to be the world’s
top IT powerhouse.We thrive to be the world’s top IT powerhouse.
Our mission is to lead innovations
in information technology, create lasting impact,
and educate next-generation leaders of the world.
- 1
- 6
to be the world’s
top IT powerhouse.We thrive to be the world’s top IT powerhouse.
Our mission is to lead innovations
in information technology, create lasting impact,
and educate next-generation leaders of the world.
- 2
- 6
to be the world’s
top IT powerhouse.We thrive to be the world’s top IT powerhouse.
Our mission is to lead innovations
in information technology, create lasting impact,
and educate next-generation leaders of the world.
- 3
- 6
to be the world’s
top IT powerhouse.We thrive to be the world’s top IT powerhouse.
Our mission is to lead innovations
in information technology, create lasting impact,
and educate next-generation leaders of the world.
- 4
- 6
to be the world’s
top IT powerhouse.We thrive to be the world’s top IT powerhouse.
Our mission is to lead innovations
in information technology, create lasting impact,
and educate next-generation leaders of the world.
- 5
- 6
are a key thrust
in EE researchAI and machine learning are a key thrust in EE research
AI/machine learning efforts are already a big part of ongoing
research in all 6 divisions - Computer, Communication, Signal,
Wave, Circuit and Device - of KAIST EE
- 6
- 6
develops a simulation
framework called vTrain
Achieves Human-Level Tactile Sensing with
Breakthrough Pressure Sensor
Seungwon Shin’s Team
Validates Cyber Risks
of LLMs
Wearable Carbon Dioxide Sensor
Joint Team Develops
Neuromorphic Semiconductor Chip
Develops High-Efficiency
Avalanche Quantum Dots
Develop AI That
Imagines and Understands
Microelectrodes Array
Hafnia-Based Ferroelectric Memory Technology
Highlights
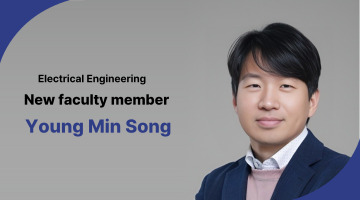
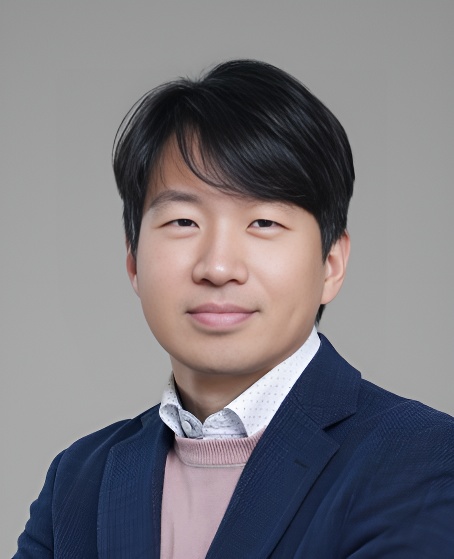
We are pleased to announce that Professor Young Min Song will be joining the KAIST School of Electrical Engineering as of July 1, 2025. Congratulations!
Professor Song’s temporary office is located in Room 1410, Saenel-dong (E3-4). His primary research areas include flexible optoelectronic devices and nanophotonics. He is actively working on biomimetic cameras for intelligent robotics, opto-neuromorphic devices and systems, nanophotonics-based reflective displays, and radiative cooling devices through infrared control. For more details on his research, please visit his homepage.
Homepage: https://www.ymsong.net
Click here to read Professor Song’s recent interview in Nature
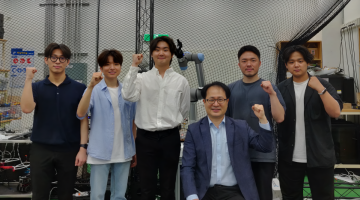
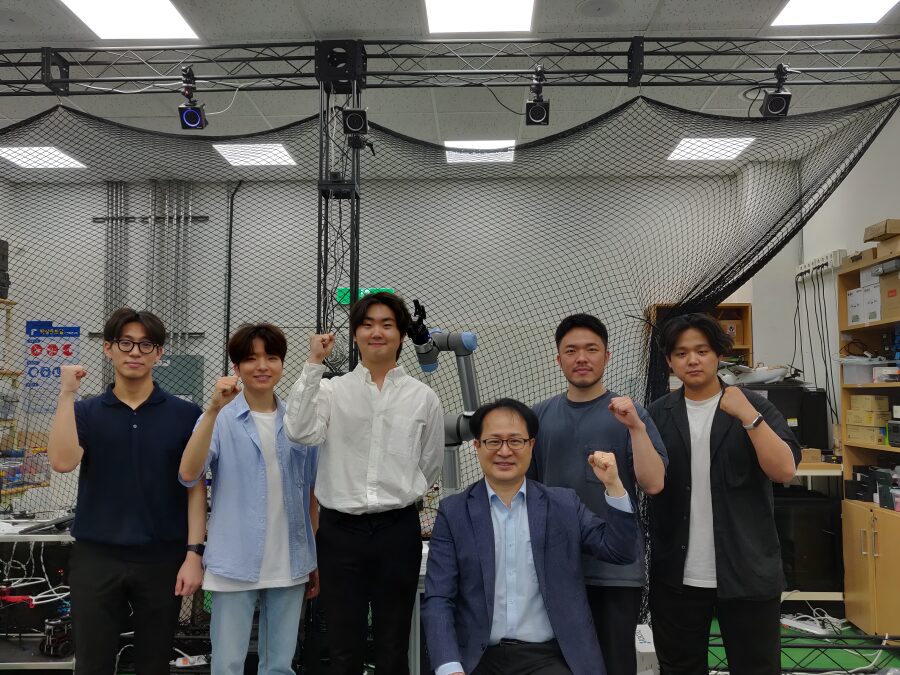
‘Team ACE’ from Professor Dong Eui Cang’s lab in our department achieved outstanding results by winning a Third Prize at the ‘Hugging Face LeRobot Worldwide Hackathon’, held over three days from June 14 to 16.
