorescence imaging. This development was made possible by utilizing fluorophores exhibiting similar emission spectra simultaneously and accurately isolating each type of the fluorophores with blind source separation. Said technique does not require specialized reagent or expensive equipment and is thus considered a promising method of better diagnosis of cancer and drug development as well as protein marker discovery.
EE PH.D student Kim, Jeewon and Materials Science and Engineering student Seo, Junyoung, and alumnus Sim, Yeonbo have led the research as first authors, and their paper was published in Nature Communications, book 13, May as “PICASSO allows ultra-multiplexed fluorescence imaging of spatially overlapping proteins without reference spectra measurement”.
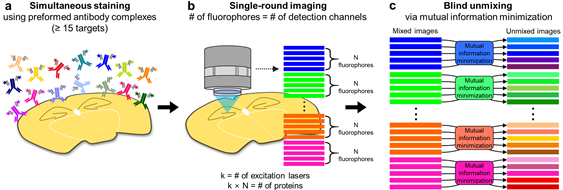
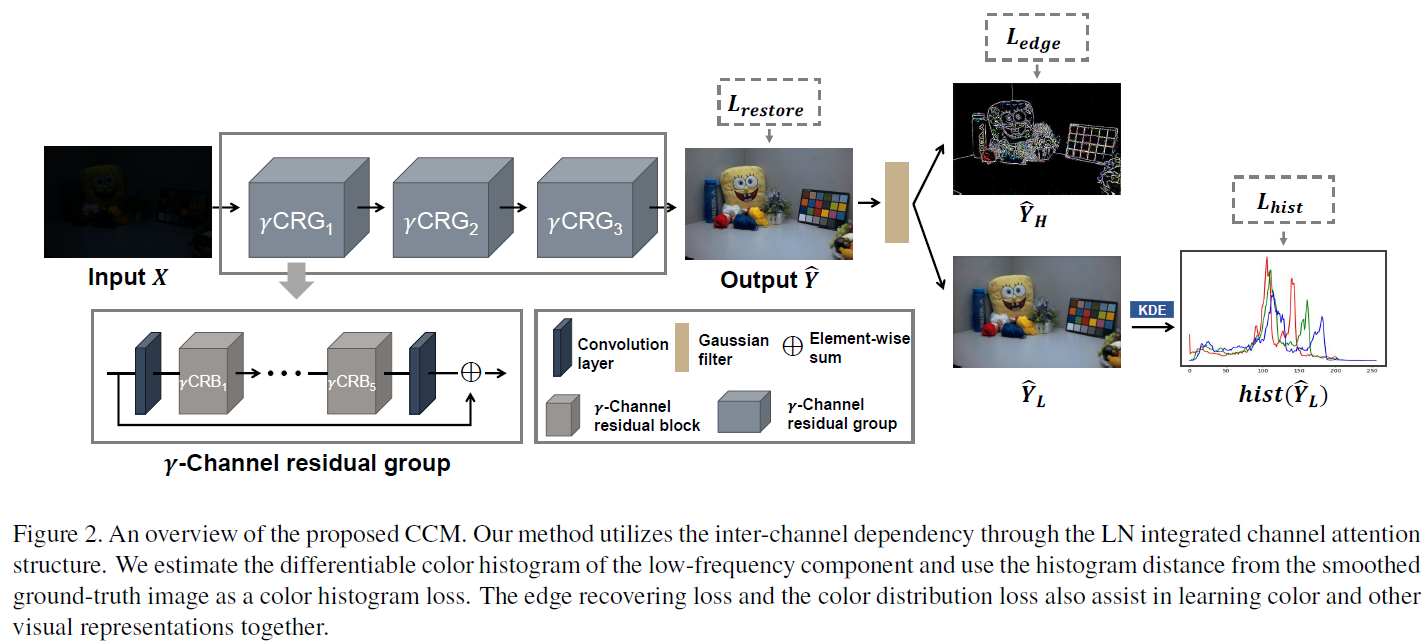
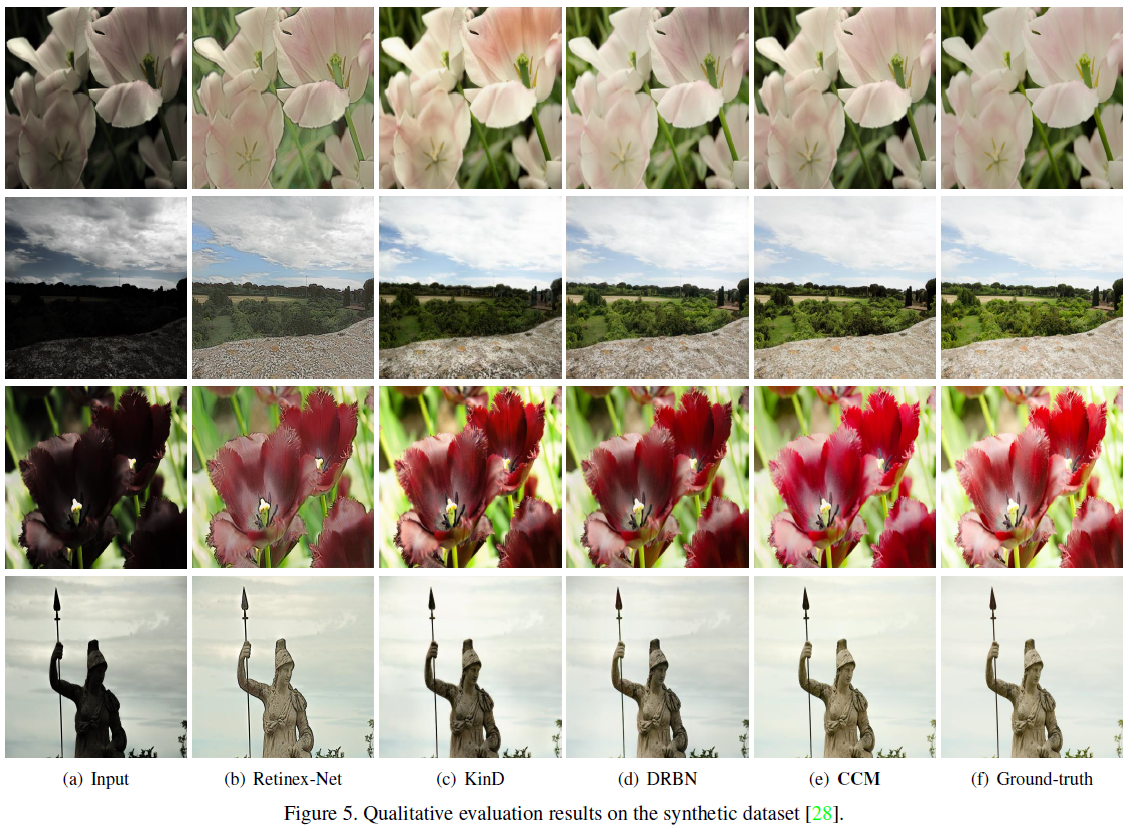
Color conveys important information about the visible world. However, under low-light conditions, both pixel intensity, as well as true color distribution, can be significantly shifted. Moreover, most of such distortions are non-recoverable due to inverse problems. In the present study, we utilized recent advancements in learning-based methods for low-light image enhancement. However, while most “deep learning” methods aim to restore high-level and object-oriented visual information, we hypothesized that learning-based methods can also be used for restoring color-based information. To address this question, we propose a novel color representation learning method for low-light image enhancement. More specifically, we used a channel-aware residual network and a differentiable intensity histogram to capture color features. Experimental results using synthetic and natural datasets suggest that the proposed learning scheme achieves state-of-the-art performance. We conclude from our study that inter-channel dependency and color distribution matching are crucial factors for learning color representations under low-light conditions.
