Imitation Learning with Bayesian Exploration (IL-BE) for Signal Integrity (SI) of PAM-4 based High-speed Serial Link: PCIe 6.0 (DesignCon 2022)
Authors: Jihun Kim, Minsu Kim, Hyunwook Park, Jiwon Yoon, Seonguk Choi, Joonsang Park, Haeyeon Kim, Keeyoung Son, Seongguk Kim, Daehwan Lho, Keunwoo Kim, Jinwook Song, Kyungsuk Kim, Jongkyu Park and Joungho Kim
Abstract: This paper proposes a novel imitation learning with Bayesian exploration (IL-BE) method to optimize via parameters of any given channel parameters for signal integrity (SI) of PAM-4 based high-speed serial link on PCIe 6.0. PCIe 6.0. is a crucial interconnect link for highspeed communication of processors, and PAM-4 signaling is a major component of PCIe 6.0 that can double bandwidth. However, the design space of PAM-4 based PCIe 6.0 is extremely complex. Moreover, because PAM-4 signaling reduces eye-margin 1/3 compared with NRZ signaling, it is more sensitive to optimize. Bayesian optimization (BO) is a candidate method because it shows powerful searching abilities on black-box continuous optimization space. However, BO has a significant limitation to apply via optimization for any given channel parameters because BO needs massive iterations to solve each problem (i.e., no adaptation to new tasks). Deep reinforcement learning is a promising method that deep neural network (DNN) agents learn to capture meta-features among problems interacting with the real-world environment. Therefore, learned DNN agents can adapt to a new problem by optimization with small iterations. However, DNN agents must learn through massive iterative trial and error; it is extremely complex to train DNN. We blend the benefit of the BO and DRL method. Firstly, we collect high-quality expert data using BO rather than relying on poor exploration of the initial DNN agent. Then we use the collected high-quality data to train DNN agents by using an imitation learning scheme. For verification, we target one pair differential PCIe 6.0 (64Gbps) interconnection of SSD board and three-layer transition; a task is formulated to optimize via given channel parameters. The statistical simulation method is used to evaluate SI performances, including PAM-4 eye-diagram. Proposed IL-BE shows 100× faster training speed than conventional DRL method, 16× reduced iterations for the via parameter optimization than BO having state-of-the-art SI performances.
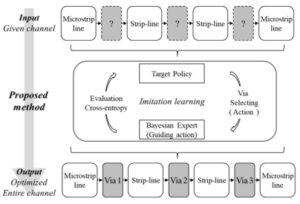
Title: PAM-4 based PCIe 6.0 Channel Design Optimization Method using Bayesian Optimization
(EPEPS 2021)
Authors: JihunKim, Hyunwook Park, Minsu Kim, Seonguk Choi, Keeyoung Son, Joonsang Park, Haeyeon Kim, Jinwook Song, Youngmin Ku, Jonggyu Park and Joungho Kim.
Abstract: This paper, for the first time, proposes a pulse amplitude modulation-4 (PAM-4) based peripheral component interconnect express (PCIe) 6.0 channel design optimization method using Bayesian Optimization (BO). The proposed method provides a sub-optimal channel design with PAM-4 signaling that maximizes target function considering signal integrity (SI). We formulate the target function of BO as a linear combination of the channel insertion loss (IL) and crosstalk (FEXT, NEXT) considering characteristics of PAM-4 signaling. To consider the trade-off between insertion loss and crosstalk in PAM-4 signaling, we obtain reasonable coefficients for formulating target function via ablation study. For verification, an eye diagram simulation with PAM-4 signaling is conducted. We compare the channel performance of the proposed method and random search method (RS). Also, the proposed method is compared with the only IL- considered BO method to verify the impact of crosstalk in PAM-4 signaling. As a result, the channel optimized by the proposed method only obtains eye height and eye width of PAM-4 eye diagram, and the PAM-4 eyes of other comparison methods are closed.
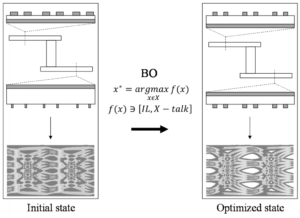
Title:
Deep Reinforcement Learning-based Channel-flexible Equalization Scheme: An Application to High Bandwidth Memory (DesignCon2022)
Authors:
Seonguk Choi, Minsu Kim, Hyunwook Park, Haeyeon Rachel Kim, Joonsang Park, Jihun Kim, Keeyoung Son, Seongguk Kim, Keunwoo Kim, Daehwan Lho, Jiwon Yoon, Jinwook Song, Kyungsuk Kim, Jonggyu Park and Joungho Kim.