Composed of Seokjoon Kwon (Master’s Program, Team Leader), Hee-Deok Jang (Ph.D. Program), Hojun Kwon (Master’s Program), Guining Pertin (Master’s Program), and Kyeongdon Lee (Master’s Program) from Professor Dong Eui Chang’s lab, ‘Team ACE’ developed a VLA-based collaborative robot object transfer system and placed 20th out of more than 600 teams worldwide, earning a Third Prize (awarded to teams ranked 6th-24th). In addition, the team also received the KIRIA President’s Award (awarded by the Korea Institute for Robot Industry Advancement) from the local organizing committee in Daegu, South Korea.
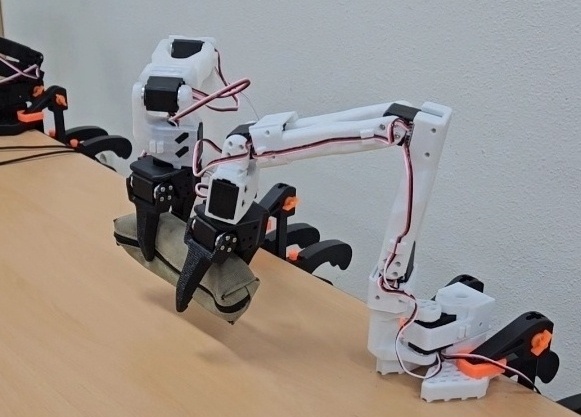
‘Hugging Face’ is a U.S.-based AI startup known as one of the world’s largest platforms for artificial intelligence, offering widely used machine learning libraries such as Transformers and Datasets. More recently, the company has also been actively providing AI resources for robotics applications.
Hugging Face regularly hosts global hackathons that bring together researchers and students from around the world to compete and collaborate on innovative AI-driven solutions.
This year’s ‘LeRobot Worldwide Hackathon’ gathered over 2,500 AI and robotics experts from 45 countries. Participants were challenged to freely propose and implement solutions to real-world problems in industry and everyday life by applying technologies such as VLA (Vision Language Action) models and reinforcement learning to robotic arms.
Through their achievement in the competition, ‘Team ACE’ was recognized for their technical excellence and creativity by both the global robotics community and experts in South Korea.
The team’s performance at the competition drew considerable attention from local media and was actively reported in regional news outlets.
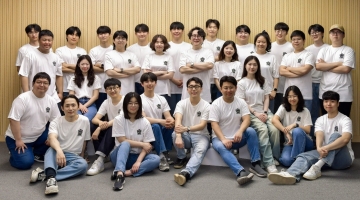
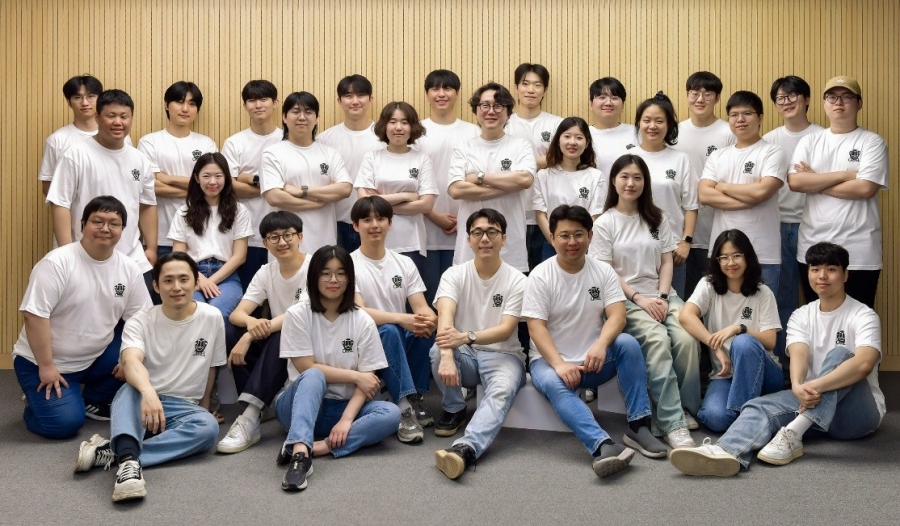
Professor Myoungsoo Jung’s research team is going to reveal their works on next-generation interconnect / semiconductor technologies in IEEE Micro, a leading journal in computer architecture.
