Professor Shim Hyun-Chul(Principal Investigators), Professor Kim Min-Jun, and Collaborative Research Team Develop World’s First Humanoid Pilot, “PiBot.”
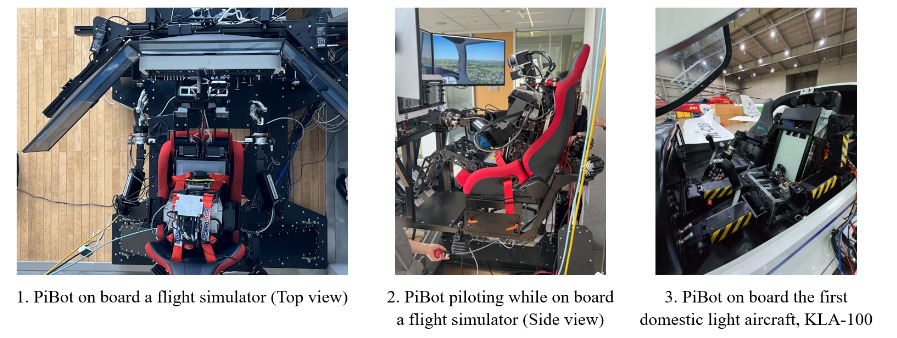
Recently, there has been a lot of attention on KAIST’s development of the humanoid pilot “PiBot” following the legendary pilot Maverick’s flights in the recent movie “Top Gun: Maverick.”
KAIST (President Lee Kwang-Hyung) announced on the 19th that they plan to develop a practical humanoid robot capable of directly piloting an aircraft based on understanding technical manuals written in natural language.
The collaborative research team, led by KAIST Professor Shim Hyun-Chul and including Professor Choo Jae-Gul, Professor Yoon Kuk-Jin, and Professor Kim Minjun, applied artificial intelligence and robotics technology to develop an “NLP-based humanoid pilot robot,” as part of the Future Challenge Project, which involves reading and comprehending pilot manuals written in general language.
The robot was able to sit in the cockpit of a conventional aircraft without any modification and directly operate various controls, showcasing a different approach from conventional aircraft’s autopilot systems or unmanned aerial vehicles that can only perform unmanned flights.
The pilot robot being developed by the research team can memorize and flawlessly operate the entire global Jeppesen Chart, which is impossible for human pilots.
It can also immediately respond by leveraging the recent breakthroughs in ChatGPT technology, recalling aircraft operation manuals and Quick Reference Handbook (QRH) procedures, and calculating real-time safe routes based on the aircraft’s flight status.
The robot can accurately perceive the internal and external conditions of the cockpit and the aircraft using the onboard cameras, precisely manipulate various switches, and control its robotic arms and hands with high precision even in highly vibrating aircraft environments.
Currently, the pilot robot is capable of performing all aircraft operations, including starting the engine, taxiing, takeoff, cruising, and landing, in a flight simulator. The research team plans to apply the pilot robot to an actual light aircraft for validation.
Professor Shim Hyun-Chul, the project leader, stated, “The humanoid pilot robot has high practicality and applicability, as it can immediately perform autonomous flights without any modification to existing aircraft. It can also operate a variety of devices, including aircraft, cars, and armored vehicles, making it highly useful in situations where there is a shortage of human resources.”
The ongoing project is supported by the Future Challenge Project from the Defense Science Institute (total funding of 5.7 billion KRW) and has been in development since 2022 through the collaboration of Professor Shim Hyun-Chul from the Department of Electrical and Electronic Engineering at KAIST (project leader), Professor Choo Jae-Gul from the AI Graduate School, Professor Yoon Kuk-Jin from the Department of Mechanical Engineering, and Professor Kim Min-Jun from the Department of Electrical and Electronic Engineering.
The project is expected to be completed in 2026 and explore commercialization plans for civilian and military applications.
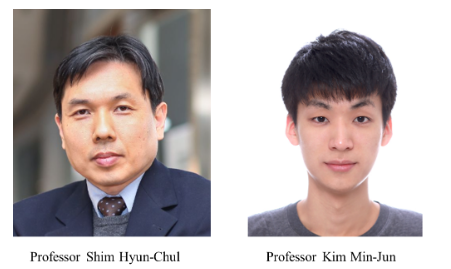
[Related News]