Developing an agent in reinforcement learning (RL) that is capable of performing complex control tasks directly from high-dimensional observation such as raw pixels is yet a challenge as efforts are made towards improving sample efficiency and generalization. This paper considers a learning framework for Curiosity Contrastive Forward Dynamics Model (CCFDM) in achieving a more sample-efficient RL based directly on raw pixels. CCFDM incorporates a forward dynamics model (FDM) and performs contrastive learning to train its deep convolutional neural network-based image encoder (IE) to extract conducive spatial and temporal information for achieving a more sample efficiency for RL. In addition, during training, CCFDM provides intrinsic rewards, produced based on FDM prediction error, encourages the curiosity of the RL agent to improve exploration. The diverge and less-repetitive observations provide by both our exploration strategy and data augmentation available in contrastive learning improve not only the sample efficiency but also the generalization. Performance of existing model-free RL methods such as Soft Actor-Critic built on top of CCFDM outperforms prior state-of-the-art pixelbased RL methods on the DeepMind Control Suite benchmark.
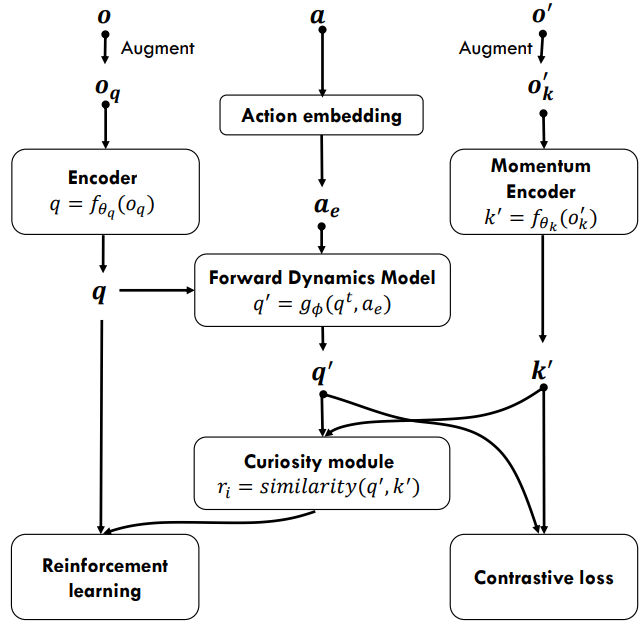
Figure 13. The proposed Curiosity Contrastive Forward Dynamics Model.
A bounding box commonly serves as the proxy for 2D object detection. However, extending this practice to 3D detection raises sensitivity to localization error. This problem is acute on flat objects since small localization error may lead to low overlaps between the prediction and ground truth. To address this problem, this paper proposes Sphere Region Proposal Network (SphereRPN) which detects objects by learning spheres as opposed to bounding boxes. We demonstrate that spherical proposals are more robust to localization error compared to bounding boxes. The proposed SphereRPN is not only accurate but also fast. Experiment results on the standard ScanNet dataset show that the proposed SphereRPN outperforms the previous state-of-the-art methods by a large margin while being 2x to 7x faster.
Figure 12: Architecture of the proposed SphereRPN
This paper proposes Weakly-supervised Moment Retrieval Network (WMRN) for Video Corpus Moment Retrieval (VCMR), which retrieves pertinent temporal moments related to natural language query in a large video corpus. Previous methods for VCMR require full supervision of temporal boundary information for training, which involves a labor-intensive process of annotating the boundaries in a large number of videos. To leverage this, the proposed WMRN performs VCMR in a weakly-supervised manner, where WMRN is learned without ground-truth labels but only with video and text queries. For weakly-supervised VCMR, WMRN addresses the following two limitations of prior methods: (1) Blurry attention over video features due to redundant video candidate proposals generation, (2) Insufficient learning due to weak supervision only with video-query pairs. To this end, WMRN is based on (1) Text Guided Proposal Generation (TGPG) that effectively generates text guided multi-scale video proposals in the prospective region related to query, and (2) Hard Negative Proposal Sampling (HNPS) that enhances video-language alignment via extracting negative video proposals in positive video sample for contrastive learning. Experimental results show that WMRN achieves state-of-the-art performance on TVR and DiDeMo benchmarks in the weakly-supervised setting. To validate the attainments of proposed components of WMRN, comprehensive ablation studies and qualitative analysis are conducted.