IEEE Micro, established in 1981, is a bimonthly publication featuring recent advances in computer architecture and semiconductor technologies. Professor Jung’s team will present a total of five papers in the upcoming May-June issue on “Cache Coherent Interconnects and Resource Disaggregation Technology”.
Among them, three papers focus on applying Compute Express Link (CXL) to storage systems. Especially, the team introduces solutions to improve the performance of CXL-SSD, a concept for which Professor Jung suggested a practical implementation in early 2022. These technologies enable memory expansion through large-capacity SSDs while providing performance comparable to DRAM.
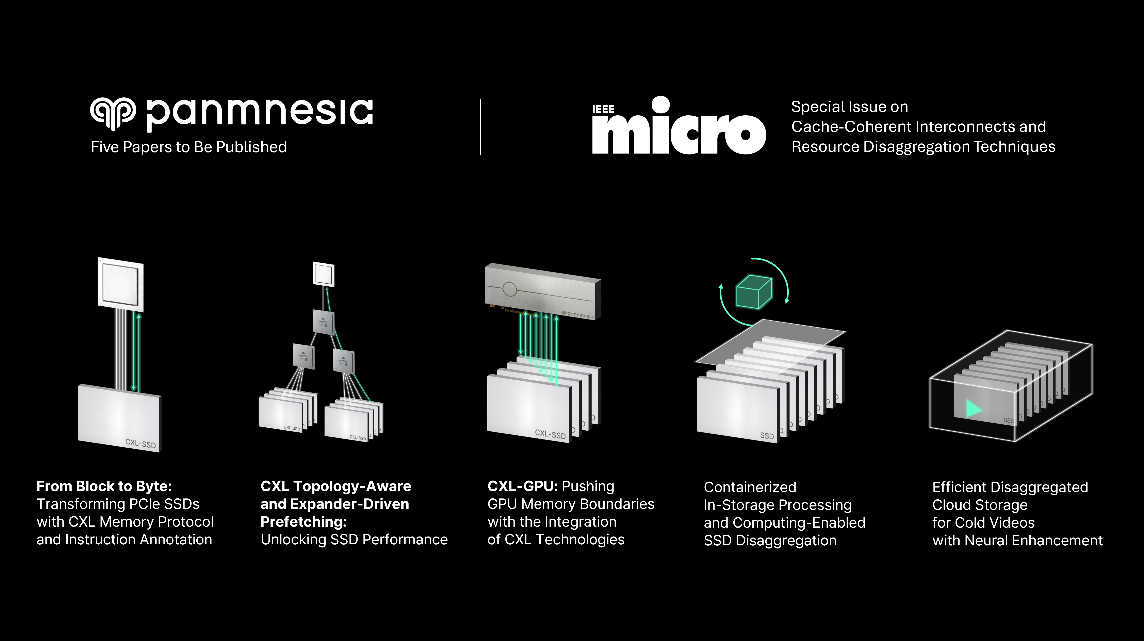
The team also explored storage architectures incorporating In-Storage Processing (ISP), which performs computation directly inside the storage pool. By processing data within storage, this approach reduces data movement and thereby improves efficiency in large-scale applications such as large language models (LLMs).
These papers, conducted in collaboration with the faculty-led startup Panmnesia, will be published through IEEE Micro’s official website and in its regular print issue.