Abstract:
In this paper, we propose a channel-flexible hybrid equalizer (HYEQ) design methodology with re-usability based on deep reinforcement learning (DRL). Proposed method suggests the optimized HYEQ design for arbitrary channel dimension. HYEQ is comprised of a continuous time linear equalizer (CTLE) for high-frequency boosting and passive equalizer (PEQ) for low frequency attenuation, and our task is to co-optimize both of them. Our model plays a role as a solver to optimize the design of equalizers, while considering all signal integrity issues such as high frequency attenuation, crosstalk and so on.
Our method utilizes recursive neural network commonly employed in natural language processing (NLP), in order to design HYEQ based on constructive DRL. Thus, each parameter of the equalizer is designed sequentially, reflecting other parameters. In this process, the design space of machine learning (ML) is determined by applying domain knowledge of equalizer, and thus even precise optimization is conducted. Furthermore, fast inference is conducted by trained neural network for any channel dimension. We validate that the proposed method outperforms conventional optimization algorithms such as random search (RS) and genetic algorithm (GA) in 3-coupled channel system of next generation high-bandwidth memory (HBM).
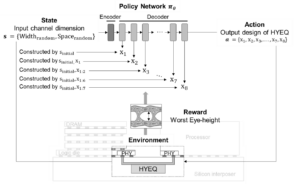
Title:
Sequential Policy Network-based Optimal Passive Equalizer Design for an Arbitrary Channel of High Bandwidth Memory using Advantage Actor Critic (EPEPS 2021)
Authors:
Seonguk Choi, Minsu Kim, Hyunwook Park, Keeyoung Son, Seongguk Kim, Jihun Kim, Joonsang Park, Haeyeon Kim, Taein Shin, Keunwoo Kim and Joungho Kim.
Abstract:
In this paper, we proposed a sequential policy network-based passive equalizer (PEQ) design method for an arbitrary channel of high bandwidth memory (HBM) using advantage actor critic (A2C) algorithm, considering signal integrity (SI) for the first time. PEQ design must consider the circuit parameters and placement for improving the performance. However, optimizing PEQ is complicated because various design parameters are coupled. Conventional optimization methods such as genetic algorithm (GA) repeat the optimization process for the changed conditions. In contrast, the proposed method suggests the improved solution based on the trained sequential policy network with flexibility for unseen conditions. For verification, we conducted electromagnetic (EM) simulation with optimized PEQs by GA, random search (RS) and the proposed method. Experimental results demonstrate that the proposed method outperformed the GA and RS by 4.4 \% and 6.4 \% respectively in terms of the eye-height.
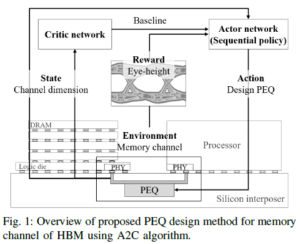
Title:
Deep Reinforcement Learning-based Channel-flexible Equalization Scheme: An Application to High Bandwidth Memory (DesignCon2022)
Authors:
Seonguk Choi, Minsu Kim, Hyunwook Park, Haeyeon Rachel Kim, Joonsang Park, Jihun Kim, Keeyoung Son, Seongguk Kim, Keunwoo Kim, Daehwan Lho, Jiwon Yoon, Jinwook Song, Kyungsuk Kim, Jonggyu Park and Joungho Kim.
Abstract:
In this paper, we propose a channel-flexible hybrid equalizer (HYEQ) design methodology with re-usability based on deep reinforcement learning (DRL). Proposed method suggests the optimized HYEQ design for arbitrary channel dimension. HYEQ is comprised of a continuous time linear equalizer (CTLE) for high-frequency boosting and passive equalizer (PEQ) for low frequency attenuation, and our task is to co-optimize both of them. Our model plays a role as a solver to optimize the design of equalizers, while considering all signal integrity issues such as high frequency attenuation, crosstalk and so on.
Our method utilizes recursive neural network commonly employed in natural language processing (NLP), in order to design HYEQ based on constructive DRL. Thus, each parameter of the equalizer is designed sequentially, reflecting other parameters. In this process, the design space of machine learning (ML) is determined by applying domain knowledge of equalizer, and thus even precise optimization is conducted. Furthermore, fast inference is conducted by trained neural network for any channel dimension. We validate that the proposed method outperforms conventional optimization algorithms such as random search (RS) and genetic algorithm (GA) in 3-coupled channel system of next generation high-bandwidth memory (HBM).