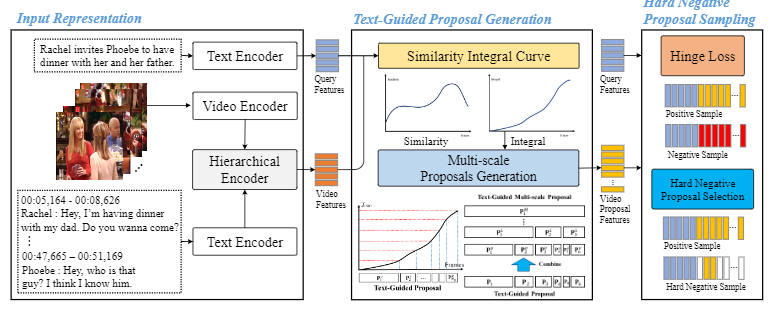
Figure 10. Weakly-supervised Moment Retrieval Network (WMRN) composed of two main modules: Text Guided Proposal Generation (TGPG) and Hard Negative Proposal Sampling (HNPS). The WMRN is designed based on following two insights: (1) Effectively generate multi-scale proposals pertinent to given query reduces the redundant computations and enhances attention performance and, (2) extract hard negative samples in contrastive learning to contribute to high-level interpretability. TGPG increases the number of candidate proposals in proximity of moments related to query, while decreases proposals in unrelated moments. HNPS selects negative samples in positive videos, which contribute to enhanced contrastive learning
Although Question-Answering has long been of research interest, its accessibility to users through a speech interface and its support to multiple languages have not been addressed in prior studies. Towards these ends, we present a new task and a synthetically-generated dataset to do Fact-based Visual Spoken-Question Answering (FVSQA). FVSQA is based on the FVQA dataset, which requires a system to retrieve an entity from Knowledge Graphs (KGs) to answer a question about an image. In FVSQA, the question is spoken rather than typed. Three sub-tasks are proposed: (1) speech-to-text based, (2) end-to-end, without speech-to-text as an intermediate component, and (3) cross-lingual, in which the question is spoken in a language different from that in which the KG is recorded. The end-to-end and cross-lingual tasks are the first to require world knowledge from a multi-relational KG as a differentiable layer in an end-to-end spoken language understanding task, hence the proposed reference implementation is called WorldlyWise (WoW). WoW is shown to perform endto-end cross-lingual FVSQA at same levels of accuracy across 3 languages – English, Hindi, and Turkish.
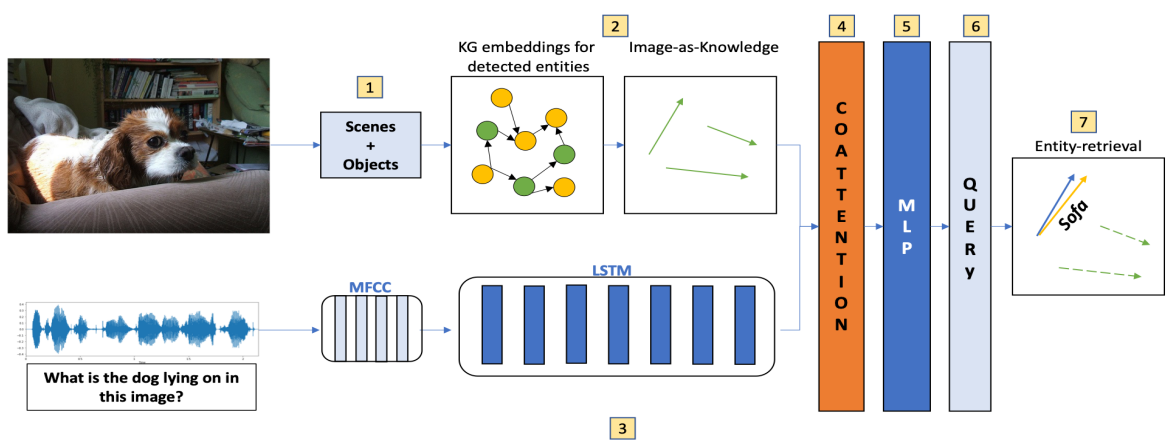
.