- Early Access Link #1: From Blocks to Byte: Transforming PCIe SSDs with CXL Memory Protocol and Instruction Annotation
- Early Access Link #2: CXL Topology-Aware and Expander-Driven Prefetching: Unlocking SSD Performance
- Early Access Link #3: CXL-GPU: Pushing GPU Memory Boundaries with the Integration of CXL Technologies
- Early Access Link #4: Containerized In-Storage Processing and Computing-Enabled SSD Disaggregation
- Early Access Link #5: Efficient Disaggregated Cloud Storage for Cold Videos with Neural Enhancement
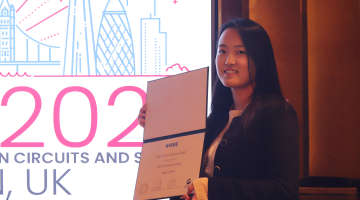
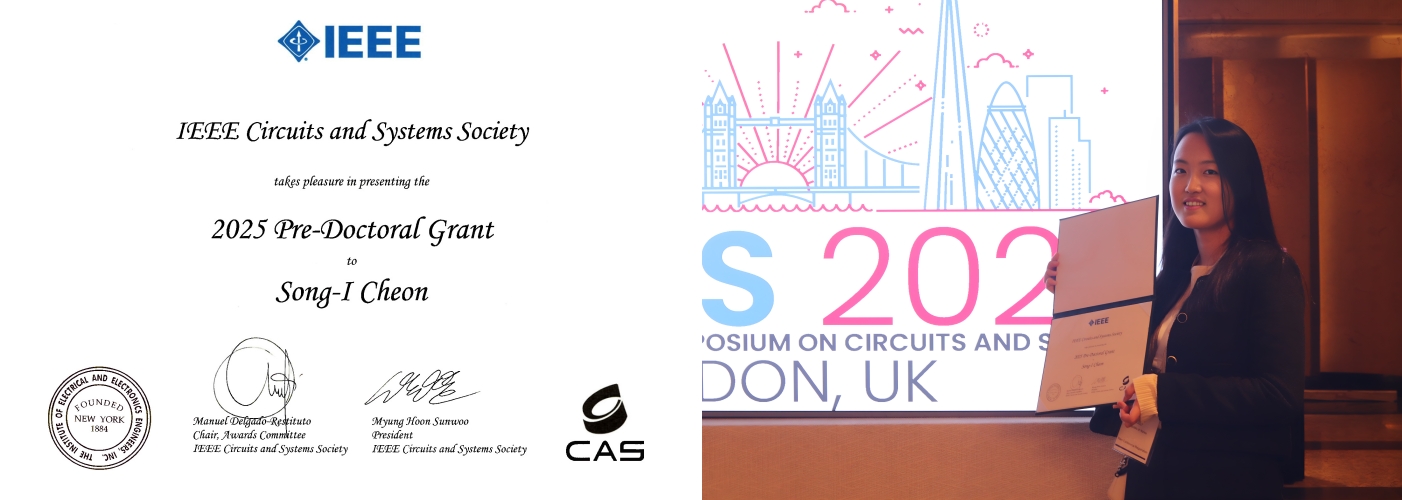
Song-I Cheon, a Ph.D. student in the Department of Electrical and Electronic Engineering under the supervision of Professor Minkyu Je, has been selected as a recipient of the 2025 IEEE Circuits and Systems Society (CASS) Pre-Doctoral Grant.
This prestigious grant is awarded annually to a select number of doctoral students worldwide who have demonstrated outstanding research potential in the field of system semiconductor circuit design. In 2025, only four students globally were selected, with Song-I being one of the recipients.
Song-I has published a total of 19 papers in international journals and conferences, including one paper at ISSCC (as co-first author) and four in IEEE journals (as first or co-first author). Notably, nine of these papers were presented at journals and conferences sponsored by IEEE CASS. Her research contributions in impedance measurement circuit design—particularly in the areas of optimization, high accuracy, and low power consumption—were highly recognized in the selection process.
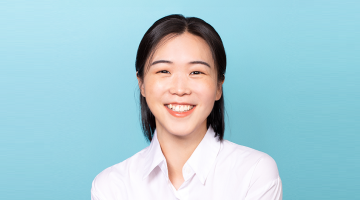
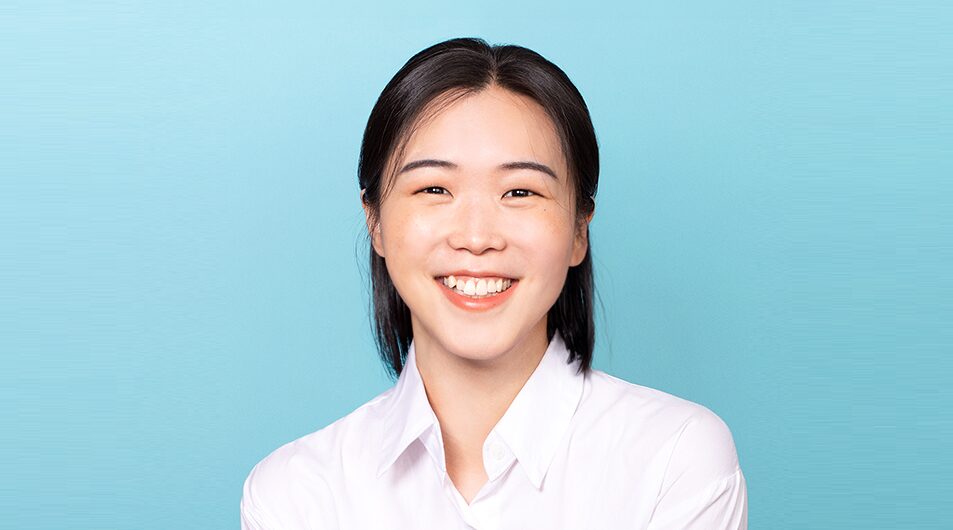
Alongside large language models, autonomous driving and humanoid robots, AI-driven optimization of industrial manufacturing has emerged as a major application of AI. In 2024, Kim Jeong-hye, a PhD student of Professor Youngchul Sung, interned on LG AI Research’s reinforcement-learning team, where she tackled a range of process-optimization challenges across LG Group’s production facilities.