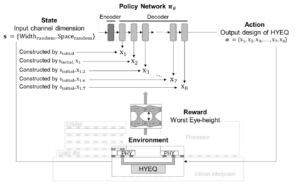
Title:
Sequential Policy Network-based Optimal Passive Equalizer Design for an Arbitrary Channel of High Bandwidth Memory using Advantage Actor Critic (EPEPS 2021)
Authors:
Seonguk Choi, Minsu Kim, Hyunwook Park, Keeyoung Son, Seongguk Kim, Jihun Kim, Joonsang Park, Haeyeon Kim, Taein Shin, Keunwoo Kim and Joungho Kim.
Abstract:
In this paper, we proposed a sequential policy network-based passive equalizer (PEQ) design method for an arbitrary channel of high bandwidth memory (HBM) using advantage actor critic (A2C) algorithm, considering signal integrity (SI) for the first time. PEQ design must consider the circuit parameters and placement for improving the performance. However, optimizing PEQ is complicated because various design parameters are coupled. Conventional optimization methods such as genetic algorithm (GA) repeat the optimization process for the changed conditions. In contrast, the proposed method suggests the improved solution based on the trained sequential policy network with flexibility for unseen conditions. For verification, we conducted electromagnetic (EM) simulation with optimized PEQs by GA, random search (RS) and the proposed method. Experimental results demonstrate that the proposed method outperformed the GA and RS by 4.4 \% and 6.4 \% respectively in terms of the eye-height.

Imitate Expert Policy and Learn Beyond: A Practical PDN Optimizer by Imitation Learning (DesignCon 2022, nominated for best paper award & Early career best paper award finalist)
Authors: Haeyeon Kim, Minsu Kim, Seonguk Choi, Jihun Kim, Joonsang Park, Keeyoung Son, Hyunwook Park, Subin Kim and Joungho Kim
Abstract: This paper proposes a practical and reusable decoupling capacitor (decap) placement solver using the attention model-based imitation learning (AM-IL). The proposed AM-IL framework imitates an expert policy by using pre-collected guiding datasets and trains a policy that outperforms the performance beyond the existing machine learning methods. The trained policy has reusability in terms of PDN with different probing port and keep-out regions; the constructed policy itself becomes the decap placement solver. In this paper, genetic algorithm is taken as an expert policy to verify how the proposed method generates a solver that learns beyond the level of the expert policy. The expert policy for imitation learning can be substituted by any algorithm or conventional tool, which means this is a fast and effective approach to improve existing methods. Moreover, by taking the existing data from the industry as guiding data or human experts as an expert policy, the proposed method can construct a reusable decap placement solver that is data-efficient, practical and guarantees a promising performance. This paper presents verification of AM-IL in comparison to two neural combinatorial optimization networks-based deep reinforcement learning methods, AM-RL and Ptr-RL. As a result, AM-IL achieved a performance score of 11.72, while AM-RL achieved 10.74 and Ptr-RL achieved 9.76. Unlike meta-heuristic methods such as genetic algorithm that require numerous iterations to find a near-optimal solution, the proposed AM-IL generates a near-optimal solution to any given problem by a single trial.
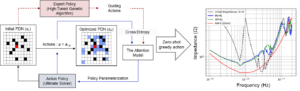
Title: Deep Reinforcement Learning Framework for Optimal Decoupling Capacitor Placement on General PDN with an Arbitrary Probing Port (EPEPS 2021)
Authors: Haeyeon Kim, Hyunwook Park, Minsu Kim, Seonguk Choi, Jihun Kim, Joonsang Park, Seongguk Kim, Subin Kim and Joungho Kim.
Abstract: This paper proposes a deep reinforcement learning (DRL) framework that learns a reusable policy to find the optimal placement of decoupling capacitors (decaps) on power distribution network (PDN) with an arbitrary probing port. The proposed DRL framework trains a policy parameterized by pointer network, which is a sequence-to-sequence neural network, based on REINFORCE algorithm. The policy finds the positional combination of a pre-defined number of decaps that best suppresses self-impedance of a given probing port on PDN with randomly assigned keep-out regions. Verification was done by allocating 20 decaps on ten randomly generated test sets with an arbitrary probing port and randomly selected keep-out regions. Performance of the policy generated by the proposed DRL framework was evaluated based on the magnitude of probing port self-impedance suppression followed by decap placement over 434 frequencies between 100MHz and 20GHz. The policy generated by the proposed framework achieves greater impedance suppression with fewer samples in comparison to random search heuristic method.
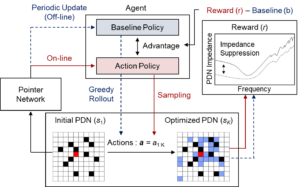
Conference: NeurIPS 2021
Title:
Learning Collaborative Policies to Solve NP-hard Routing Problems
Authors:
Minsu Kim, Jinkyoo Park and Joungho Kim.