Figure 9: Architecture of the proposed method
Cascaded architectures have brought significant performance improvement in object detection and instance segmentation. However, there are lingering issues regarding the disparity in the Intersection-over-Union (IoU) distribution of the samples between training and inference. This disparity can potentially exacerbate detection accuracy. This paper proposes an architecture referred to as Sample Consistency Network (SCNet) to ensure that the IoU distribution of the samples at training time is close to that at inference time. Furthermore, SCNet incorporates feature relay and utilizes global contextual information to further reinforce the reciprocal relationships among classifying, detecting, and segmenting sub-tasks. Extensive experiments on the standard COCO dataset reveal the effectiveness of the proposed method over multiple evaluation metrics, including box AP, mask AP, and inference speed. In particular, while running 38% faster, the proposed SCNet improves the AP of the box and mask predictions by respectively 1.3 and 2.3 points compared to the strong Cascade Mask R-CNN baseline.
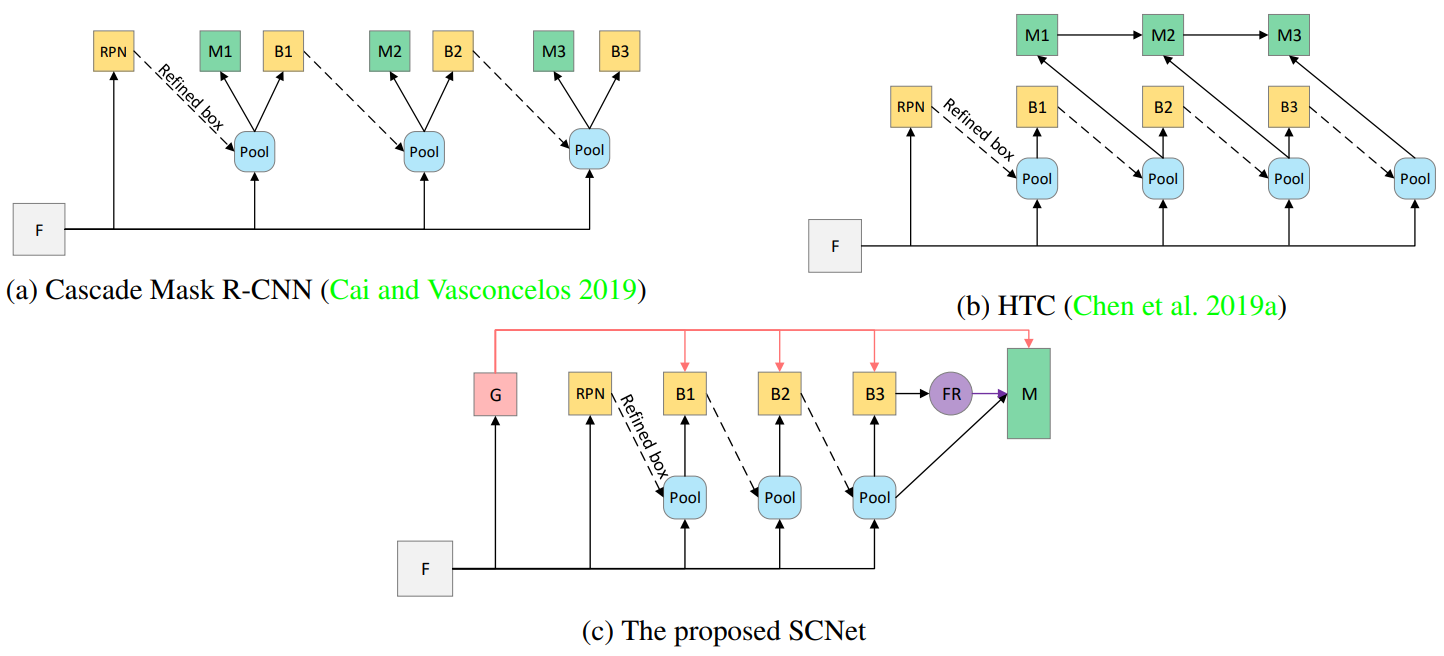
Figure 8. Architecture of cascade approaches: (a) Cascade Mask R-CNN. (b) Hybrid Task Cascade (HTC). (c) the proposed SCNet. Here, “F”, “RPN”, “Pool”, “B”, “M”, “FR” and “G” denote image features, Region Proposal Network, region-wise pooling, box branch, mask branch, feature relay, and global context branch, respectively.