That team applied optimization majorly based on reinforcement learning to LG Chem’s Daesan plant’s naphtha-cracking facility (NCC), improving production efficiency by 3%, far beyond of 0.1% of initial expectation, and yielding an extra KRW 10 billion in annual profit for that plant alone. Because training reinforcement learning agents via on-line interaction in a production environment is impractical, such optimization typically relies on offline reinforcement learning, which optimizes policies with pre-collected data.
Jeonghye contributed to the development of PARS, a novel offline reinforcement learning algorithm that significantly outperforms existing methods. By enhancing the neural network’s feature resolution with reward scaling with layer normalization, this new approach better differentiates between in-sample and out-of-distribution data, eliminating Q-value divergence, the core issue of off-line reinforcement learning. This advancement promises to accelerate future production-process optimizations as well as many RL applications with difficulty in on-line environment interaction.
This research result will be presented as a Spotlight paper at the International Conference on Machine Learning (ICML) 2025.
Related Yonhap News article: https://www.yna.co.kr/view/AKR20250613153400003
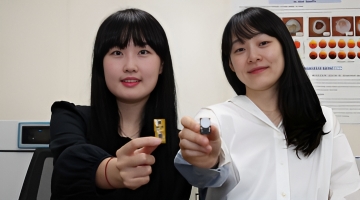
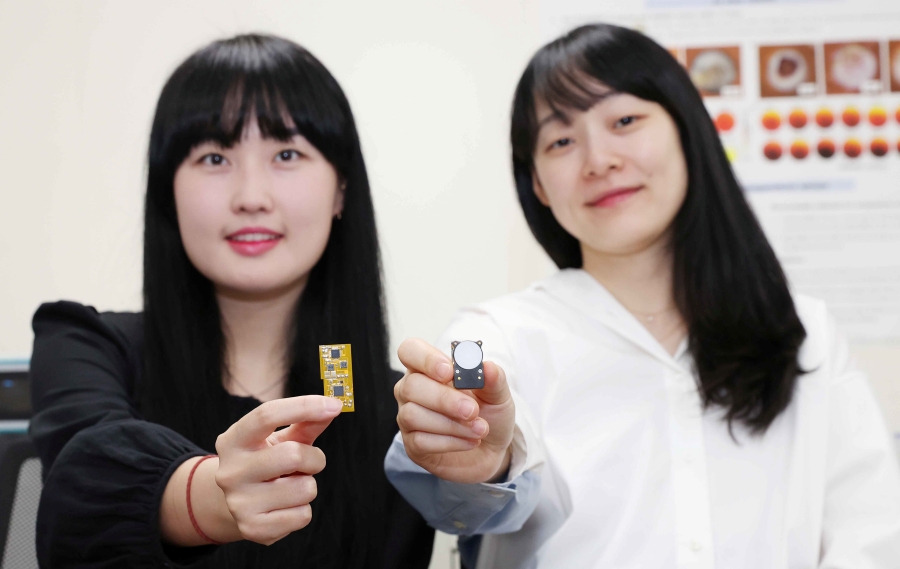
The core of this new system is an “Inertia-driven Triboelectric Nanogenerator (TENG)” that converts vibrations (with amplitudes ranging from 20-4000 ㎛ and frequencies from 0-300 Hz) generated by industrial equipment or pipelines into electricity. This enables periodic CO2 concentration measurements and wireless transmission without the need for batteries.
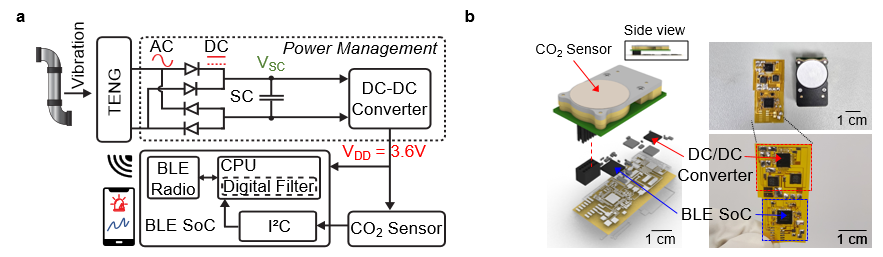
The research team successfully amplified fine vibrations and induced resonance by combining spring-attached 4-stack TENGs. They achieved stable power production of 0.5 mW under conditions of 13 Hz and 0.56 g acceleration. The generated power was then used to operate a CO2 sensor and a Bluetooth Low Energy (BLE) system-on-a-chip (SoC).
Professor Kyeongha Kwon emphasized, “For efficient environmental monitoring, a system that can operate continuously without power limitations is essential.” She explained, “In this research, we implemented a self-powered system that can periodically measure and wirelessly transmit CO2 concentrations based on the energy generated from an inertia-driven TENG.” She added, “This technology can serve as a foundational technology for future self-powered environmental monitoring platforms integrating various sensors.”