Abstract:
Recently, deep reinforcement learning (DRL) frameworks have shown potential for solving NP-hard routing problems such as the traveling salesman problem (TSP) without problem-specific expert knowledge. Although DRL can be used to solve complex problems, DRL frameworks still struggle to compete with state-of-the-art heuristics showing a substantial performance gap. This paper proposes a novel hierarchical problem-solving strategy, termed learning collaborative policies (LCP), which can effectively find the near-optimum solution using two iterative DRL policies: the seeder and reviser. The seeder generates as diversified candidate solutions as possible (seeds) while being dedicated to exploring over the full combinatorial action space (i.e., sequence of assignment action). To this end, we train the seeder’s policy using a simple yet effective entropy regularization reward to encourage the seeder to find diverse solutions. On the other hand, the reviser modifies each candidate solution generated by the seeder; it partitions the full trajectory into sub-tours and simultaneously revises each sub-tour to minimize its traveling distance. Thus, the reviser is trained to improve the candidate solution’s quality, focusing on the reduced solution space (which is beneficial for exploitation). Extensive experiments demonstrate that the proposed two-policies collaboration scheme improves over single-policy DRL framework on various NP-hard routing problems, including TSP, prize collecting TSP (PCTSP), and capacitated vehicle routing problem (CVRP).
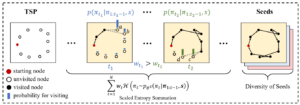
우리 학부 이준구 교수님 연구팀이 독일 및 남아공 연구팀과의 협력 연구를 통해 비선 형 양자 기계학습 인공지능 알고리즘을 개발하였습니다.
이번 연구를 통해 비선형 커널이 고안되어 복잡한 데이터에 대한 양자 기계학습이 가능하게 되었습니다. 특히 이준구 교수님 연구팀이 개발한 양자 지도학습 알고리즘은 학습에 있어 매우 적은 계산량으로 연산이 가능하여, 대규모 계산량이 필요한 현재의 인공지능 기술을 추월할 가능성을 제시한 것으로 평가를 받고 있습니다.
이준구 교수님 연구팀은 학습데이터와 테스트데이터를 양자 정보로 생성한 후 양자 정보의 병렬연산을 가능하게 하는 양자포킹 기술과 간단한 양자 측정기술을 조합해 양자 데이터 간의 유사성을 효율적으로 계산하는 비선형 커널 기반의 지도학습을 구현하는 양자 알고리즘 체계를 만들었습니다. 이후 IBM 클라우드 서비스를 통해 실제 양자컴퓨터에서 양자 지도학습을 실제 시연하는 데 성공했습니다. KAIST 박경덕 연구교수가 공동 제1 저자로 참여한 이번 연구결과는 국제 학술지 네이처 자매지인 `npj Quantum Information’ 誌 2020년 5월 6권에 게재되었습니다. (논문명: Quantum classifier with tailored quantum kernel).
연구팀은 이와 함께 양자 회로의 체계적 설계를 통해 다양한 양자 커널 구현이 가능함을 이론적으로 증명했습니다. 커널 기반 기계학습에서는 주어진 입력 데이터에 따라 최적 커널이 달라질 수 있으므로, 다양한 양자 커널을 효율적으로 구현할 수 있게 된 점은 양자 커널 기반 기계학습의 실제 응용에 있어 매우 중요한 성과입니다.
이 연구에 참여한 박경덕 연구교수님은 “연구팀이 개발한 커널 기반 양자 기계학습 알고리즘은 수년 안에 상용화될 것으로 예측되는 수백 큐비트의 NISQ(Noisy Intermediate-Scale Quantum) 컴퓨팅의 시대가 되면 기존의 고전 커널 기반 지도학습을 뛰어넘을 것ˮ이라면서 “복잡한 비선형 데이터의 패턴 인식 등을 위한 양자 기계학습 알고리즘으로 활발히 사용될 것ˮ이라고 말했습니다.
한편 이번 연구는 각각 한국연구재단의 창의 도전 연구기반 지원 사업과 한국연구재단의 한-아프리카 협력기반 조성 사업, 정보통신기획평가원의 정보통신기술인력 양성사업(ITRC)의 지원을 받아 수행되었습니다.
아래의 링크에서 관련 논문에 대한 정보를 확인하실 수 있습니다.
다시 한 번, 양자 분야에서 뛰어난 행보를 보이시는 이준구 교수님 연구팀 성과에 박수를 보냅니다.
[Link]
https://www.nature.com/articles/s41534-020-0272-6