EE Professor Joung-Ho Kim Establishes NAVER-Intel-KAIST AI Joint Research Center(NIK AI Research Center) for the Development of Next-Generation AI Semiconductor Eco-System
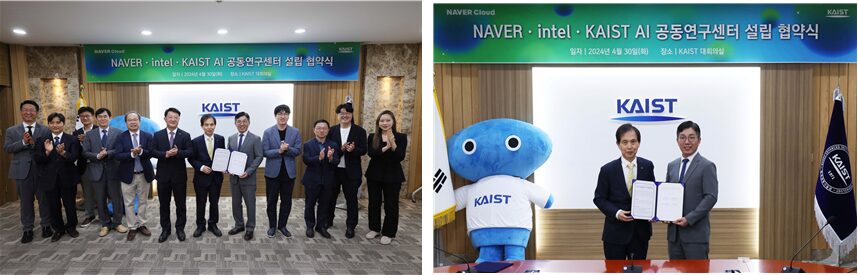
<MoU Singing Ceremony of Joint Research Center>
As generative AI, sparked by ChatGPT, sweeps the globe, Professor Joung-Ho Kim(KAIST), is joining forces with Naver and Intel to consolidate their capabilities and strengths in the new “NAVER·Intel·KAIST AI Joint Research Center (NIK AI Research Center)” to establish an ecosystem for new AI semiconductors.
Industry professionals view the strategic partnership between these three institutions as a proactive challenge to establish a new AI semiconductor ecosystem and secure market and technological leadership. They aim to integrate their individual hardware and software technologies and infrastructures in AI, including the development of open-source software necessary for the operation of AI semiconductors, AI servers, and data centers.
In particular, it is noteworthy that Intel, a global semiconductor company known for advanced CPU design and foundry capabilities, is establishing and supporting a joint research center at a domestic university—KAIST—for the first time. This initiative aims to develop open-source software and other necessary tools to optimally operate Intel’s AI semiconductor, “GAUDI”, marking a significant step beyond traditional central processing units (CPUs).
KAIST announced on the 30th that it has signed a Memorandum of Understanding (MOU) to establish and operate the “NAVER·Intel·KAIST AI Joint Research Center (NIK AI Research Center)” at its main campus in Daejeon. This collaboration with Naver Cloud, led by CEO Yu-won Kim, focuses on developing advanced open-source software aimed at enhancing the performance and optimizing the operation of AI semiconductors, AI servers, clouds, and data centers.
A KAIST representative emphasized the significance of Intel’s decision, stating, “It is of great strategic importance that Intel has chosen Naver and KAIST as partners for the development of open-source software in the fields of AI and semiconductors.“
The representative further detailed, “The combination of Naver Cloud’s excellence in computing, databases, and various AI services based on the NAVER Cloud Platform, Intel’s next-generation AI chip technology, and KAIST’s world-class expertise and software research capabilities, is expected to successfully create a distinctively creative and innovative ecosystem in the AI semiconductor sector.”
At the MOU signing ceremony, key KAIST officials including President Kwang-Hyung Lee, Provost and Executive Vice President Gyun-Min Lee, Senior Vice President for Research Sang-yup Lee, and Professor Joung-Ho Kim from the Department of Electrical Engineering were present. From Naver Cloud, key executives such as CEO Yu-won Kim, Head of AI Innovation Jung-Woo Ha, and Executive Officer Dong-soo Lee, responsible for Hyperscale AI, also attended.
Following the MOU signing, KAIST and Naver Cloud plan to establish the “NAVER·Intel·KAIST AI Joint Research Center (NIK AI Research Center)” at KAIST within the first half of the year. They are scheduled to commence full-scale research activities starting in July.
At KAIST, Professor Joung-Ho Kim of the Department of Electrical Engineering, recognized globally as a leading scholar in AI semiconductor design and AI application design (AI-X), will co-lead the NIK AI Research Center. From Naver Cloud, Executive Officer Dong-soo Lee, an expert in AI semiconductor design and AI software, will serve as the other co-director of the center. Additionally, Professor Min-hyuk Sung from the KAIST Department of Computer Science and Naver Cloud’s Leader Se-jung Kwon will each serve as deputy directors, collaboratively steering the center’s research initiatives.
The operation period of the joint research center is initially set for three years, with the possibility of extension based on research outcomes and the needs of the participating institutions. As a key research center, about 20 faculty members specializing in artificial intelligence and software from KAIST, along with approximately 100 master’s and doctoral students, will participate as researchers, ensuring the center is equipped with substantial expertise and innovation capacity.
During the initial two years, the joint research center will focus on establishing a platform ecosystem specifically for the AI training and inference chip, “GAUDI”, developed by Intel’s Habana Labs. To achieve this, approximately 20 to 30 collaborative industry-academic research projects will be conducted.
Research at the joint research center primarily focuses on the development of open-source software in fields such as natural language processing, computer vision, and machine learning. Of the center’s research efforts, 50% is devoted to autonomous subject research, while 30% and 20% of the efforts are allocated to studies on the miniaturization and optimization of AI semiconductors, respectively.
To facilitate this research, Naver and Intel will provide the “GAUDI 2”—based on the Naver Cloud Platform—to the KAIST Joint Research Center. In turn, the KAIST research team will utilize “GAUDI 2” for their studies and annually publish their findings and papers related to this work.
Additionally, beyond their existing capabilities in artificial intelligence and cloud technologies, Naver and Intel will share various infrastructure facilities and equipment necessary for joint research. They also plan to engage in numerous collaborative activities, including supporting the joint research center with the necessary space and administrative staff and facilitating the exchange of research personnel between the institutions. This comprehensive support is designed to enhance the effectiveness and impact of their cooperative efforts.
Professor Joung-Ho Kim of highlighted the significant benefits of the joint research center, stating, “KAIST can acquire technical know-how in AI development, semiconductor design, and operational software development through the use of the GAUDI series. Particularly, the establishment of this joint research center is highly meaningful as it allows us to gain experience in operating large-scale AI data centers and to secure the AI computing infrastructure needed for future research and development.”
Director Dong-soo Lee from Naver Cloud expressed his aspirations for the collaboration: “Naver Cloud looks forward to leading various research initiatives with KAIST and expanding the AI ecosystem centered around HyperCLOVA X. Through the joint research center, we hope to invigorate AI research in the country and enhance the diversity of the AI chip ecosystem.”
[ Terminologies ]
* Generative AI
: Artificial intelligence technology that uses deep learning models to learn from large datasets. It can actively generate outputs such as text, images, and videos based on user requests.
** GAUDI
: A general-purpose AI accelerator for data centers, developed by Habana Labs, an Israeli AI chip company acquired by Intel in 2019.
*** High Bandwidth Memory (HBM)
: A high-performance DRAM technology where multiple DRAM chips are interconnected using Through Silicon Vias (TSVs) to significantly enhance data processing speeds. It is primarily used in conjunction with GPUs to accelerate AI training and generation speeds. Characteristically, HBM is designed to maximize memory bandwidth, making it especially suitable for high-speed parallel processing. It is a critical semiconductor in AI computers installed in mega-scale generative AI data centers. The technology has evolved through several generations: HBM, HBM2, HBM2E, HBM3, and the current HBM3E. Companies like Samsung Electronics and SK Hynix are currently developing HBM4, which is used in GPU modules by NVIDIA, Intel, and AMD.