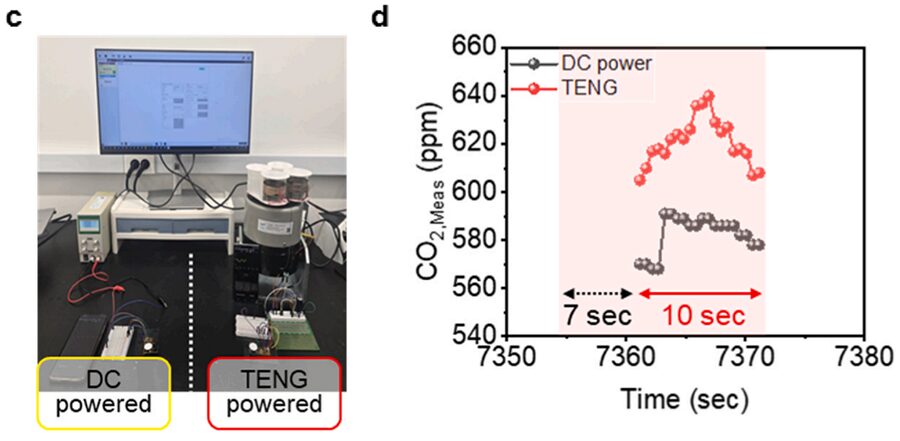
This research was published on June 1st in the internationally renowned academic journal `Nano Energy (IF 16.8)`. Gyurim Jang, a master’s student at KAIST, and Daniel Manaye Tiruneh, a master’s student at Chung-Ang University, are the co-first authors of the paper. *Paper Title: Highly compact inertia-driven triboelectric nanogenerator for self-powered wireless CO2 monitoring via fine-vibration harvesting *DOI: https://doi.org/10.1016/j.nanoen.2025.110872
This research was supported by the Saudi Aramco-KAIST CO2 Management Center.
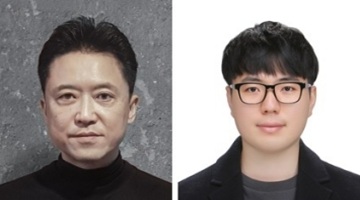
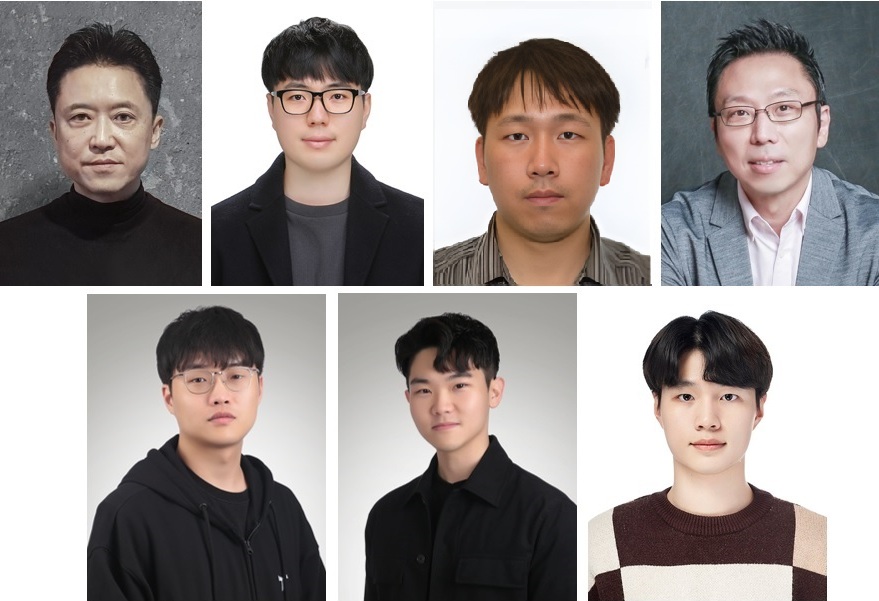
South Korea is the only country in the world that mandates the installation of government-approved security software—known as Korea Security Applications (KSAs)—for access to online financial and public services. But according to new research to be presented at USENIX Security 2025, this well-intentioned policy may be turning into a national cybersecurity liability.
A team of researchers from KAIST, Korea University, Sungkyunkwan University, and the cybersecurity firm Theori has uncovered systemic design flaws and critical implementation vulnerabilities in the very software meant to protect millions of South Koreans. In total, the team found 19 severe security issues across seven KSA tools, including keylogging, remote code execution, man-in-the-middle attacks, certificate exfiltration, and user tracking.
The research was motivated by real-world attacks: in several confirmed incidents, North Korean threat actors exploited vulnerabilities in these very security tools to compromise South Korean users. These events prompted the researchers to conduct a deeper investigation into the KSA ecosystem—and what they found was alarming.
“The fact that this software is mandated and installed across millions of endpoints makes it an especially attractive and efficient target,” said Professor Yongdae Kim of KAIST. “After seeing repeated evidence that attackers were exploiting these tools—not despite their security function, but because of it—we realized a systematic analysis was urgently needed.”
