KAIST EE Prof. Jang, Min Seok and his research team succeed in observing strongly confined mid-infrared light propagating on monocrystalline gold with a scattering-type scanning near-field optical microscope (s-SNOM).
It is highly applicable in next-generation optoelectronic devices development, with increasing the interaction between light and matter by confining the light in an atomically flat nanostructure. The study will be useful in advancing high-efficient nanophotonics and quantum computing.

[From left, Prof. Jang, Min Seok and Research Prof. Sergey Menabde]
KAIST announced on the July 18th that a joint research effort has succeeded in implementing a new platform for strongly confined light propagation in a low-dimensional material. This finding is expected to contribute to the development of next-generation optoelectronic devices development based on strong light-matter interactions.
Stacking atomically flat two-dimensional materials results in van der Waals crystals, which exhibit properties different from the original two-dimensional materials. Phonon-polaritons are the composite quasi-particles resulting from the polar dielectric ions’ oscillations coupling with electromagnetic waves. In particular, phonon-polaritons forming in van der Waals crystals placed on highly conductive metals have a high degree of compression. This is because the charges in the polariton crystals reflect in the underneath metal due to the image charge effect, and thus produce a new kind of polariton called image phonon-polaritons.
Light propagating as image phonon-polaritons may induce strong light-matter interactions, but their propagation is limited on rough metal surfaces and thus suffers low feasibility.
Prof. Jang said, “This work illustrates very well the advantages of image polaritons, especially image phonon-polaritons. Image phonon-polaritons exhibit low loss and strong light-matter interactions useful in the development of next-generation optoelectronic devices.” He then added that he hopes to advance the commercialization of high-efficiency nanophotonic devices, including in metasurfaces, optical switches, and optical sensors.
This work, first-authored by Research Prof. Sergey Menabde, was published in Science Advances on the July 13th. It has been supported by Samsung Science & Technology Foundation and the National Research Foundation of Korea, as well as Korea Institute of Science and Technology, Japanese Ministry of Education, Culture, Sports, Science and Technology, and the Villum Foundation of Denmark.
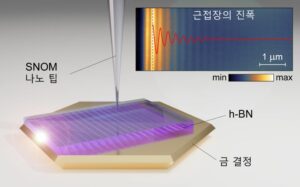
Fig. 1 Nanotip used in measuring the image phonon-polaritons traveling in h-BN in super-high resolution