This paper considers a video caption generating network referred to as Semantic Grouping Network (SGN) that attempts (1) to group video frames with discriminating word phrases of partially decoded caption and then (2) to decode those semantically aligned groups in predicting the next word. As consecutive frames are not likely to provide unique information, prior methods have focused on discarding or merging repetitive information based only on the input video. The SGN learns an algorithm to capture the most discriminating word phrases of the partially decoded caption and a mapping that associates each phrase to the relevant video frames – establishing this mapping allows semantically related frames to be clustered, which reduces redundancy. In contrast to the prior methods, the continuous feedback from decoded words enables the SGN to dynamically update the video representation that adapts to the partially decoded caption. Furthermore, a contrastive attention loss is proposed to facilitate accurate alignment between a word phrase and video frames without manual annotations. The SGN achieves state-of-the-art performances by outperforming runner-up methods by a margin of 2.1%p and 2.4%p in a CIDEr-D score on MSVD and MSR-VTT datasets, respectively. Extensive experiments demonstrate the effectiveness and interpretability of the SGN.
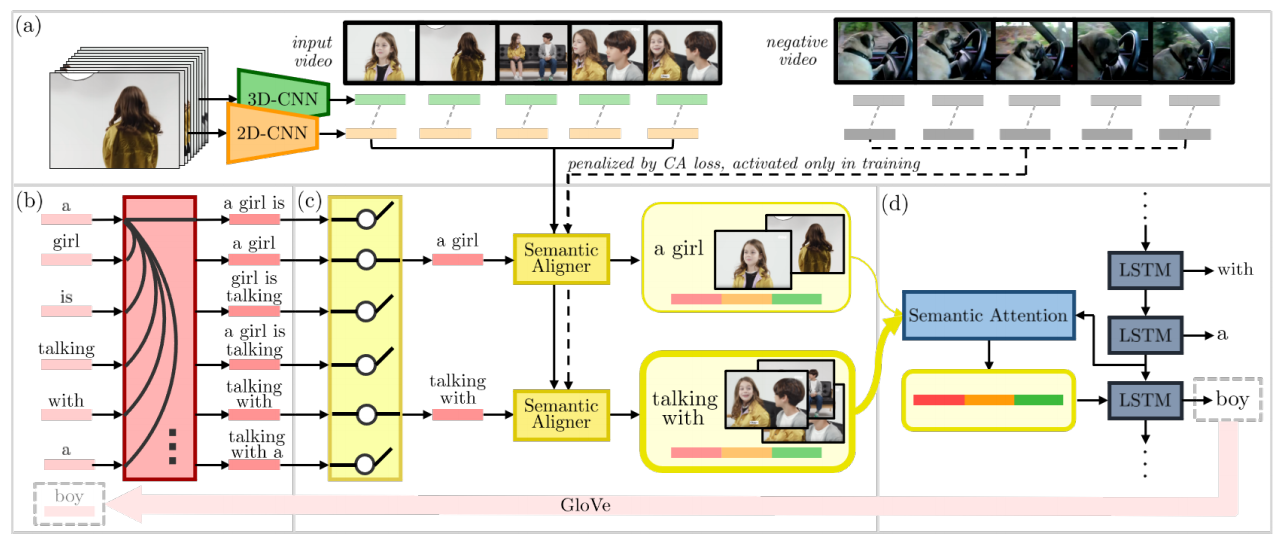
Figure 7: The SGN consists of (a) Visual Encoder, (b) Phrase Encoder, (c) Semantic Grouping, and (d) Decoder. In training, a negative video is introduced in addition to the input video for calculating the CA loss. The words predicted by the Decoder are added to the input of the Phrase Encoder and become word candidates that make up phrases.