While some of the flaws discovered by the researchers have since been patched, many of the root causes remain unresolved. At issue is the architecture itself: rather than working with modern browser security models, KSA tools bypass them entirely. Designed to provide enhanced protections like encrypted keyboard input and certificate management, KSAs accomplish this by circumventing browser-level protections such as the Same-Origin Policy, sandboxing, and privilege separation—core tenets of modern web security.
Historically, this was achieved through now-defunct technologies like ActiveX. After ActiveX was phased out in 2015 due to widespread vulnerabilities, developers began distributing standalone executable files (.exe) that performed the same functions with many of the same risks—effectively reintroducing the problem in a different form.
In two proof-of-concept videos released by the research team, an attacker-controlled website is shown intercepting keystrokes—including passwords—and silently downloading malware by abusing KSA components. These behaviors would be blocked under standard browser security, but the KSAs, running with elevated privileges, make them possible.
A nationwide survey of 400 South Korean users found that 97.4% had installed KSA software, while nearly 60% said they didn’t understand what the programs did. Analysis of 48 real-world PCs revealed that users had an average of nine KSA programs installed, many of them outdated by several years.
“This isn’t just about bugs,” said Kim. “This is a philosophical misalignment between modern security standards and legacy design choices. When you hardcode mistrust of the web into your system architecture, you end up with software that behaves like spyware.”
The researchers argue that it’s time for South Korea to abandon its reliance on non-standard, government-mandated software and instead embrace web standards and modern browser-based security models. They warn that, if left unaddressed, the KSA ecosystem will continue to pose not only a risk to individual users but also a systemic threat to national cybersecurity.
The full paper, “Too Much of a Good Thing: (In-)Security of Mandatory Security Software for Financial Services in South Korea,” will be presented at the USENIX Security Symposium 2025, one of the premier venues for cybersecurity research. The project was supported by grants from the Institute of Information & Communications Technology Planning & Evaluation (IITP).
Paper: Too Much of a Good Thing (PDF)
Demo Video 1 (Keystroke Interception)
Demo Video 2 (Remote Code Execution):
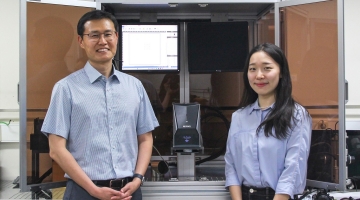
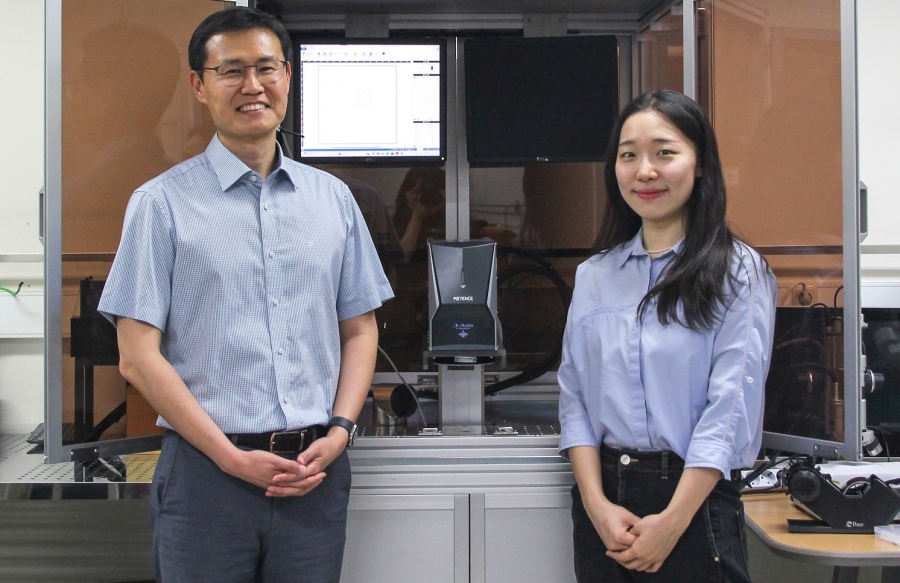
A team of researchers from KAIST and Seoul National University has developed a groundbreaking electronic ink that enables room-temperature printing of variable-stiffness circuits capable of switching between rigid and soft modes. This advancement marks a significant leap toward next-generation wearable, implantable, and robotic devices.
Variable-stiffness electronics are at the forefront of adaptive technology, offering the ability for a single device to transition between rigid and soft modes depending on its use case. Gallium, a metal known for its high rigidity contrast between solid and liquid states, is a promising candidate for such applications. However, its use has been hindered by challenges including high surface tension, low viscosity, and undesirable phase transitions during manufacturing.