A video-grounded dialogue system referred to as the Structured Co-reference Graph Attention (SCGA) is presented for decoding the answer sequence to a question regarding a given video while keeping track of the dialogue context. Although recent efforts have made great strides in improving the quality of the response, performance is still far from satisfactory. The two main challenging issues are as follows: (1) how to deduce co-reference among multiple modalities and (2) how to reason on the rich underlying semantic structure of video with complex spatial and temporal dynamics. To this end, SCGA is based on (1) Structured Co-reference Resolver that performs dereferencing via building a structured graph over multiple modalities, (2) Spatio-temporal Video Reasoner that captures local-to-global dynamics of video via gradually neighboring graph attention. SCGA makes use of pointer network to dynamically replicate parts of the question for decoding the answer sequence. The validity of the proposed SCGA is demonstrated on AVSD@DSTC7 and AVSD@DSTC8 datasets, a challenging video-grounded dialogue benchmarks, and TVQA dataset, a large-scale videoQA benchmark. Our empirical results show that SCGA outperforms other state-of-the-art dialogue systems on both benchmarks, while extensive ablation study and qualitative analysis reveal performance gain and improved interpretability.
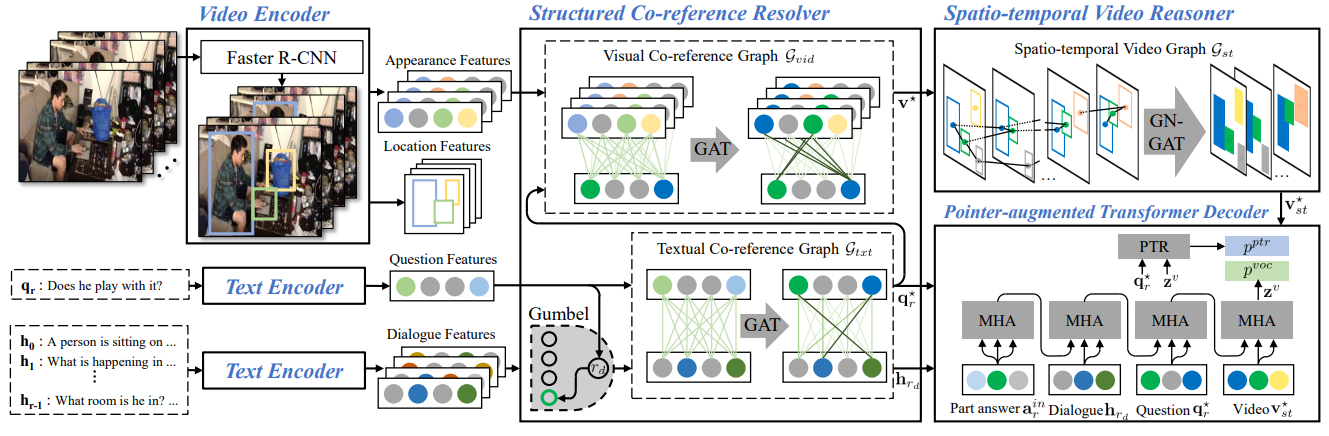
Figure 6. Illustration of Structured Co-reference Graph Attention (SCGA) which is composed of: (1) Input Encoder, (2) Structured Co-reference Resolver, (3) Spatio-temporal Video Reasoner, (4) Pointer-augmented Transformer Decoder.