On June 4th, a research team led by Professor Jae-Woong Jeong from the School of Electrical Engineering at KAIST, Professor Seongjun Park from the Digital Healthcare Major at Seoul National University, and Professor Steve Park from the Department of Materials Science and Engineering at KAIST introduced a novel liquid metal electronic ink. This ink allows for micro-scale circuit printing – thinner than a human hair – at room temperature, with the ability to reversibly switch between rigid and soft modes depending on temperature.
The new ink combines printable viscosity with excellent electrical conductivity, enabling the creation of complex, high-resolution multilayer circuits comparable to commercial printed circuit boards (PCBs). These circuits can dynamically change stiffness in response to temperature, presenting new opportunities for multifunctional electronics, medical technologies, and robotics.
Conventional electronics typically have fixed form factors – either rigid for durability or soft for wearability. Rigid devices like smartphones and laptops offer robust performance but are uncomfortable when worn, while soft electronics are more comfortable but lack precise handling. As demand grows for devices that can adapt their stiffness to context, variable-stiffness electronics are becoming increasingly important.

To address this challenge, the researchers focused on gallium, which melts just below body temperature. Solid gallium is quite stiff, while its liquid form is fluid and soft. Despite its potential, gallium’s use in electronic printing has been limited by its high surface tension and instability when melted.
To overcome these issues, the team developed a pH-controlled liquid metal ink printing process. By dispersing micro-sized gallium particles into a hydrophilic polyurethane matrix using a neutral solvent (dimethyl sulfoxide, or DMSO), they created a stable, high-viscosity ink suitable for precision printing. During post-print heating, the DMSO decomposes to form an acidic environment, which removes the oxide layer on the gallium particles. This triggers the particles to coalesce into electrically conductive networks with tunable mechanical properties.
The resulting printed circuits exhibit fine feature sizes (~50 μm), high conductivity (2.27 × 10⁶ S/m), and a stiffness modulation ratio of up to 1,465 – allowing the material to shift from plastic-like rigidity to rubber-like softness. Furthermore, the ink is compatible with conventional printing techniques such as screen printing and dip coating, supporting large-area and 3D device fabrication.
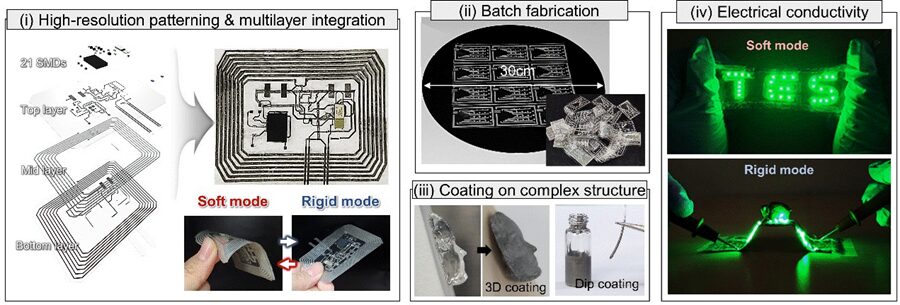
The team demonstrated this technology by developing a multi-functional device that operates as a rigid portable electronic under normal conditions but transforms into a soft wearable healthcare device when attached to the body. They also created a neural probe that remains stiff during surgical insertion for accurate positioning but softens once inside brain tissue to reduce inflammation – highlighting its potential for biomedical implants.

“The core achievement of this research lies in overcoming the longstanding challenges of liquid metal printing through our innovative technology,” said Professor Jeong. “By controlling the ink’s acidity, we were able to electrically and mechanically connect printed gallium particles, enabling the room-temperature fabrication of high-resolution, large-area circuits with tunable stiffness. This opens up new possibilities for future personal electronics, medical devices, and robotics.”
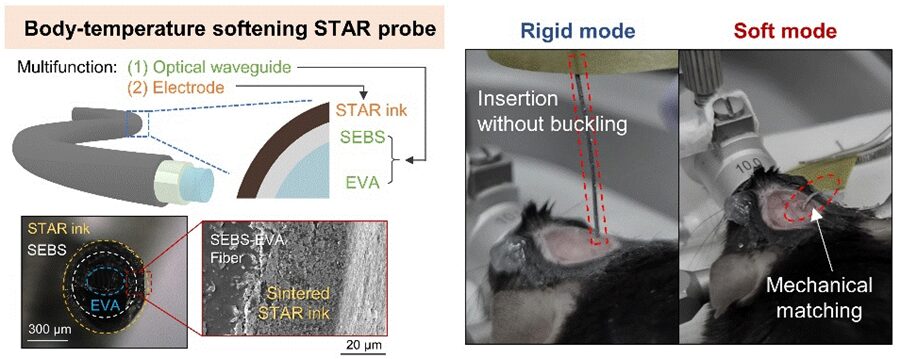
This research was published in Science Advances under the title, “Phase-Change Metal Ink with pH-Controlled Chemical Sintering for Versatile and Scalable Fabrication of Variable Stiffness Electronics.” The work was supported by the National Research Foundation of Korea, the Boston-Korea Project, and the BK21 FOUR Program.